Active Feedback using Riemannian Features for Motor Imagery Classification
2023 International Conference on Bio Signals, Images, and Instrumentation (ICBSII)(2023)
摘要
Brain-Computer Interface (BCI) technologies employing electroencephalography (EEG) signals heavily depend on effective and accurate signal classification strategies. Researchers have extensively developed various machine learning (ML) algorithms. However, very little has been done to improve the user’s ability to elicit better brain patterns easily distinguishable across different stimuli. This paper proposes four feedback mechanisms through which the user trains himself for a motor imagery (MI) task via active feedback. The feedback strategies involve t-Distributed Stochastic Neighbor Embedding (t-SNE)-transformed Riemannian covariance matrices, mean correlations between C3 and C4 channels, tangent space transformed mean correlations between C3 and C4 channels and the power spectral density difference between C3 and C4 channels. Using a standard SVM classifier, the subjects showed significant improvement in MI accuracy post the training session. An increase in accuracy of more than 9% is achieved for two feedback mechanisms on an in-house dataset of 24 subjects indicating the effectiveness of proper feedback for eliciting better MI ability from users.
更多查看译文
关键词
Electroencephalogram (EEG),Brain-Computer Interfaces (BCI),Motor Imagery (MI),user feedback,Riemannian manifold
AI 理解论文
溯源树
样例
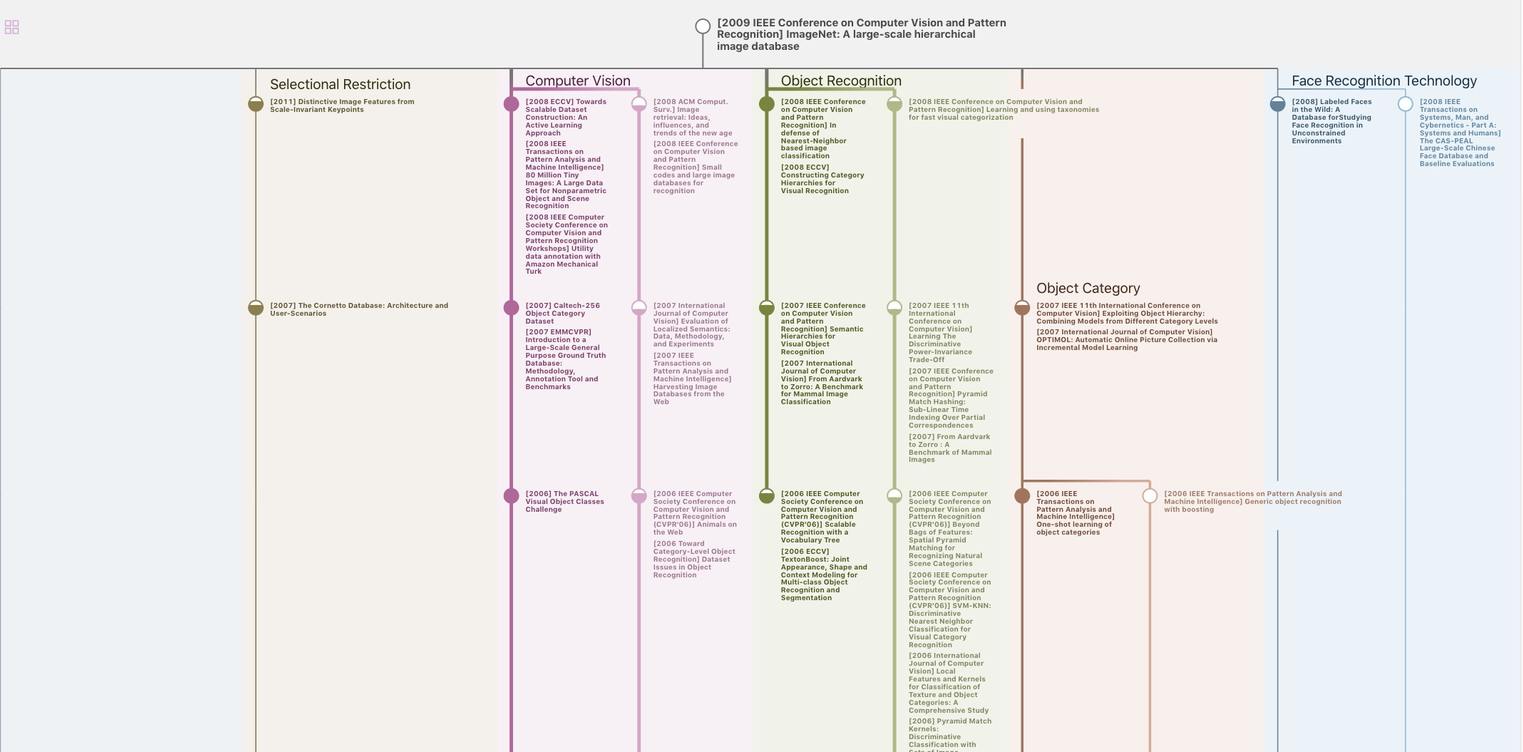
生成溯源树,研究论文发展脉络
Chat Paper
正在生成论文摘要