Comparing explanations in RL
NEURAL COMPUTING & APPLICATIONS(2024)
摘要
As deep reinforcement learning (RL)’s capabilities surpass traditional reinforcement learning, the community is working to make these black boxes less opaque. Explanations about algorithms’ choices and strategies serve this purpose. However, information about RL algorithms’ operations is not easily accessible. Our research aimed to extract such information, use it to build explanations, and test those explanations with users. For our user study, eight RL agents were trained using OpenAI baselines. Then, the HIGHLIGHTS-VIS algorithm was created by altering previous HIGHLIGHTS algorithms to collect data about the agents’ interactions with the environment. The data were used to create explanations that were compared to previous works’ video-based summaries in a user study. The between-subjects user study had participants answer questions about different agents. Participants were measured using both self-reported trust and performance on downstream tasks. Downstream tasks are tasks that a participant is more likely to do correctly with the information contained in the explanation. Collecting data about both trust and the utility of explanations allowed comparison and analysis of the explanations’ effectiveness. Results showed that the alternative explanations built from the collected data led to more correct answers about the agents and their strategies. Additionally, explanations’ utility depended on the context. Finally, users’ reported trust in an explanation did not directly correlate to performance. These results suggest trust and effectiveness may need to be measured and calibrated separately in future examinations of explanations.
更多查看译文
关键词
Interpretable machine learning,Explanation design,Appropriate trust,Human-centered computing,Human computer interaction (HCI) design and evaluation methods,User studies
AI 理解论文
溯源树
样例
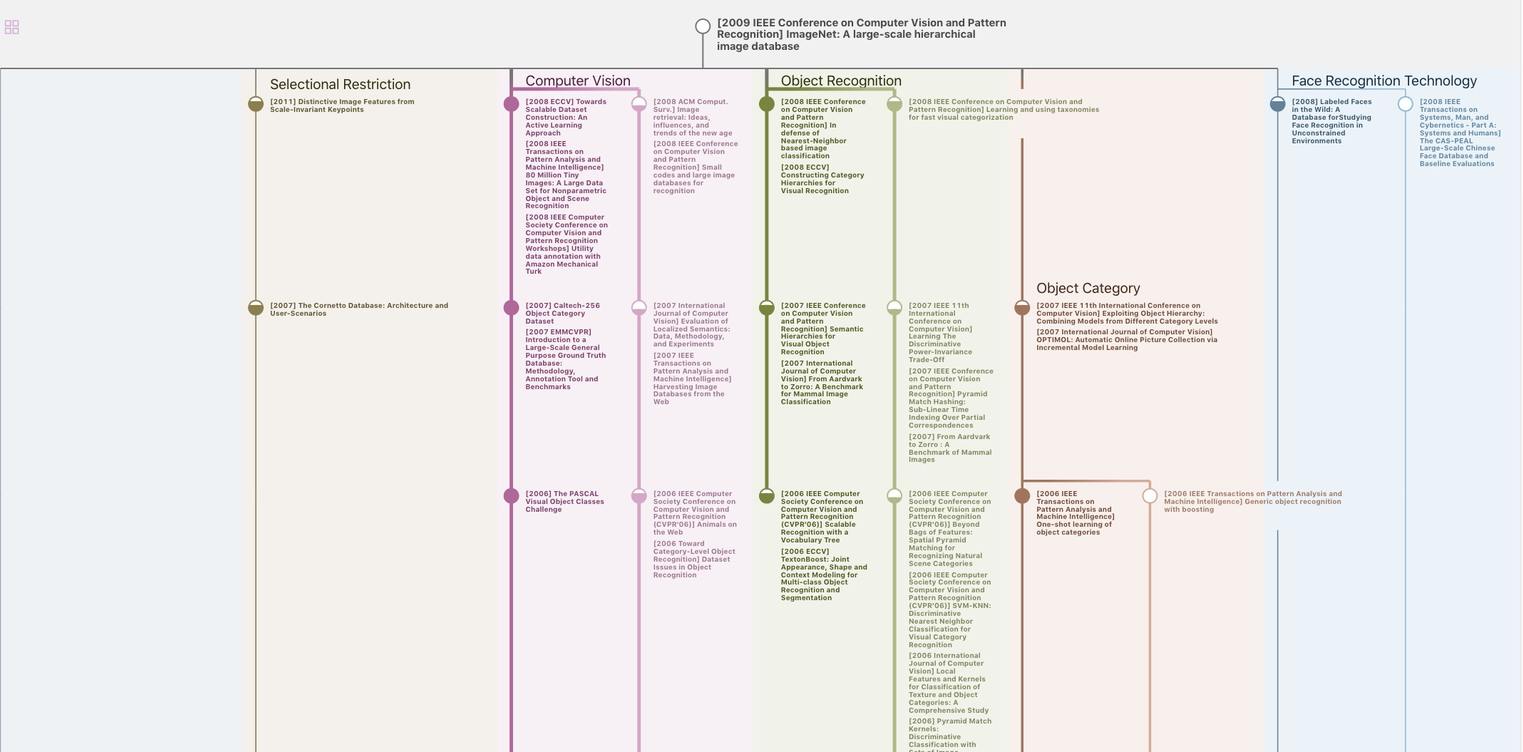
生成溯源树,研究论文发展脉络
Chat Paper
正在生成论文摘要