Class Disagreement Detection with Application to EO-SAR Fusion
AUTOMATIC TARGET RECOGNITION XXXIII(2023)
摘要
This paper considers the problem of aerial view object classification using co-registered electro-optical (EO) and synthetic aperture radar (SAR) images. Both EO and SAR sensors possess different advantages and drawbacks. There have been many research efforts focusing on joint multi-modal machine learning trying to take advantage of both modalities to develop a more performant classifier. These approaches usually assume the images produced by both modalities are consistent, meaning they contain the information of the same target. However, due to the limitation of EO sensor, it is not always true. For example, aerial viewed EO images may suffer from cloud occlusion. In some cases, inclusion of the cloud occluded EO images for inference may limit performace. This paper proposes an approach to detect if the EO-SAR chip pair contains cloud occluded EO image or not. We use the term "class disagreement detection" (CDD) to describe the mechanism to distinguish the normal EO chips from the corrupted EO chips by treating the corrupted EO chips as another class which is different from the class of the target in the corresponding SAR chips. The EOSAR-CDD machine-learning based approach is to encode EO and SAR features in a way that the distances between the features of the same classes are small while the distances between the features of different classes are large. The EOSAR-CCD can be utilized to construct a simple yet effective modality selection-based EO-SAR fusion scheme that outperforms a popular EO-SAR fusion scheme.
更多查看译文
关键词
Sensor fusion, aerial view object classification, joint training, EO-SAR fusion, co-registered EO-SAR images
AI 理解论文
溯源树
样例
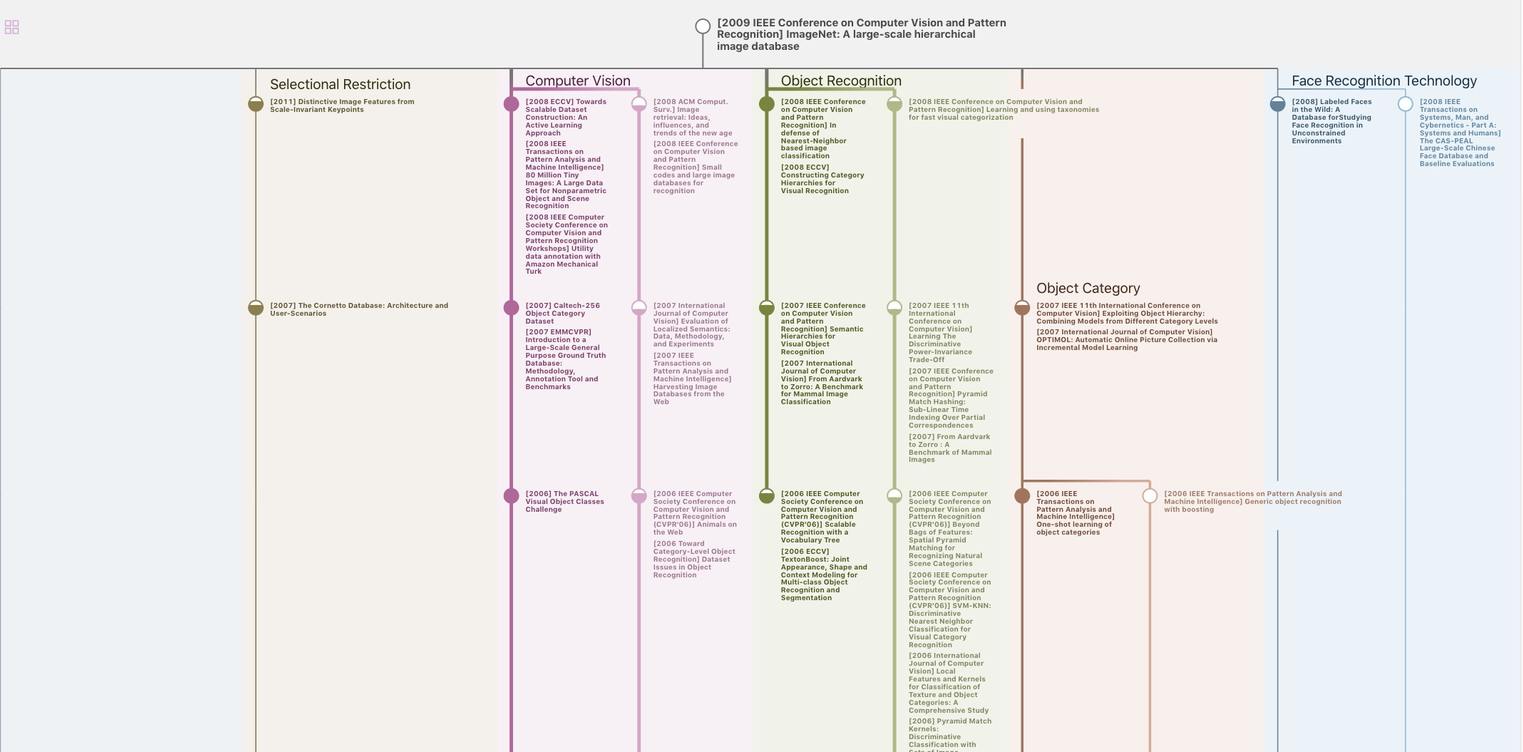
生成溯源树,研究论文发展脉络
Chat Paper
正在生成论文摘要