A deep feature matching pipeline with triple search strategy
JOURNAL OF SUPERCOMPUTING(2023)
摘要
Local feature matching between images is a challenging task, and current research focuses on pursuing higher accuracy matching results at the cost of higher time consumption and resource consumption, e.g., using multilayer search strategies to obtain higher matching accuracy. On the other hand, low-time consumption methods perform poorly in matching accuracy, such as using a coarse-to-fine strategy due to the loss of information of many feature maps resulting in lower matching accuracy. To address the above problems, we propose a matching pipeline that balances matching accuracy and time consumption. This pipeline uses a triple search strategy to search the information on three feature maps for local feature matching, which can obtain both higher matching accuracy than the coarse-to-fine method and lower computational complexity than the hierarchical strategy method, thus achieving a balance between accuracy and time consumption. In our pipeline, a pre-trained network is used as the backbone to generate feature maps from different layers. In addition, we collect the coarse matches and geometric transformations of the coarse feature maps. Then, local feature maps centered on matching points are cropped from the middle feature maps for refinement matching. After this step, the positioning of the refined middle matches on the fine layer feature map can be obtained with high accuracy. Extensive experiments are conducted on the Hpatches, IMC2020, and Aachen Day–Night datasets to demonstrate the effectiveness of the proposed pipeline, which is competitive with the current state-of-the-art methods.
更多查看译文
关键词
Image matching,Triple search strategy,Pre-trained network
AI 理解论文
溯源树
样例
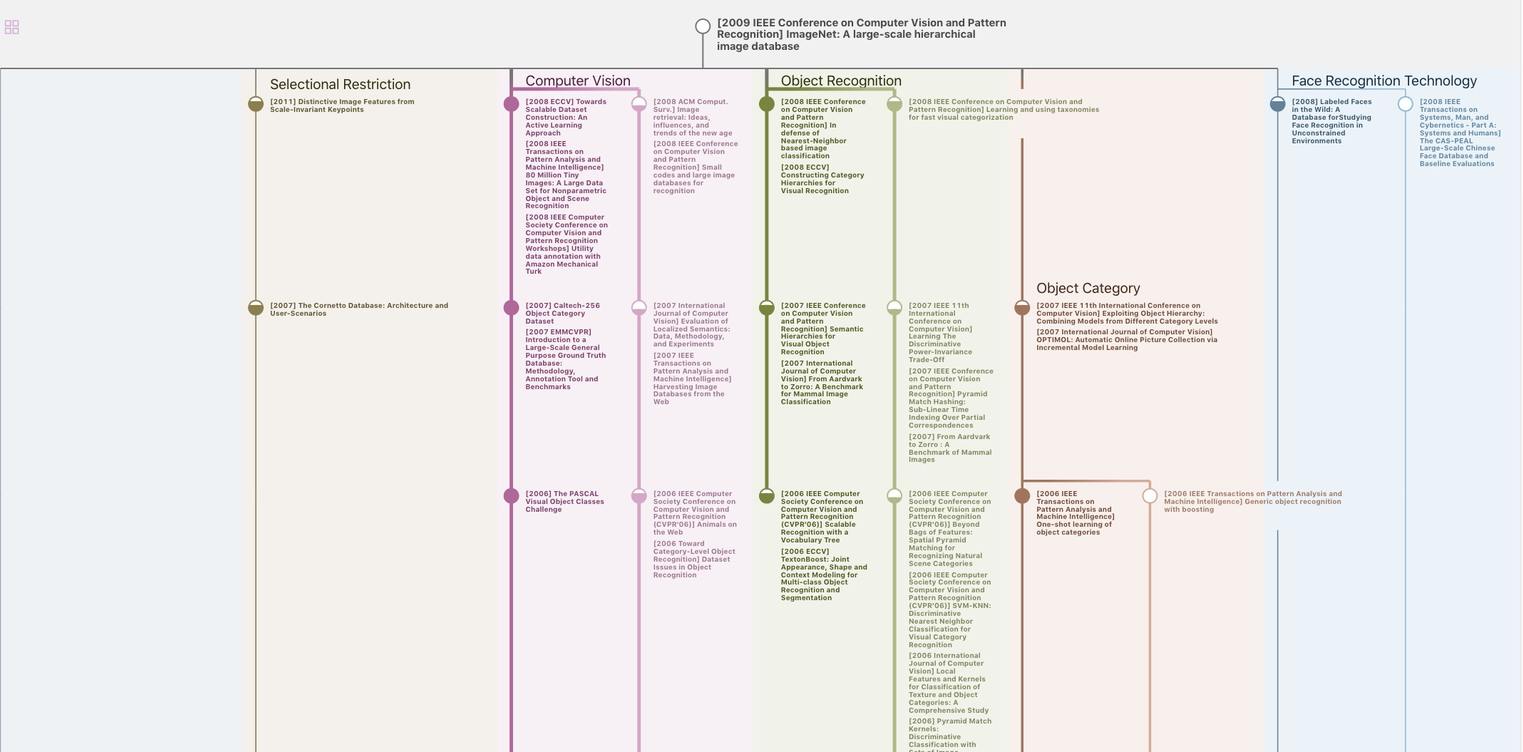
生成溯源树,研究论文发展脉络
Chat Paper
正在生成论文摘要