A systematic comparison of computational methods for expression forecasting.
bioRxiv : the preprint server for biology(2023)
摘要
Due to the abundance of single cell RNA-seq data, a number of methods for predicting expression after perturbation have recently been published. Expression prediction methods are enticing because they promise to answer pressing questions in fields ranging from developmental genetics to cell fate engineering and because they are faster, cheaper, and higher-throughput than their experimental counterparts. However, the absolute and relative accuracy of these methods is poorly characterized, limiting their informed use, their improvement, and the interpretation of their predictions. To address these issues, we created a benchmarking platform that combines a panel of large-scale perturbation datasets with an expression forecasting software engine that encompasses or interfaces to current methods. We used our platform to systematically assess methods, parameters, and sources of auxiliary data. We found that uninformed baseline predictions, which were not always included in prior evaluations, yielded the same or better mean absolute error than benchmarked methods in all test cases. These results cast doubt on the ability of current expression forecasting methods to provide mechanistic insights or to rank hypotheses for experimental follow-up. However, given the rapid pace of innovation in the field, new approaches may yield more accurate expression predictions. Our platform will serve as a neutral benchmark to improve methods and to identify contexts in which expression prediction can succeed.
更多查看译文
关键词
expression,computational methods,systematic comparison
AI 理解论文
溯源树
样例
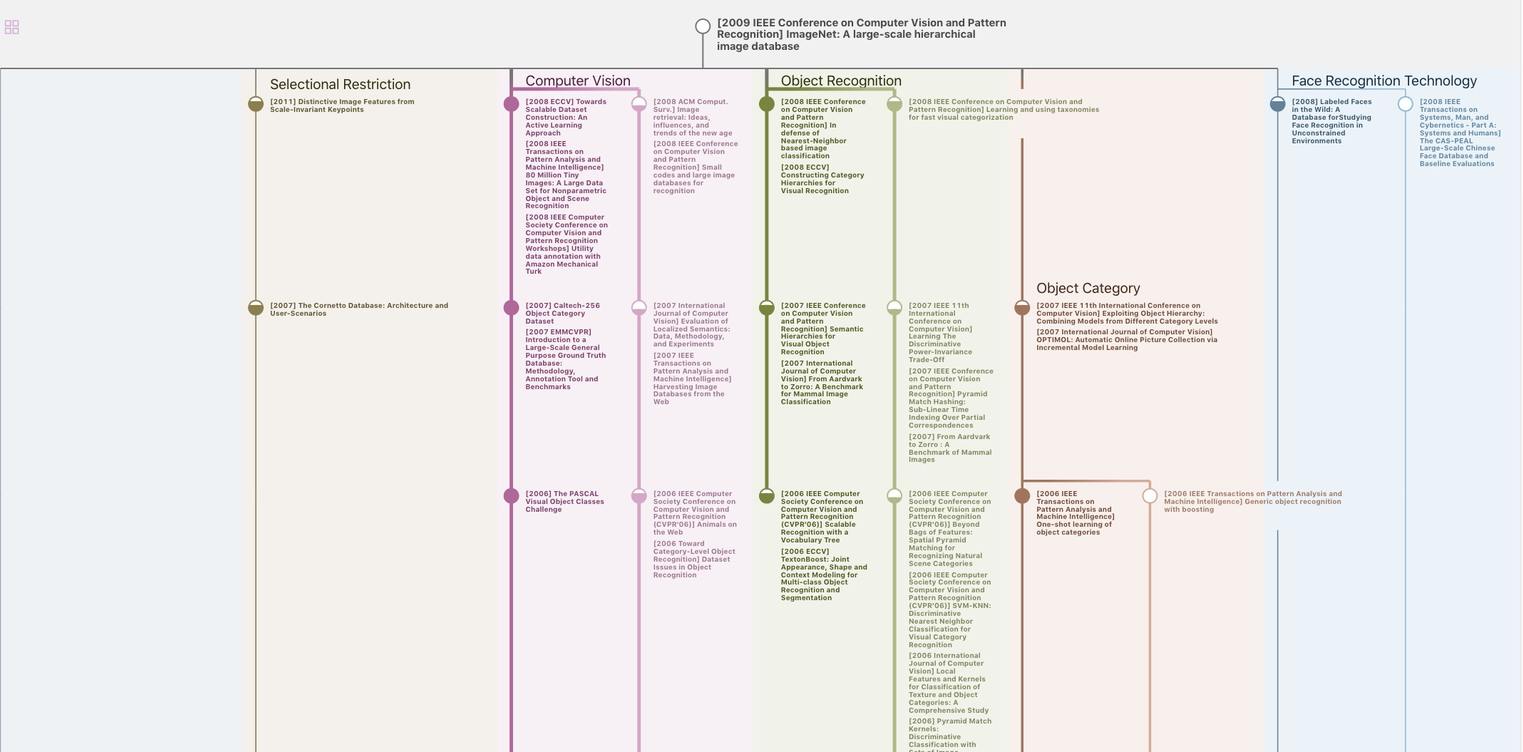
生成溯源树,研究论文发展脉络
Chat Paper
正在生成论文摘要