Spatial-temporal gated graph convolutional network: a new deep learning framework for long-term traffic speed forecasting.
J. Intell. Fuzzy Syst.(2023)
摘要
The key to solving traffic congestion is the accurate traffic speed forecasting. However, this is difficult owing to the intricate spatial-temporal correlation of traffic networks. Most existing studies either ignore the correlations among distant sensors, or ignore the time-varying spatial features, resulting in the inability to extract accurate and reliable spatial-temporal features. To overcome these shortcomings, this study proposes a new deep learning framework named spatial-temporal gated graph convolutional network for long-term traffic speed forecasting. Firstly, a new spatial graph generation method is proposed, which uses the adjacency matrix to generate a global spatial graph with more comprehensive spatial features. Then, a new spatial-temporal gated recurrent unit is proposed to extract the comprehensive spatial-temporal features from traffic data by embedding a new graph convolution operation into gated recurrent unit. Finally, a new self-attention block is proposed to extract global features from the traffic data. The evaluation on two real-world traffic speed datasets demonstrates the proposed model can accurately forecast the long-term traffic speed, and outperforms the baseline models in most evaluation metrics.
更多查看译文
关键词
Traffic speed forecasting,graph convolution operation,gated recurrent unit,self-attention block
AI 理解论文
溯源树
样例
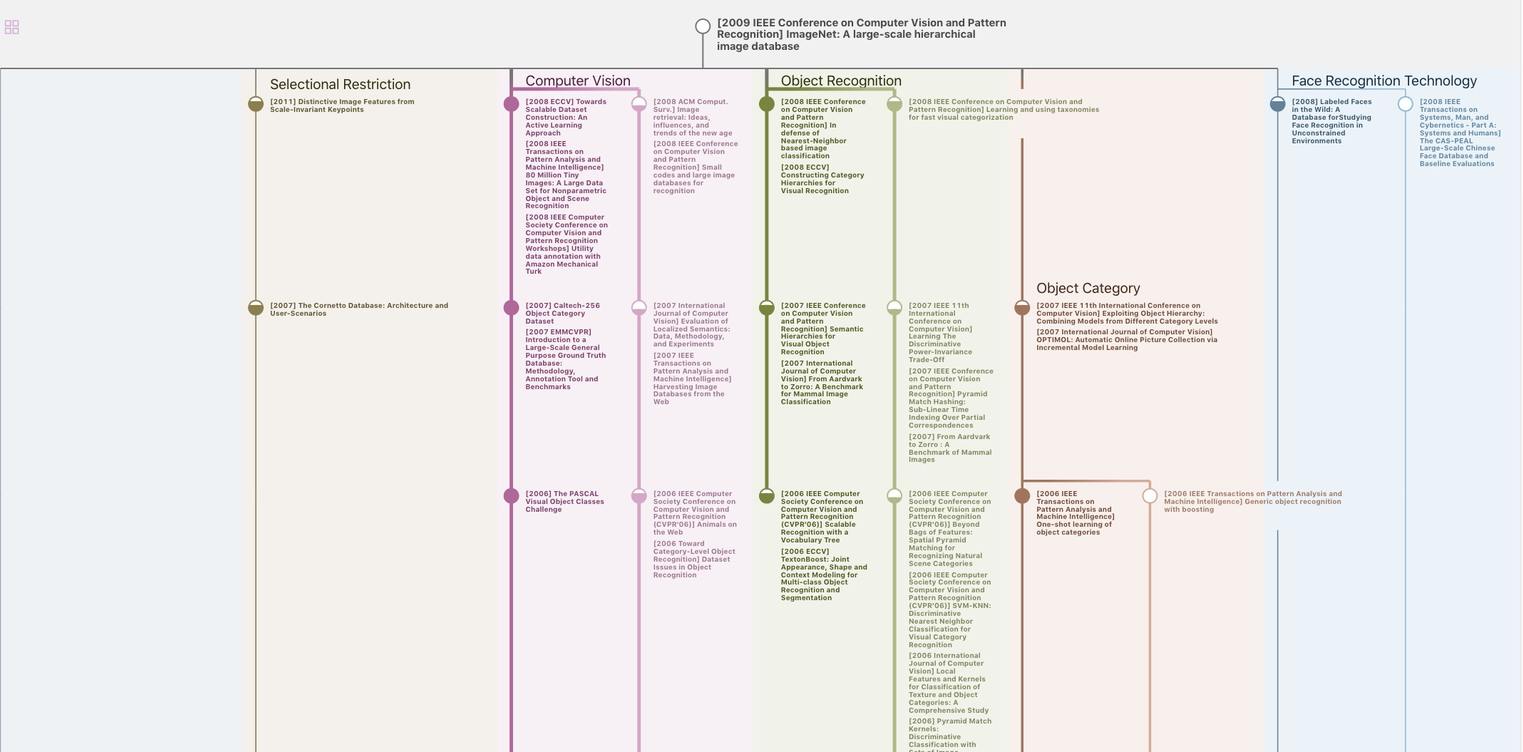
生成溯源树,研究论文发展脉络
Chat Paper
正在生成论文摘要