AdaptivePose++: A Powerful Single-Stage Network for Multi-Person Pose Regression
arXiv (Cornell University)(2022)
摘要
Multi-person pose estimation generally follows top-down and bottom-up paradigms. Both of them use an extra stage ($\boldsymbol{e.g.,}$ human detection in top-down paradigm or grouping process in bottom-up paradigm) to build the relationship between the human instance and corresponding keypoints, thus leading to the high computation cost and redundant two-stage pipeline. To address the above issue, we propose to represent the human parts as adaptive points and introduce a fine-grained body representation method. The novel body representation is able to sufficiently encode the diverse pose information and effectively model the relationship between the human instance and corresponding keypoints in a single-forward pass. With the proposed body representation, we further deliver a compact single-stage multi-person pose regression network, termed as AdaptivePose. During inference, our proposed network only needs a single-step decode operation to form the multi-person pose without complex post-processes and refinements. We employ AdaptivePose for both 2D/3D multi-person pose estimation tasks to verify the effectiveness of AdaptivePose. Without any bells and whistles, we achieve the most competitive performance on MS COCO and CrowdPose in terms of accuracy and speed. Furthermore, the outstanding performance on MuCo-3DHP and MuPoTS-3D further demonstrates the effectiveness and generalizability on 3D scenes. Code is available at https://github.com/buptxyb666/AdaptivePose.
更多查看译文
关键词
adaptivepose++,regression,network,single-stage,multi-person
AI 理解论文
溯源树
样例
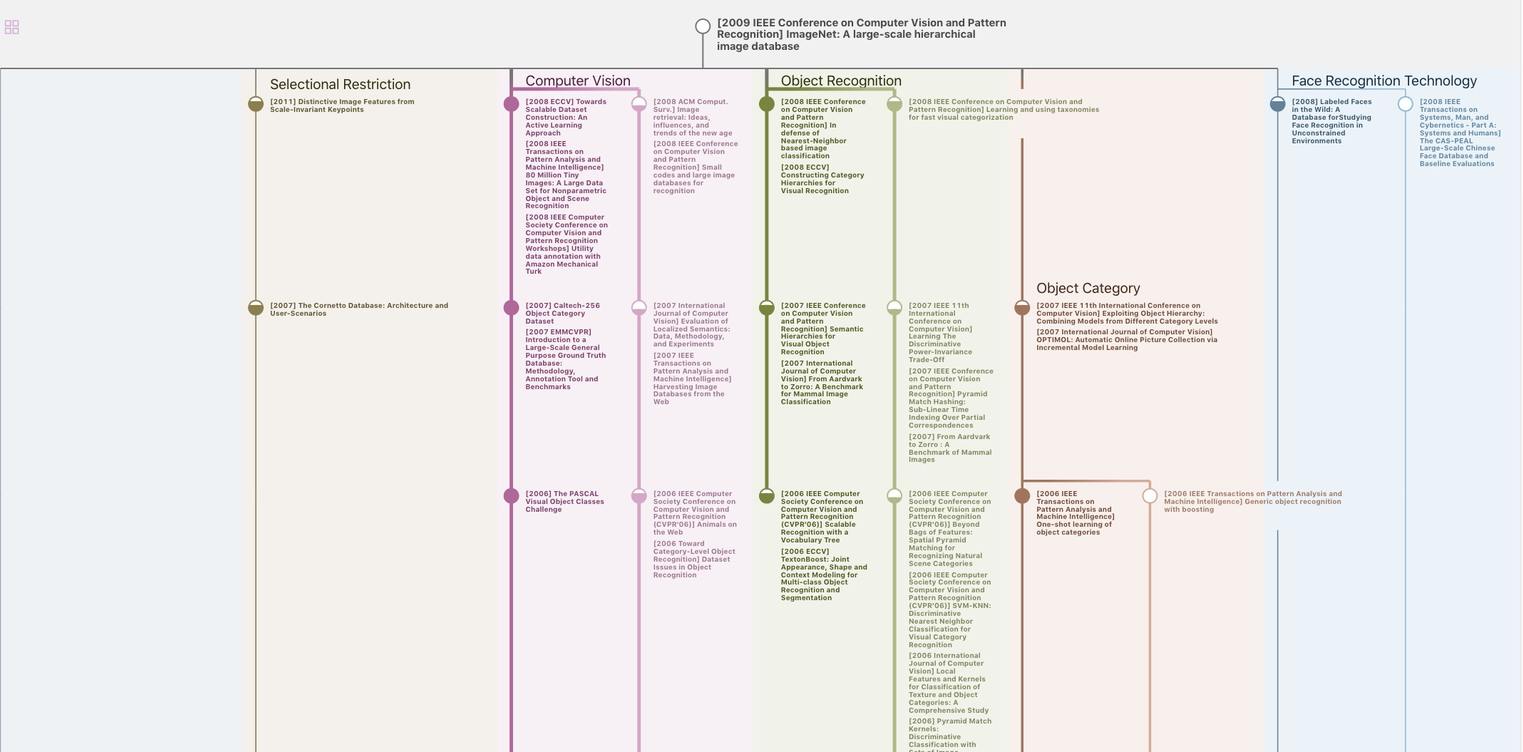
生成溯源树,研究论文发展脉络
Chat Paper
正在生成论文摘要