Unlocking the Potential of Similarity Matching: Scalability, Supervision and Pre-training
CoRR(2023)
摘要
While effective, the backpropagation (BP) algorithm exhibits limitations in terms of biological plausibility, computational cost, and suitability for online learning. As a result, there has been a growing interest in developing alternative biologically plausible learning approaches that rely on local learning rules. This study focuses on the primarily unsupervised similarity matching (SM) framework, which aligns with observed mechanisms in biological systems and offers online, localized, and biologically plausible algorithms. i) To scale SM to large datasets, we propose an implementation of Convolutional Nonnegative SM using PyTorch. ii) We introduce a localized supervised SM objective reminiscent of canonical correlation analysis, facilitating stacking SM layers. iii) We leverage the PyTorch implementation for pre-training architectures such as LeNet and compare the evaluation of features against BP-trained models. This work combines biologically plausible algorithms with computational efficiency opening multiple avenues for further explorations.
更多查看译文
关键词
similarity matching,supervision
AI 理解论文
溯源树
样例
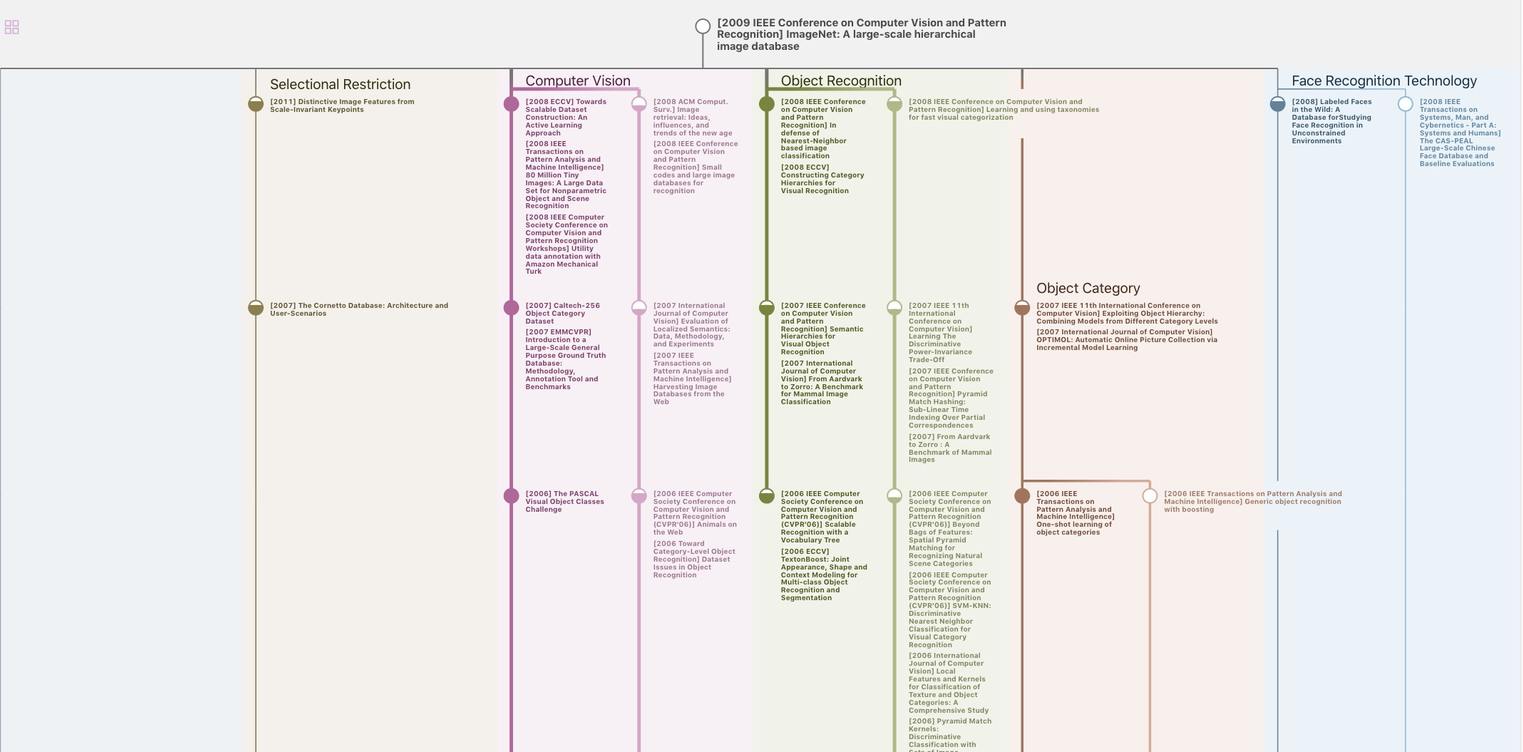
生成溯源树,研究论文发展脉络
Chat Paper
正在生成论文摘要