Metric Multi-View Graph Clustering.
AAAI(2023)
摘要
Graph-based methods have hitherto been used to pursue coherent patterns of data due to their ease of implementation and efficiency. These methods have been increasingly applied in multi-view learning and achieved promising performance in various clustering tasks. However, despite their noticeable empirical success, existing graph-based multi-view clustering methods may still suffer the suboptimal solution considering that multi-view data can be very complicated in raw feature space. Moreover, existing methods usually adopt the similarity metric by an ad hoc approach, which largely simplifies the relationship among real-world data and results in an inaccurate output. To address these issues, we propose to seamlessly integrate metric learning and graph learning for multiview clustering. Specifically, we employ a useful metric to depict the inherent structure with linearity-aware of affinity graph representation learned based on the self-expressiveness property. Furthermore, instead of directly utilizing the raw features, we prefer to recover a smooth representation such that the geometric structure of the original data can be retained. We model the above concerns into a unified learning framework, and hence complement each learning subtask in a mutual reinforcement manner. The empirical studies corroborate our theoretical findings and demonstrate that the proposed method is able to boost the multi-view clustering performance.
更多查看译文
关键词
graph,multi-view
AI 理解论文
溯源树
样例
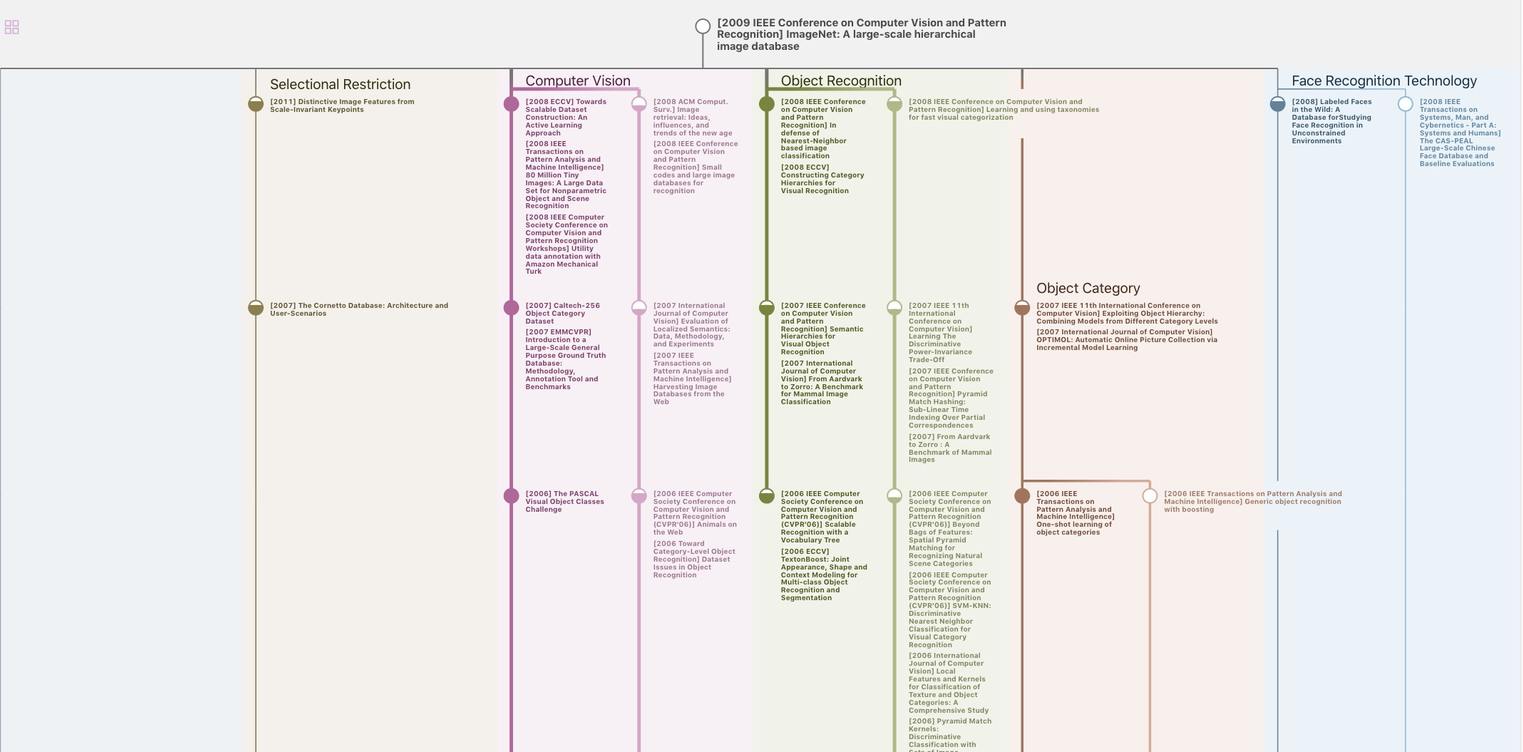
生成溯源树,研究论文发展脉络
Chat Paper
正在生成论文摘要