Improvement of pattern recognition in spiking neural networks by modifying threshold parameter and using image inversion
Multimedia Tools and Applications(2024)
摘要
In recent years, spiking neural networks (SNNs) have gained popularity as a biologically plausible and energy-efficient alternative to artificial neural networks. Unlike non-spiking networks, SNNs use asynchronous, scattered pulses to communicate between spiking neurons, making them suitable for portable systems with limited hardware and energy resources. In this paper, we propose a feed-forward spiking neural network (SNN) with two spiking convolutional layers, one spiking classification layer, and a single non-spiking leaky integrator for readout. This network uses reverse pixel values and a type of time encoding known as constant-current-leaky integrate and fire (LIF) coding. Here, for the first time, the idea of adjusting the threshold voltage parameter of network neurons and inverting input images is introduced to improve recognition accuracy. We demonstrate that decreasing the threshold voltage parameter below its default voltage and optimizing input images by inverting pixel values can improve the recognition accuracy of the network. Using this approach, we test the network on the popular MNIST and Fashion-MNIST benchmarks, achieving test accuracies of 99.28% and 90.43%, respectively. We also test our approach on models from several studies and different datasets to prove its validity and generality. Results show that our proposed approach is effective in improving recognition accuracy compared to the initial accuracies of the models.
更多查看译文
关键词
Spiking neural networks (SNNs),Current-based LIF neuron model,Threshold voltage parameter,Constant-current-LIF encoding
AI 理解论文
溯源树
样例
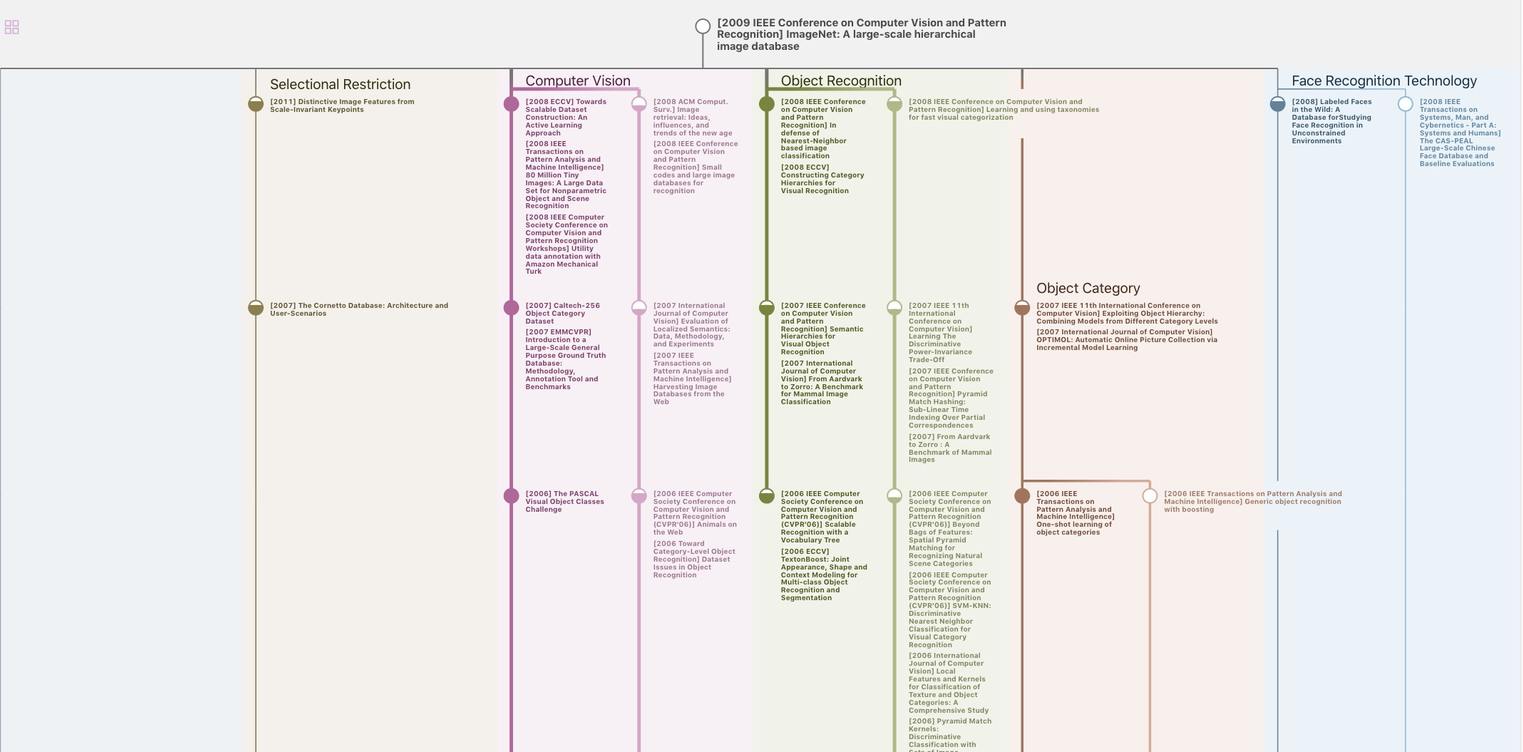
生成溯源树,研究论文发展脉络
Chat Paper
正在生成论文摘要