Deep learning framework for early detection of COVID-19 using X-ray images
MULTIMEDIA TOOLS AND APPLICATIONS(2024)
摘要
The whole world is imposing efforts to combat the deadly COVID-19 virus that continues to have a disastrous effect on health, economy, education, transport & communication, and many other sectors. The crucial action taken to control its rapid spread is first to detect the infected person. Deep learning-based algorithms utilize mathematical models to detect Covid-19 cases. Deep learning approach is applied to track and diagnose Covid-19 and help radiologists and medical doctors enhance prognosis performance. X-ray images are popularly used deep learning methods for Covid-19 detection. However, the existing techniques suffer from several limitations that need to be addressed to detect Covid-19 cases more accurately: Firstly, there is a small number of Covid-19 images. Secondly, an unbalanced dataset. Thirdly, model overfitting, and fourthly, correct detection of Covid-19 and pneumonia cases sometimes does not provide accurate results because COVID-19 and pneumonia symptoms are similar. Therefore, this paper aimed to develop an automated solution to classify the detected Covid-19 into two classes to overcome the small and unbalanced dataset, and model overfitting problems. This study compared nine state-of-the-art CNN architectures through a transfer learning approach. Our approach achieved better results in comparison to the work done on this benchmark dataset yielding 99.86% accuracy with 99.9% recall using VGG-16 using deep learning model. The proposed framework presents a transfer learning technique to increase the performance of the deep learning-based Covid-19 detection method. Moreover, the Comparative evaluation presents that the proposed framework outperforms existing methods. Close results show that VGG-16 on a large dataset correctly identified COVID-19.
更多查看译文
关键词
Covid-19,Deep learning,Prediction,Transfer learning,Chest X-ray
AI 理解论文
溯源树
样例
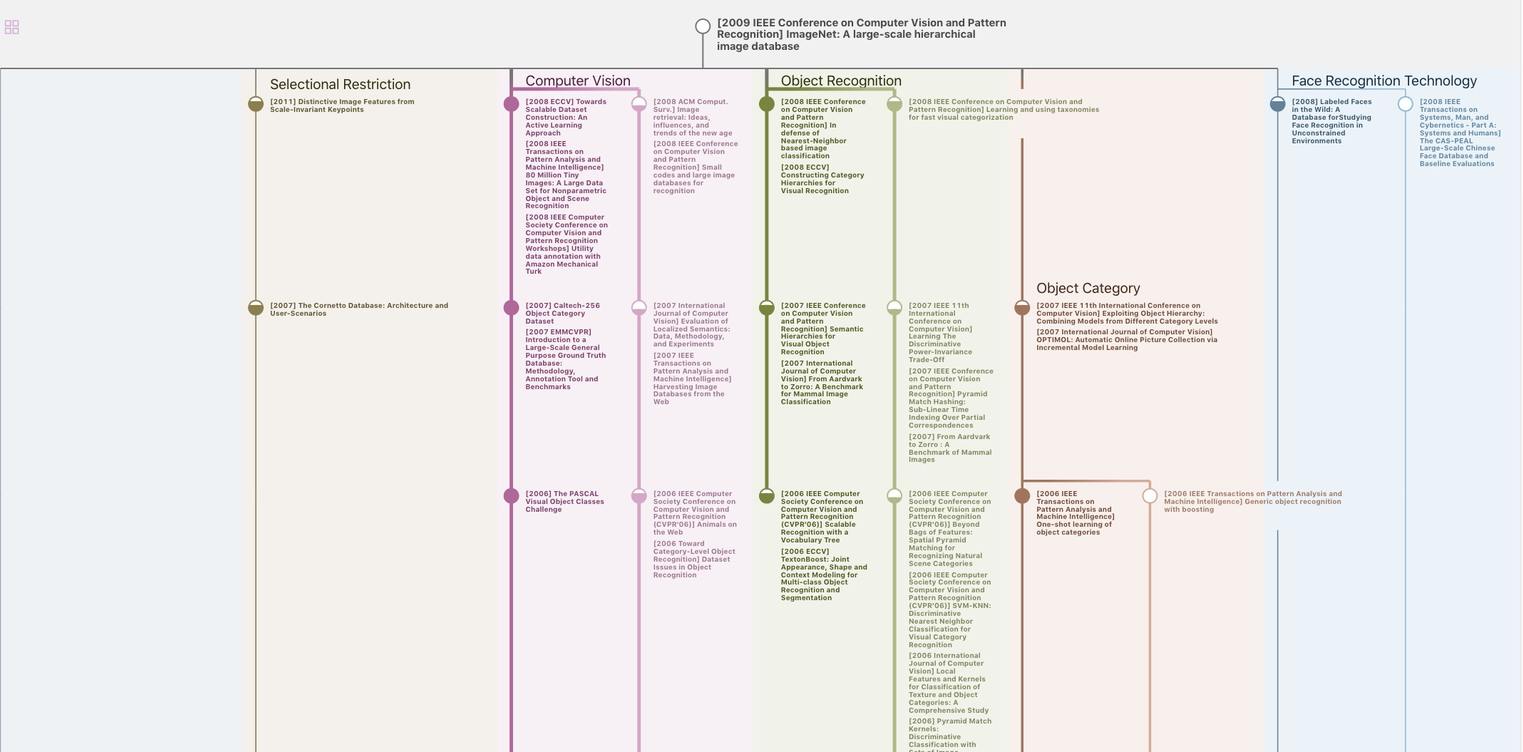
生成溯源树,研究论文发展脉络
Chat Paper
正在生成论文摘要