Leveraging Few-Shot Data Augmentation and Waterfall Prompting for Response Generation
CoRR(2023)
Abstract
This paper discusses our approaches for task-oriented conversational modelling using subjective knowledge, with a particular emphasis on response generation. Our methodology was shaped by an extensive data analysis that evaluated key factors such as response length, sentiment, and dialogue acts present in the provided dataset. We used few-shot learning to augment the data with newly generated subjective knowledge items and present three approaches for DSTC11: (1) task-specific model exploration, (2) incorporation of the most frequent question into all generated responses, and (3) a waterfall prompting technique using a combination of both GPT-3 and ChatGPT.
MoreTranslated text
Key words
waterfall prompting,augmentation,response,data,few-shot
AI Read Science
Must-Reading Tree
Example
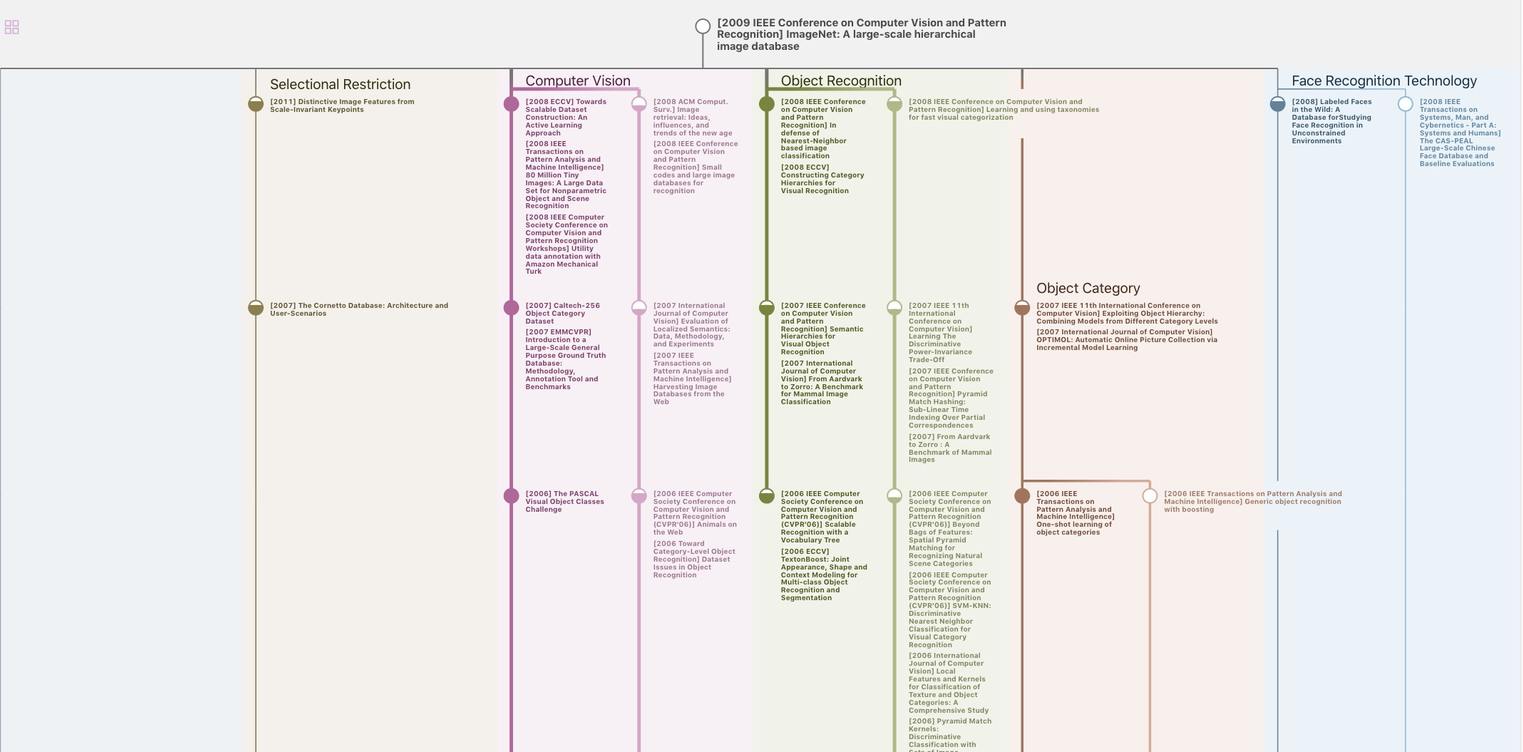
Generate MRT to find the research sequence of this paper
Chat Paper
Summary is being generated by the instructions you defined