The Impact of Group Membership Bias on the Quality and Fairness of Exposure in Ranking
SIGIR '24: Proceedings of the 47th International ACM SIGIR Conference on Research and Development in Information Retrieval(2023)
Abstract
When learning to rank from user interactions, search and recommender systems
must address biases in user behavior to provide a high-quality ranking. One
type of bias that has recently been studied in the ranking literature is when
sensitive attributes, such as gender, have an impact on a user's judgment about
an item's utility. For example, in a search for an expertise area, some users
may be biased towards clicking on male candidates over female candidates. We
call this type of bias group membership bias. Increasingly, we seek rankings
that are fair to individuals and sensitive groups. Merit-based fairness
measures rely on the estimated utility of the items. With group membership
bias, the utility of the sensitive groups is under-estimated, hence, without
correcting for this bias, a supposedly fair ranking is not truly fair. In this
paper, first, we analyze the impact of group membership bias on ranking quality
as well as merit-based fairness metrics and show that group membership bias can
hurt both ranking and fairness. Then, we provide a correction method for group
bias that is based on the assumption that the utility score of items in
different groups comes from the same distribution. This assumption has two
potential issues of sparsity and equality-instead-of-equity; we use an
amortized approach to address these. We show that our correction method can
consistently compensate for the negative impact of group membership bias on
ranking quality and fairness metrics.
MoreTranslated text
AI Read Science
Must-Reading Tree
Example
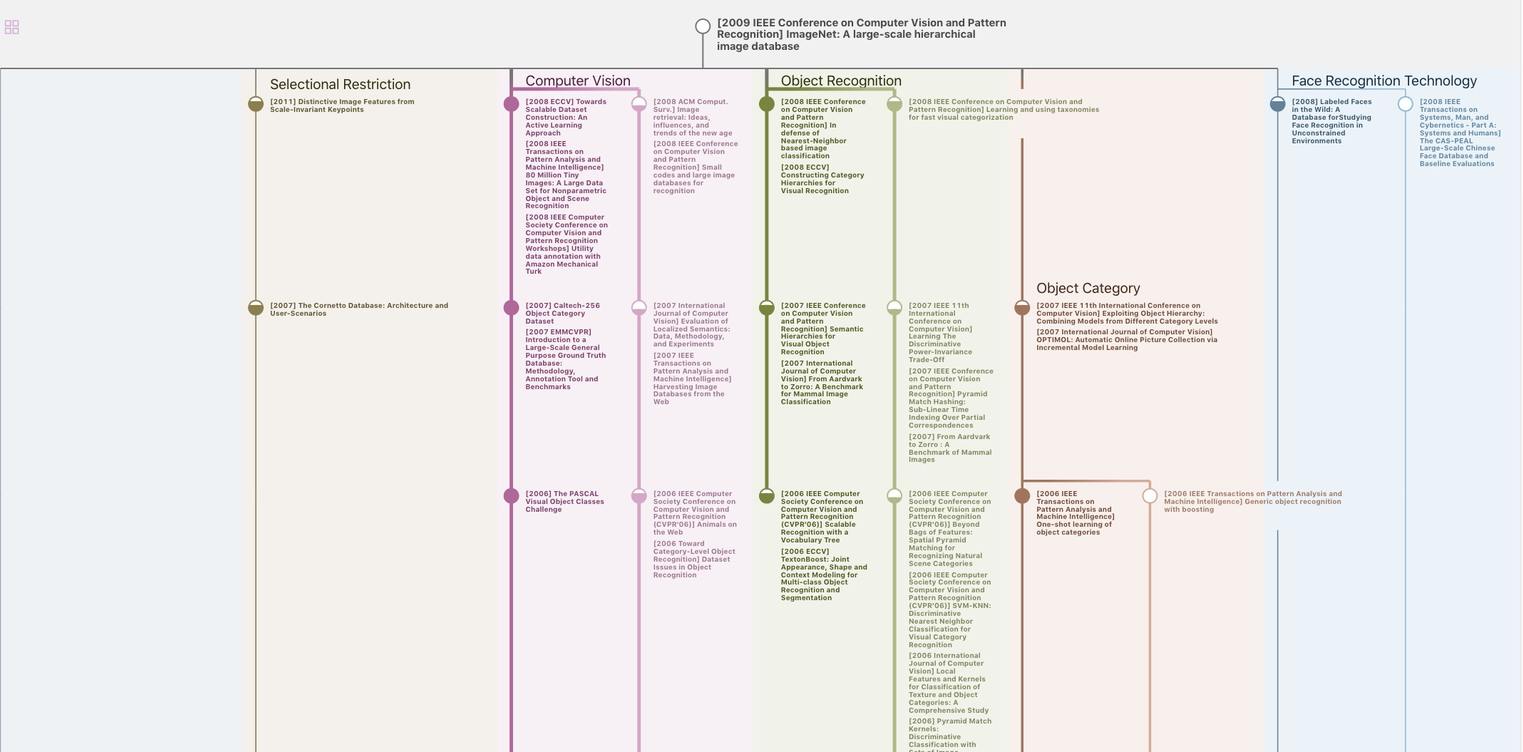
Generate MRT to find the research sequence of this paper
Chat Paper
Summary is being generated by the instructions you defined