Adversarial Dense Contrastive Learning for Semi-supervised Semantic Segmentation.
IEEE transactions on image processing : a publication of the IEEE Signal Processing Society(2023)
摘要
Semi-supervised dense prediction tasks, such as semantic segmentation, can be greatly improved through the use of contrastive learning. However, this approach presents two key challenges: selecting informative negative samples from a highly redundant pool and implementing effective data augmentation. To address these challenges, we present an adversarial contrastive learning method specifically for semi-supervised semantic segmentation. Direct learning of adversarial negatives is adopted to retain discriminative information from the past, leading to higher learning efficiency. Our approach also leverages an advanced data augmentation strategy called AdverseMix, which combines information from under-performing classes to generate more diverse and challenging samples. Additionally, we use auxiliary labels and classifiers to prevent over-adversarial negatives from affecting the learning process. Our experiments on the Pascal VOC and Cityscapes datasets demonstrate that our method outperforms the state-of-the-art by a significant margin, even when using a small fraction of labeled data.
更多查看译文
关键词
Semi-supervised learning,semantic segmentation,adversarial contrastive learning,data augmentation
AI 理解论文
溯源树
样例
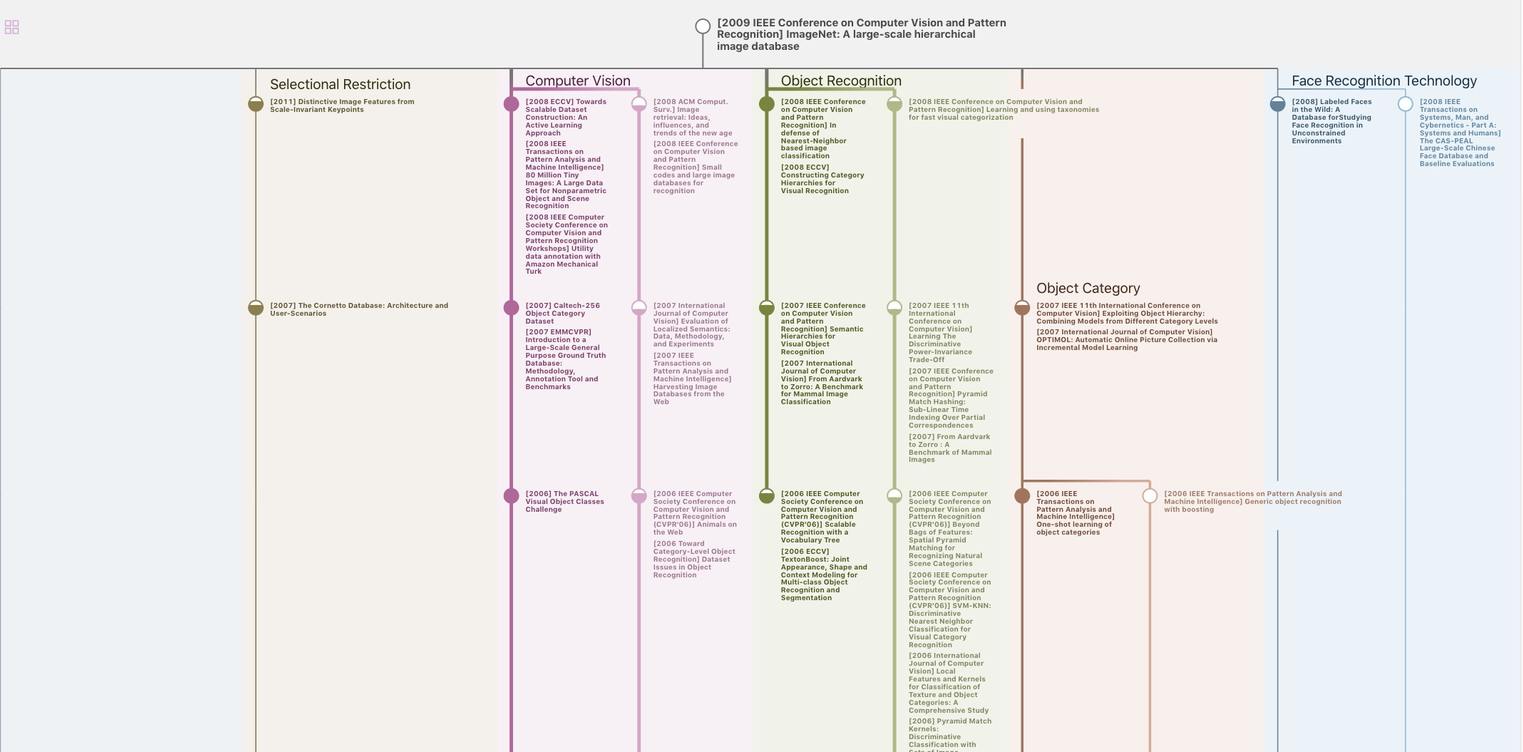
生成溯源树,研究论文发展脉络
Chat Paper
正在生成论文摘要