Deep Sparse Depth Completion Using Joint Depth and Normal Estimation.
ISCAS(2023)
摘要
Depth completion densifies sparse depth images obtained from LiDAR and is a great challenge due to the given extremely sparse information. In this paper, we propose deep sparse depth completion using joint depth and normal estimation. There exists a mutually convertible geometric relationship between depth and surface normal in 3D coordinate space. Based on the geometric relationship, we build a novel adversarial model that consists of one generator and two discriminators. We adopt an encoder-decoder structure for the generator. The encoder extracts features from RGB image, sparse depth image and its binary mask that represent the inherent geometric relationship between depth and surface normal, while two decoders with the same structure generate dense depth and surface normal based on the geometric relationship. We utilize two discriminators to generate guide information for sparse depth completion from the input RGB image while imposing an auxiliary geometric constraint for depth refinement. Experimental results on KITTI dataset show that the proposed method generates dense depth images with accurate object boundaries and outperforms state-of-the-art ones in terms of visual quality and quantitative measurements.
更多查看译文
关键词
Depth completion, adversarial learning, discriminator, generator, LiDAR, surface normal
AI 理解论文
溯源树
样例
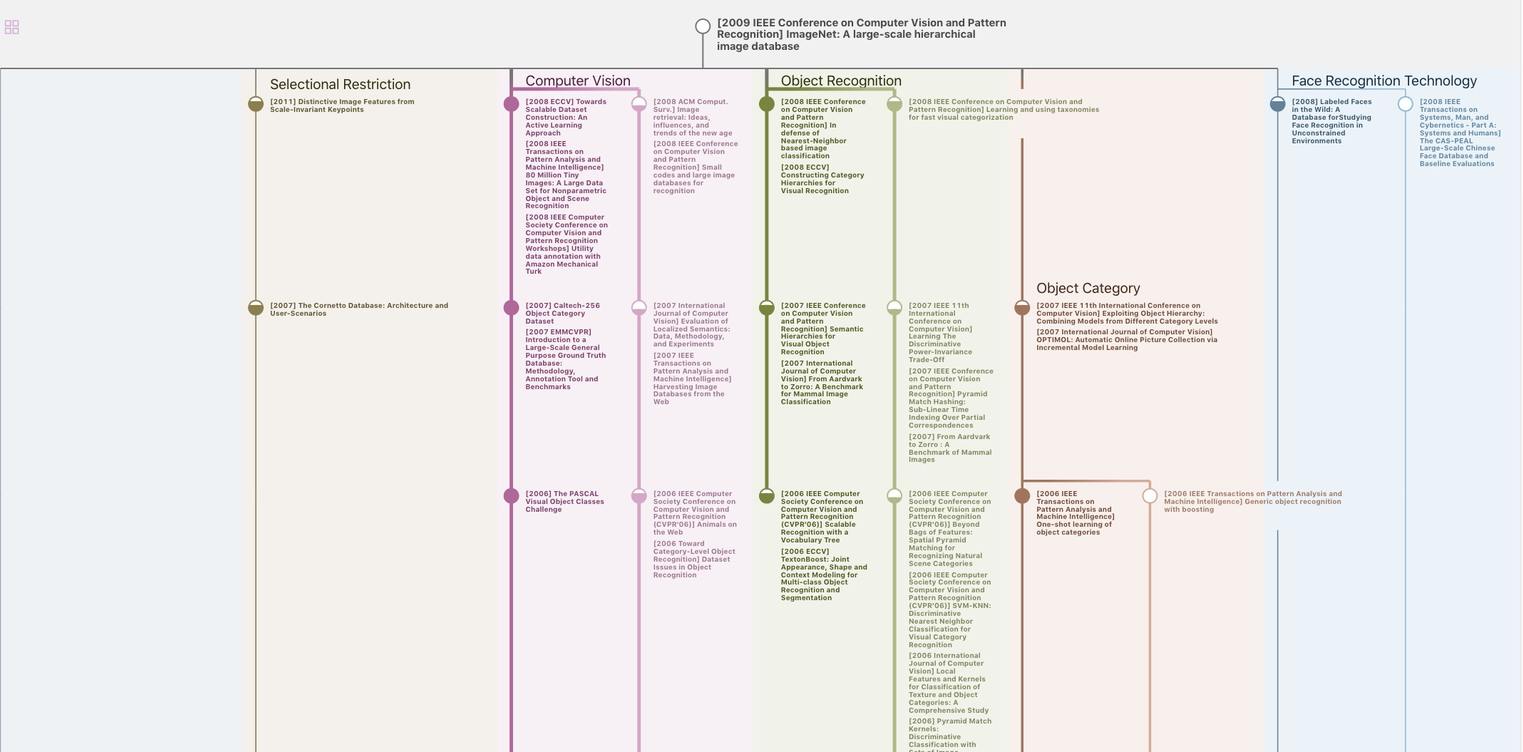
生成溯源树,研究论文发展脉络
Chat Paper
正在生成论文摘要