Variable importance for causal forests: breaking down the heterogeneity of treatment effects
HAL (Le Centre pour la Communication Scientifique Directe)(2023)
摘要
Causal random forests provide efficient estimates of heterogeneous treatment effects. However, forest algorithms are also well-known for their black-box nature, and therefore, do not characterize how input variables are involved in treatment effect heterogeneity, which is a strong practical limitation. In this article, we develop a new importance variable algorithm for causal forests, to quantify the impact of each input on the heterogeneity of treatment effects. The proposed approach is inspired from the drop and relearn principle, widely used for regression problems. Importantly, we show how to handle the forest retrain without a confounding variable. If the confounder is not involved in the treatment effect heterogeneity, the local centering step enforces consistency of the importance measure. Otherwise, when a confounder also impacts heterogeneity, we introduce a corrective term in the retrained causal forest to recover consistency. Additionally, experiments on simulated, semi-synthetic, and real data show the good performance of our importance measure, which outperforms competitors on several test cases. Experiments also show that our approach can be efficiently extended to groups of variables, providing key insights in practice.
更多查看译文
关键词
causal forests,variable importance,treatment effects
AI 理解论文
溯源树
样例
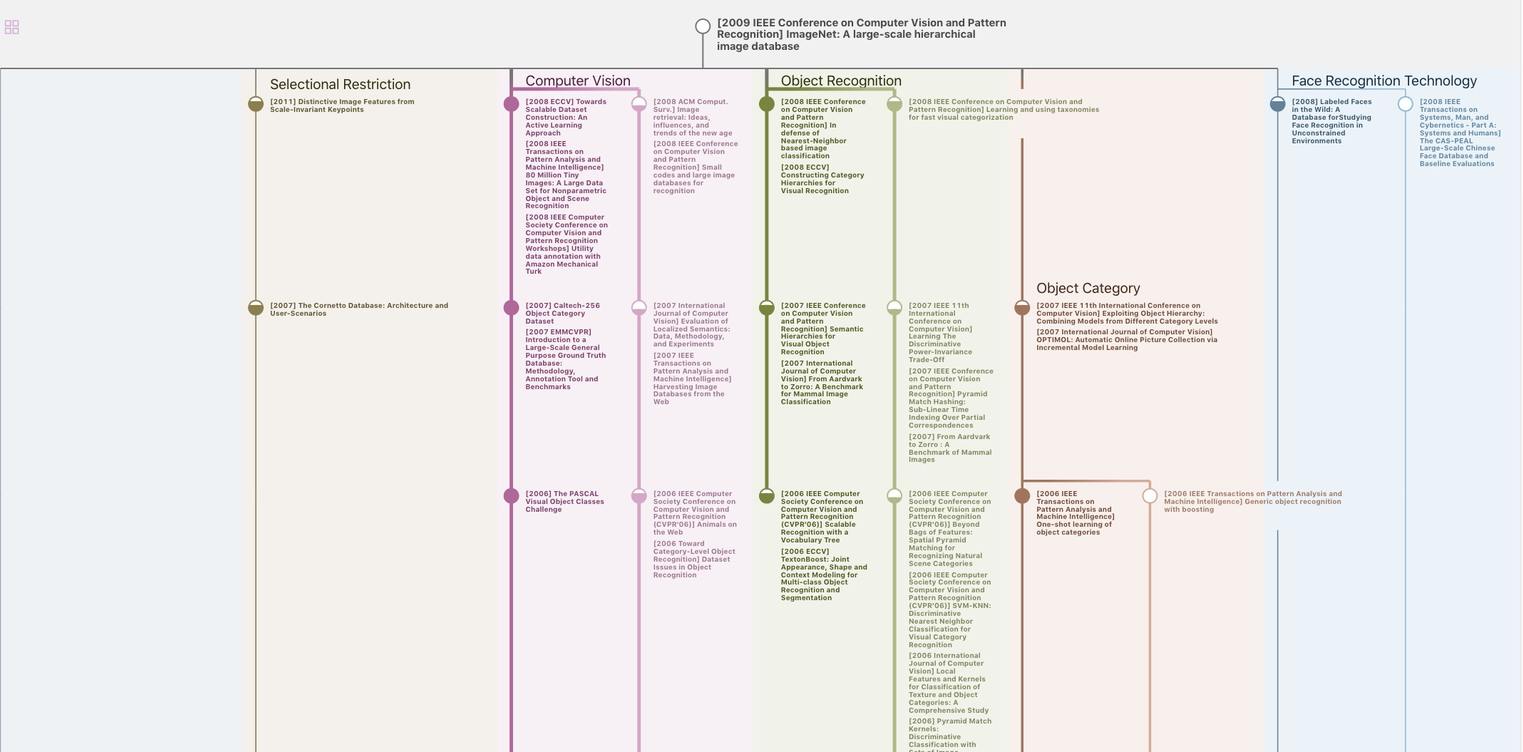
生成溯源树,研究论文发展脉络
Chat Paper
正在生成论文摘要