High-throughput cryo-ET structural pattern mining by unsupervised deep iterative subtomogram clustering
PROCEEDINGS OF THE NATIONAL ACADEMY OF SCIENCES OF THE UNITED STATES OF AMERICA(2023)
摘要
Cryoelectron tomography directly visualizes heterogeneous macromolecular structures in their native and complex cellular environments. However, existing computer assisted structure sorting approaches are low throughput or inherently limited due to their dependency on available templates and manual labels. Here, we introduce a high-throughput template-and-label-free deep learning approach, Deep Iterative Subtomogram Clustering Approach (DISCA), that automatically detects subsets of homogeneous structures by learning and modeling 3D structural features and their distributions. Evaluation on five experimental cryo-ET datasets shows that an unsupervised deep learning based method can detect diverse structures with a wide range of molecular sizes. This unsupervised detection paves the way for systematic unbiased recognition of macromolecular complexes in situ.
更多查看译文
关键词
structural pattern mining,high-throughput
AI 理解论文
溯源树
样例
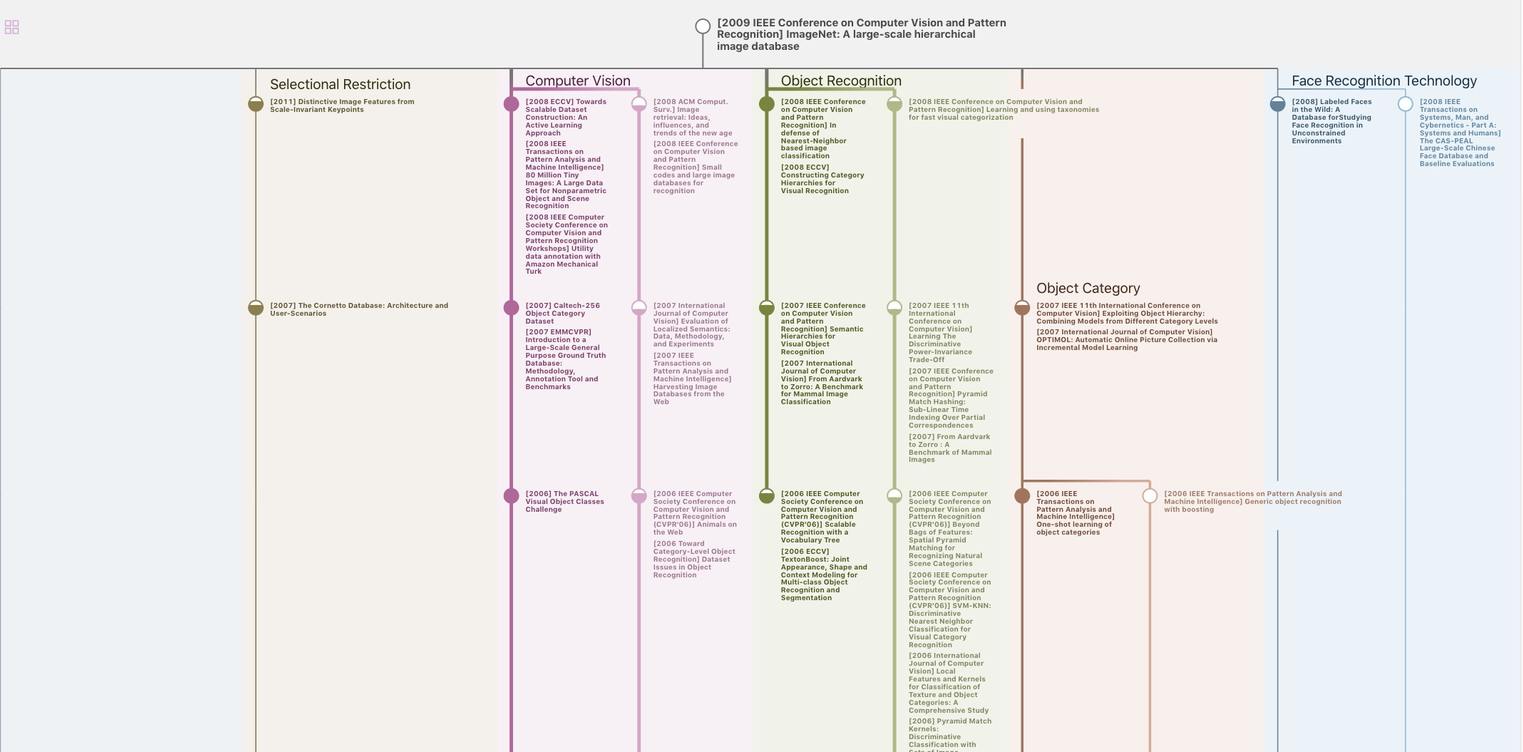
生成溯源树,研究论文发展脉络
Chat Paper
正在生成论文摘要