Deep Reinforcement Learning-Based Short-Term Traffic Signal Optimizing Using Disaggregated Vehicle Data
Data Science for Transportation(2023)
摘要
Adaptive traffic signals equipped with sensors are becoming increasingly important in enhancing the efficiency of existing transportation networks. Machine learning techniques are emerging as a promising tool for improving traffic operations. This paper adopts a Deep Q Network (DQN)-based reinforcement learning (RL) approach to control traffic signals. In DQN, an agent learns from interacting with the traffic signal through a sequence of observation, action, and reward. The agent observes the traffic conditions through a state matrix generated by individual vehicle position with the aim to make the agent’s actions control the signal phase selection in real time. In this study, the traffic scenario is developed using the traffic micro-simulator SUMO. The simulation model is calibrated using signal retiming, vehicle count, and speed obtained from real traffic data. The data are collected from nine signalized traffic intersections along State Road 50 in Orlando, Florida. Results show that the proposed deep reinforcement learning model reduces the waiting time by 18–53% and traffic conflict by 19–25% compared to the benchmark. Moreover, the proposed reinforcement learning method is effective for mobility and safety by optimizing traffic signal timing at multiple intersections.
更多查看译文
关键词
Adaptive traffic intersection,Reinforcement learning,Microscopic traffic simulation,Intelligent transportation system,Traffic safety
AI 理解论文
溯源树
样例
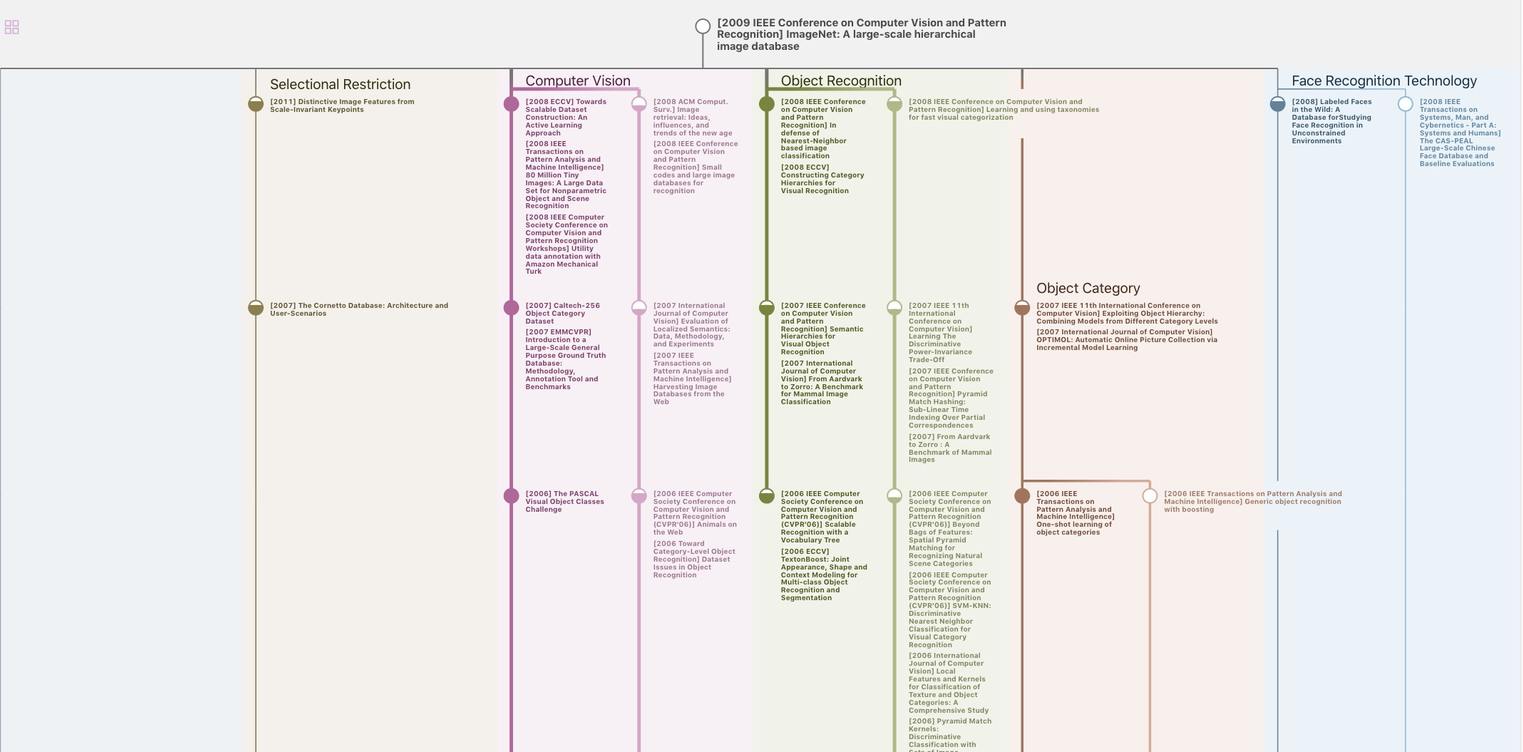
生成溯源树,研究论文发展脉络
Chat Paper
正在生成论文摘要