Joint Optimization of Bandwidth and Power Allocation in Uplink Systems with Deep Reinforcement Learning.
Sensors (Basel, Switzerland)(2023)
摘要
Wireless resource utilizations are the focus of future communication, which are used constantly to alleviate the communication quality problem caused by the explosive interference with increasing users, especially the inter-cell interference in the multi-cell multi-user systems. To tackle this interference and improve the resource utilization rate, we proposed a joint-priority-based reinforcement learning (JPRL) approach to jointly optimize the bandwidth and transmit power allocation. This method aims to maximize the average throughput of the system while suppressing the co-channel interference and guaranteeing the quality of service (QoS) constraint. Specifically, we de-coupled the joint problem into two sub-problems, i.e., the bandwidth assignment and power allocation sub-problems. The multi-agent double deep Q network (MADDQN) was developed to solve the bandwidth allocation sub-problem for each user and the prioritized multi-agent deep deterministic policy gradient (P-MADDPG) algorithm by deploying a prioritized replay buffer that is designed to handle the transmit power allocation sub-problem. Numerical results show that the proposed JPRL method could accelerate model training and outperform the alternative methods in terms of throughput. For example, the average throughput was approximately 10.4-15.5% better than the homogeneous-learning-based benchmarks, and about 17.3% higher than the genetic algorithm.
更多查看译文
关键词
uplink, multi-cell multi-user system, joint-priority-based reinforcement learning (JPRL), prioritized replay buffer, throughput
AI 理解论文
溯源树
样例
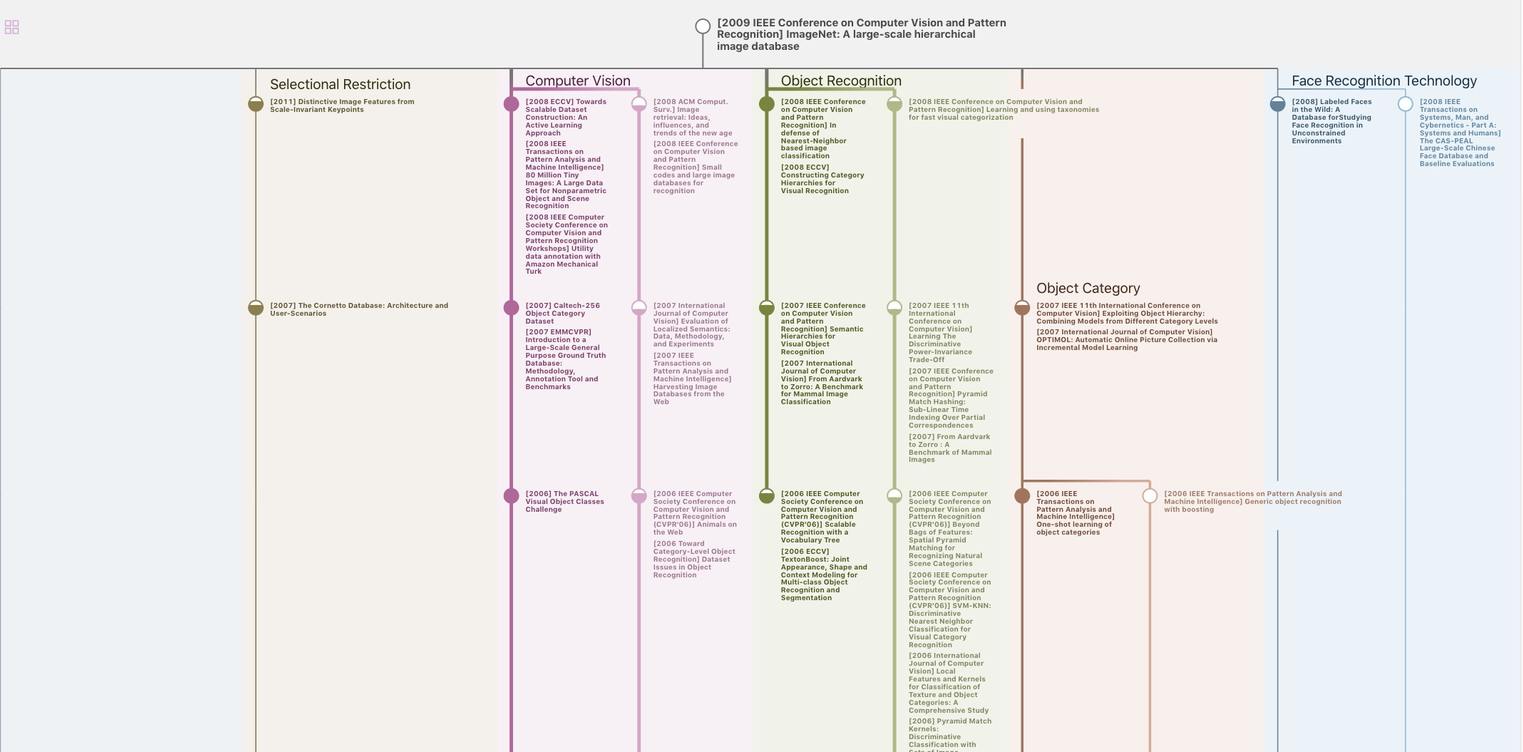
生成溯源树,研究论文发展脉络
Chat Paper
正在生成论文摘要