Quantifying quantum entanglement via a hybrid quantum-classical machine learning framework
PHYSICAL REVIEW A(2023)
摘要
Quantifying entanglement for quantum states whose density matrices are unknown is a difficult task, but also becomes more and more necessary because of the fast development of quantum engineering. Machine learning provides practical solutions to this fundamental problem, where one has to train a proper machine learning model to predict entanglement measures of unknown quantum states based on experimentally measurable data, say, moments of density matrices or correlation data produced by local measurements. In this paper, we compare the performance of these two different machine learning approaches systematically. Particularly, we first show that the approach based on moments enjoys a remarkable advantage over that based on correlation data, though the cost of measuring moments is much higher. Next, since correlation data is much easier to obtain experimentally, we try to better its performance by proposing a hybrid quantum-classical machine learning framework for this problem, where the key is to train optimal local measurements, or even optimal tensor products of single-qubit observables, to generate more informative correlation data. Our numerical simulations show that the new hybrid framework brings us comparable performance with the approach based on moments to quantify entanglement, and furthermore, it turns out that the new hybrid framework has a nice noise-resistant capability when handling realistic correlation data.
更多查看译文
关键词
quantum entanglement,machine learning,quantum-classical
AI 理解论文
溯源树
样例
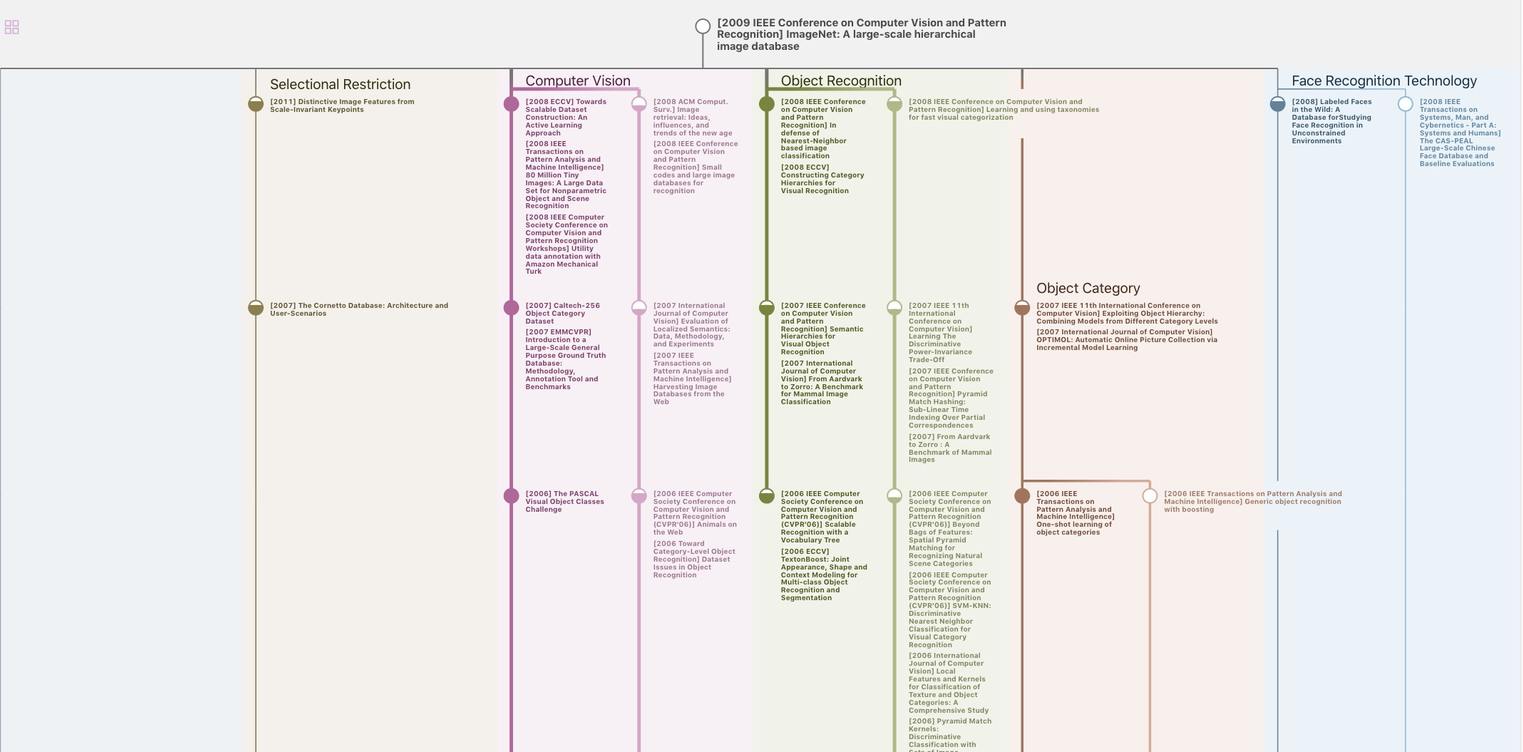
生成溯源树,研究论文发展脉络
Chat Paper
正在生成论文摘要