Anomaly detection in the probability simplex under different geometries
Information Geometry(2023)
摘要
An open problem in data science is that of anomaly detection. Anomalies are instances that do not maintain a certain property that is present in the remaining observations in a dataset. Several anomaly detection algorithms exist, since the process itself is ill-posed mainly because the criteria that separates common or expected vectors from anomalies are not unique. In the most extreme case, data is not labelled and the algorithm has to identify the vectors that are anomalous, or assign a degree of anomaly to each vector. The majority of anomaly detection algorithms do not make any assumptions about the properties of the feature space in which observations are embedded, which may affect the results when those spaces present certain properties. For instance, compositional data such as normalized histograms, that can be embedded in a probability simplex, constitute a particularly relevant case. In this contribution, we address the problem of detecting anomalies in the probability simplex, relying on concepts from Information Geometry, mainly by focusing our efforts in the distance functions commonly applied in that context. We report the results of a series of experiments and conclude that when a specific distance-based anomaly detection algorithm relies on Information Geometry-related distance functions instead of the Euclidean distance, the performance is significantly improved.
更多查看译文
关键词
Anomaly detection,Probability simplex,Information geometry
AI 理解论文
溯源树
样例
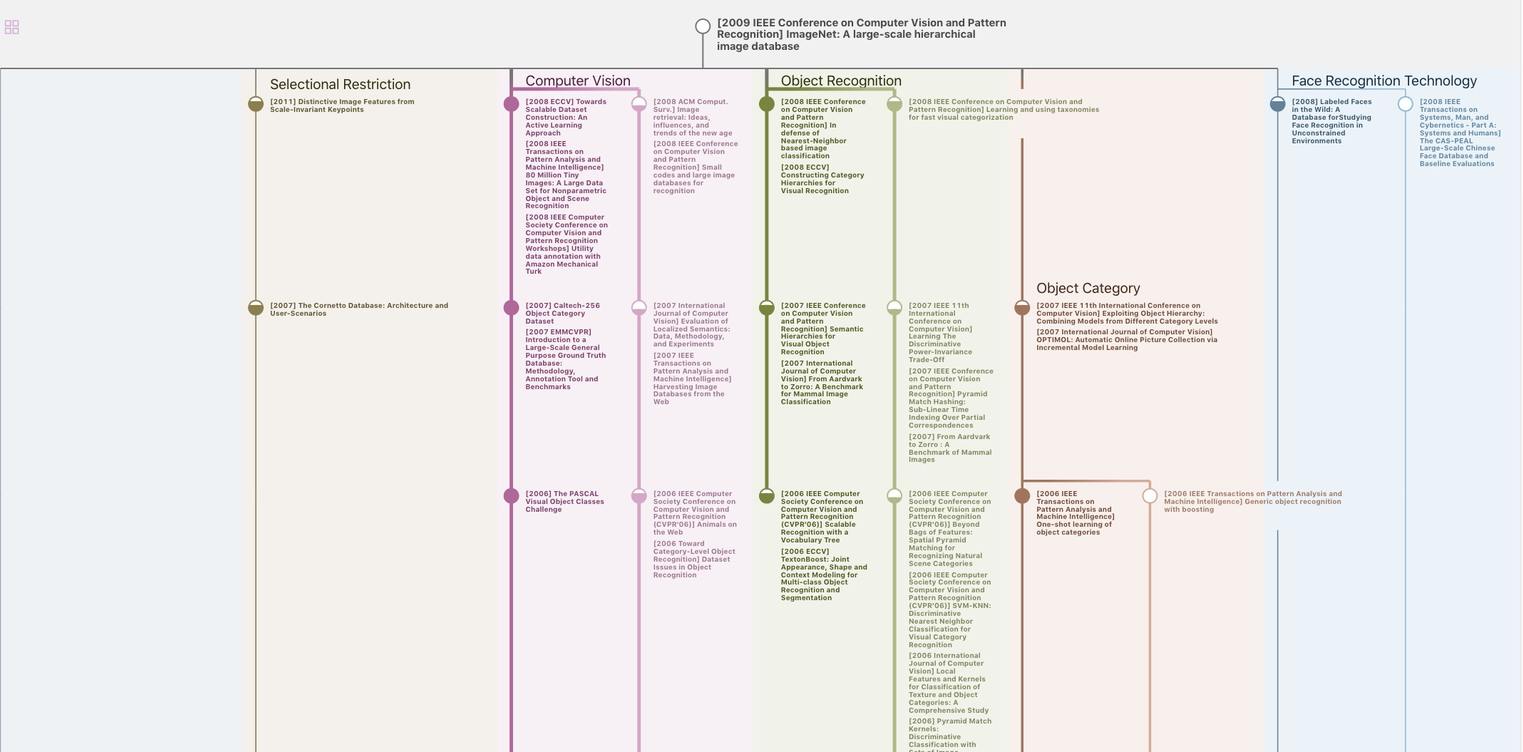
生成溯源树,研究论文发展脉络
Chat Paper
正在生成论文摘要