Multitask Deep Learning Methods for Improving Human Context Recognition From Low Sampling Rate Sensor Data
IEEE Sensors Journal(2023)
摘要
High-fidelity sensor data gathered at high sampling rates is required by human context recognition (HCR) to accurately predict the users' current context. This approach consumes a significant amount of energy and increases the likelihood of uninstallation. Prior work addressed energy scarcity by reducing smartphone sensor sampling rates, which in turn reduces HCR accuracy. Up-sampling or domain adaptation (DA) techniques were then introduced to maximize HCR accuracy from sensor data with low-sampling rates. These solutions, however, are only applicable if high sampling rates of data from all context classes can be gathered from the target population, which is not realistic in a real-world application. Alternatively, the learning of HCR on multiple datasets with different sampling rates and labels is proposed in this study. We address the problem as a dataset shift problem to improve the learning of the low-sampling rate HCR dataset using information augmented from a high-sampling dataset, collected under various setups (labels, mobile devices, and population). To reduce the discrepancy of feature distribution between sampling rates and dataset domains, we propose maximum mean discrepancy-based multitask learning (MMD-MTL), which innovatively introduces MMD into MTL to address the covariance shift between sampling rates within the same dataset and the dataset shift between multiple HCR datasets. In rigorous evaluation, using an external HCR dataset that gathered sensor data at 50 Hz from various sets of populations, devices, and HCR labels, MMD-MTL was found to significantly increase the HCR accuracy of a crowdsourced dataset that was sampled at a rate of 5 Hz up to 74%.
更多查看译文
关键词
Deep learning,human context recognition (HCR),low sampling
AI 理解论文
溯源树
样例
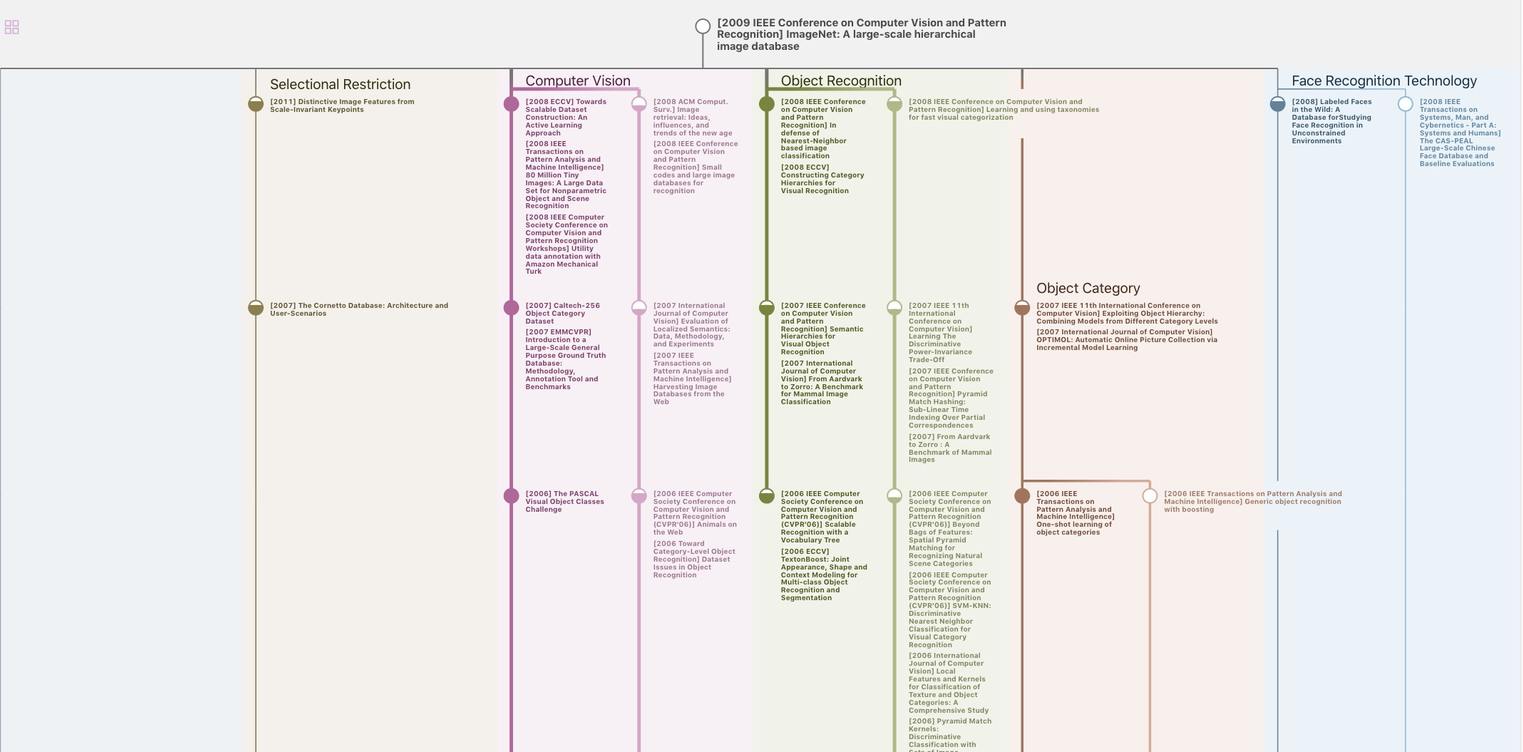
生成溯源树,研究论文发展脉络
Chat Paper
正在生成论文摘要