Mitigating Label Noise in GANs via Enhanced Spectral Normalization
IEEE Transactions on Circuits and Systems for Video Technology(2023)
摘要
Label noise is a ubiquitous issue in GANs, which degrades the generalization ability of the discriminator and usually leads to instability when training GANs. This issue stems from both real data and generated data. Previous works either only consider one of these two sources, or are not robust enough to noisy labels. In this paper, we revisit spectral normalization in robust learning with noisy labels. Based on its pros and cons, we propose to combine spectral normalization and weight decay to regularize the discriminator, which enjoys a more robust training process. To extend to conditional GANs, we propose to balance the relative importance of marginal matching and conditional matching in the projection discriminator. The proposed Enhanced Spectral Normalization for Generative Adversarial Networks (ESNGAN) can be easily integrated into various existing GANs frameworks without excessive additional cost. The effectiveness of the proposed method is validated on the CIFAR10, LSUN Church, CelebA, and ImageNet datasets, including the unconditional image generation task and the class-conditional image generation task. We also show that the proposed method can further improve the performance of the high-resolution image generation task.
更多查看译文
关键词
gans,label noise,enhanced spectral normalization
AI 理解论文
溯源树
样例
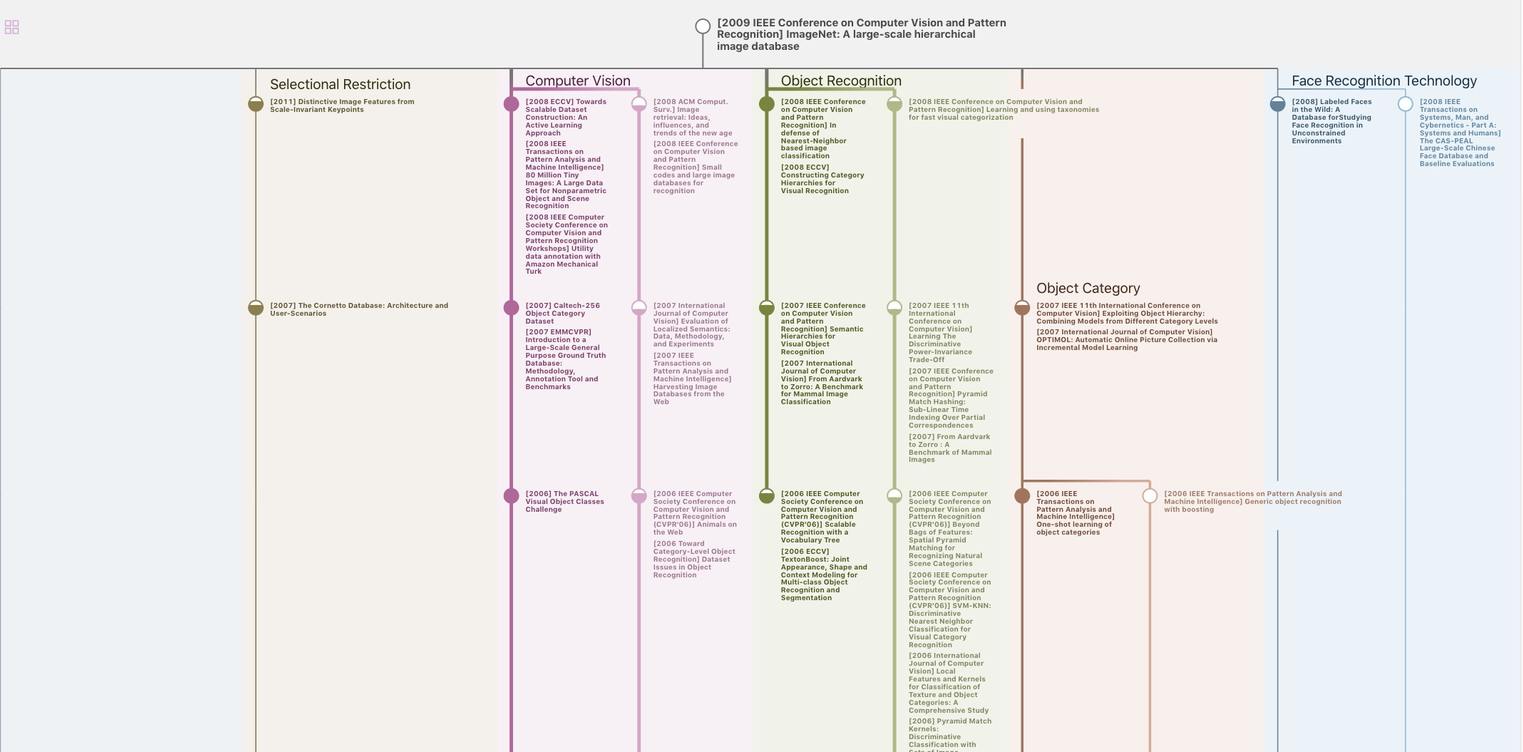
生成溯源树,研究论文发展脉络
Chat Paper
正在生成论文摘要