Estimating operationalized intent using random forests
Human-Intelligent Systems Integration(2022)
摘要
Teams of human operators and artificial intelligent agents (AIAs) in multi-agent systems present a unique set of challenges to team coordination. This research endeavors to employ a machine learning framework to estimate a set of ranks among quality goals, where the quality goals are designed to help communicate important elements of operator intent to aid the development of a Shared Mental Model among members in a multi-agent team. Using a representation referred to as the Operationalized Intent model to capture quality goals relevant to “how” the operator would like to execute the team’s mission, this paper details the development and evaluation of a random forest algorithm to estimate operator priorities. Estimation is structured as a label ranking problem in which quality goals, which constrain “how” work is to be conducted, are ranked according to their priority. Modifying an existing label ranking algorithm, we demonstrate that the Operationalized Intent Estimator-Random Forest (OIE-RF) can estimate quality goal rankings more accurately than a situation baseline which is derived by observing the variability among operators. OIE-RF demonstrates stability in dynamic testing and the ability to use explicit communication and operator identity to increase accuracy. This exploratory research opens a new avenue for improving coordination and performance of human-agent teams.
更多查看译文
关键词
Intent Estimation,Situation,Multi-Agent Systems,Human-Agent Interaction
AI 理解论文
溯源树
样例
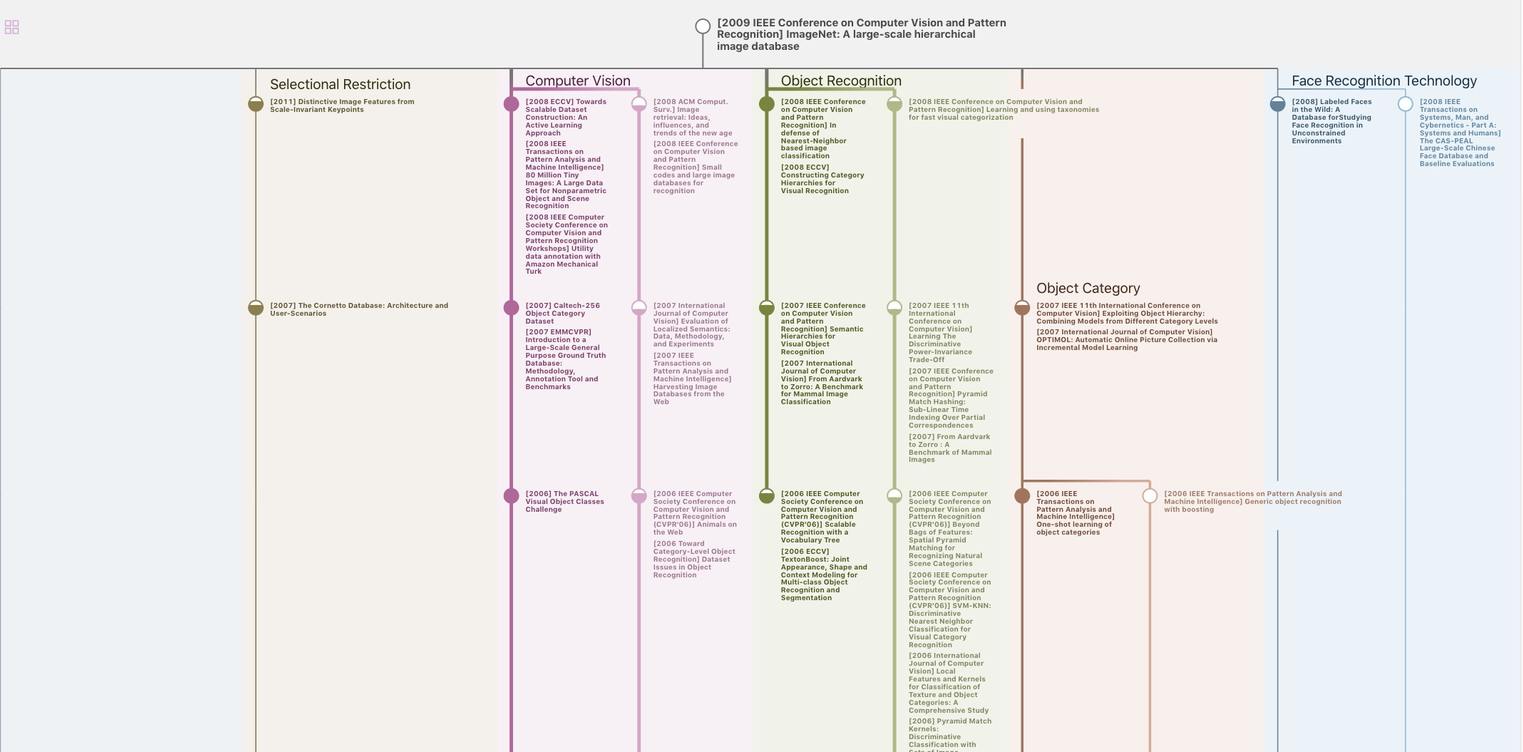
生成溯源树,研究论文发展脉络
Chat Paper
正在生成论文摘要