Graph attention network-optimized dynamic monocular visual odometry
APPLIED INTELLIGENCE(2023)
摘要
Monocular Visual Odometry (VO) is often formulated as a sequential dynamics problem that relies on scene rigidity assumption. One of the main challenges is rejecting moving objects and estimating camera pose in dynamic environments. Existing methods either take the visual cues in the whole image equally or eliminate the fixed semantic categories by heuristics or attention mechanisms. However, they fail to tackle unknown dynamic objects which are not labeled in the training sets of the network. To solve these issues, we propose a novel framework, named graph attention network (GAT)-optimized dynamic monocular visual odometry (GDM-VO), to remove dynamic objects explicitly with semantic segmentation and multi-view geometry in this paper. Firstly, we employ a multi-task learning network to perform semantic segmentation and depth estimation. Then, we reject priori known and unknown objective moving objects through semantic information and multi-view geometry, respectively. Furthermore, to our best knowledge, we are the first to leverage GAT to capture long-range temporal dependencies from consecutive image sequences adaptively, while existing sequential modeling approaches need to select information manually. Extensive experiments on the KITTI and TUM datasets demonstrate the superior performance of GDM-VO overs existing state-of-the-art classical and learning-based monocular VO.
更多查看译文
关键词
Monocular visual odometry,Multi-task learning,Multi-view geometry,Dynamic objects removal,Graph attention network
AI 理解论文
溯源树
样例
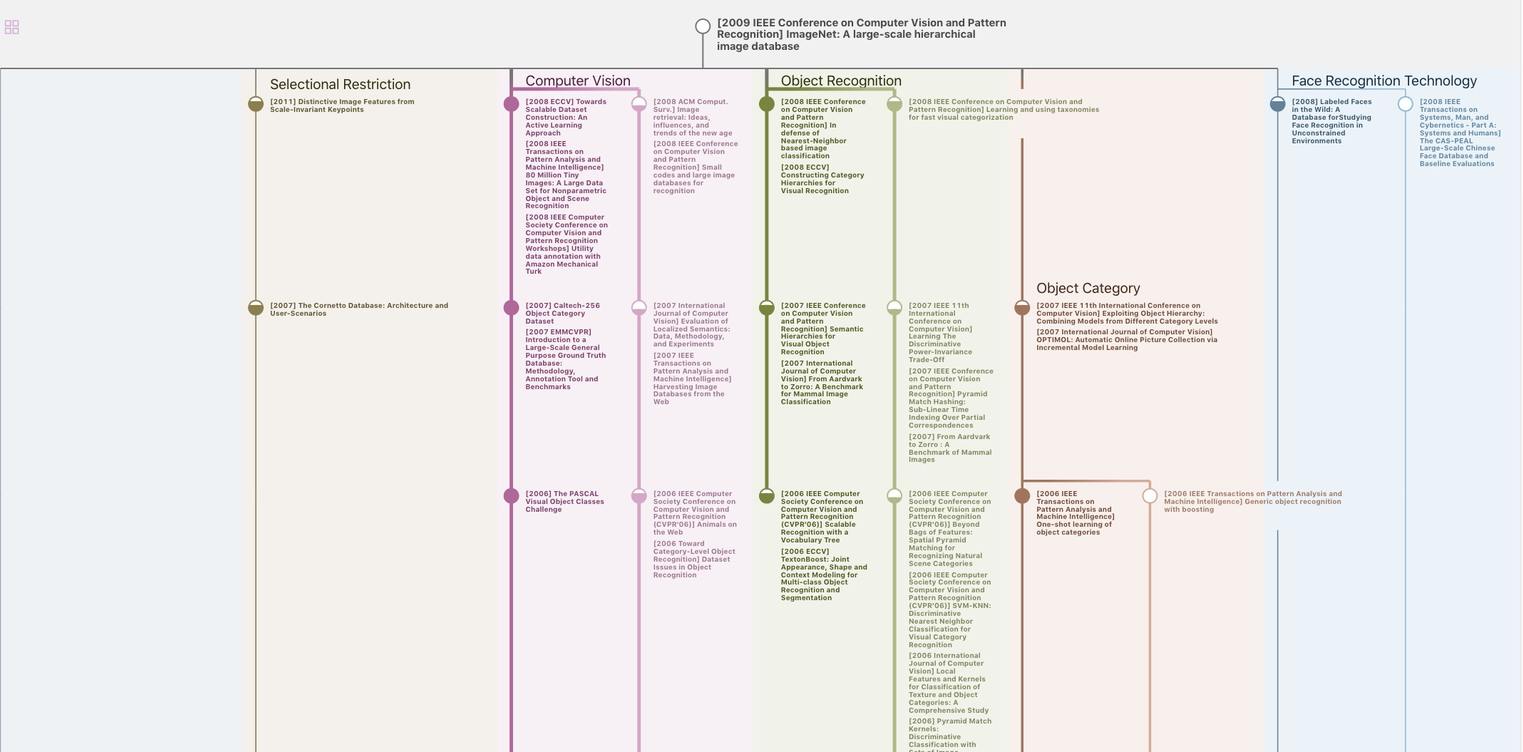
生成溯源树,研究论文发展脉络
Chat Paper
正在生成论文摘要