A Crowd-AI Collaborative Duo Relational Graph Learning Framework towards Social Impact Aware Photo Classification.
AAAI(2023)
摘要
In artificial intelligence (AI), negative social impact (NSI) represents the negative effect on the society as a result of mistakes conducted by AI agents. While the photo classification problem has been widely studied in the AI community, the NSI made by photo misclassification is largely ignored due to the lack of quantitative measurements of the NSI and effective approaches to reduce it. In this paper, we focus on an NSI-aware photo classification problem where the goal is to develop a novel crowd-AI collaborative learning framework that leverages online crowd workers to quantitatively estimate and effectively reduce the NSI of misclassified photos. Our problem is motivated by the limitations of current NSI-aware photo classification approaches that either 1) cannot accurately estimate NSI because they simply model NSI as the semantic difference between true and misclassified categories or 2) require costly human annotations to estimate NSI of pairwise class categories. To address such limitations, we develop SocialCrowd, a crowdsourcing-based NSI-aware photo classification framework that explicitly reduces the NSI of photo misclassification by designing a duo relational NSI-aware graph with the NSI estimated by online crowd workers. The evaluation results on two large-scale image datasets show that SocialCrowd not only reduces the NSI of photo misclassification but also improves the classification accuracy on both datasets.
更多查看译文
关键词
social,photo
AI 理解论文
溯源树
样例
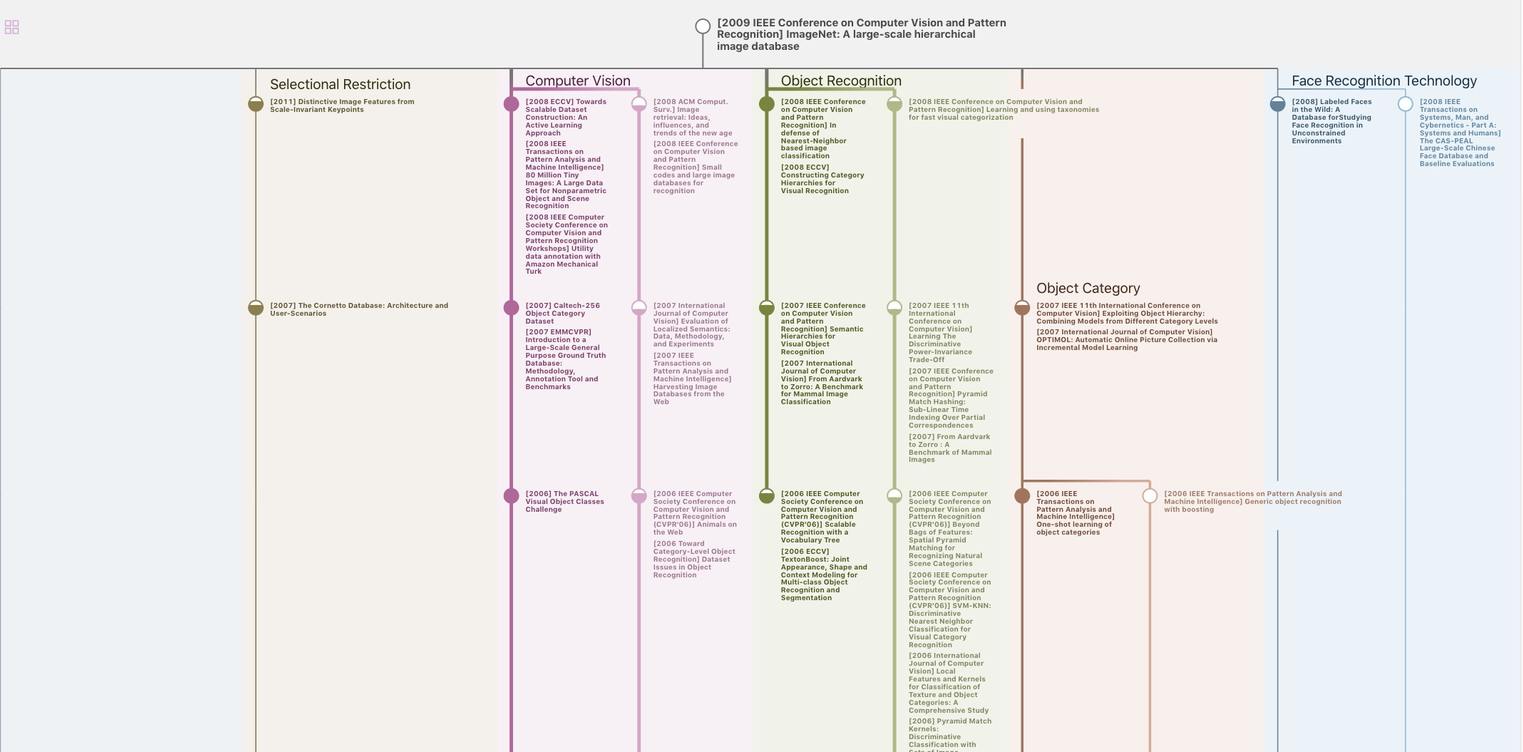
生成溯源树,研究论文发展脉络
Chat Paper
正在生成论文摘要