Neural-network-encoded variational quantum algorithms
PHYSICAL REVIEW APPLIED(2024)
摘要
We introduce a general framework called neural -network- (NN) encoded variational quantum algorithms (VQAs), or NNVQA for short, to address the challenges of implementing VQAs on noisy intermediate -scale quantum (NISQ) computers. Specifically, NNVQA feeds input (such as parameters of a Hamiltonian) from a given problem to a neural network and uses its outputs to parameterize an ansatz circuit for the standard VQA. Combining the strengths of NN and parameterized quantum circuits, NNVQA can accelerate the training process of VQAs and handle a broad family of related problems with varying input parameters with the pretrained NN. To concretely illustrate the merits of NNVQA, we present results on a NN variational quantum eigensolver (VQE) for solving the ground state of parameterized XXZ spin models in one and two dimensions. Our results demonstrate that NNVQE is able to estimate the ground -state energies of parameterized Hamiltonians with high precision without fine tuning, and significantly reduce the overall training cost to estimate ground -state properties across the phases of the given Hamiltonian. We also employ an active learning strategy to further increase the training efficiency while maintaining prediction accuracy. These encouraging results demonstrate that NNVQAs offer an alternative hybrid quantum -classical paradigm to utilize NISQ resources for solving more realistic and challenging computational problems.
更多查看译文
AI 理解论文
溯源树
样例
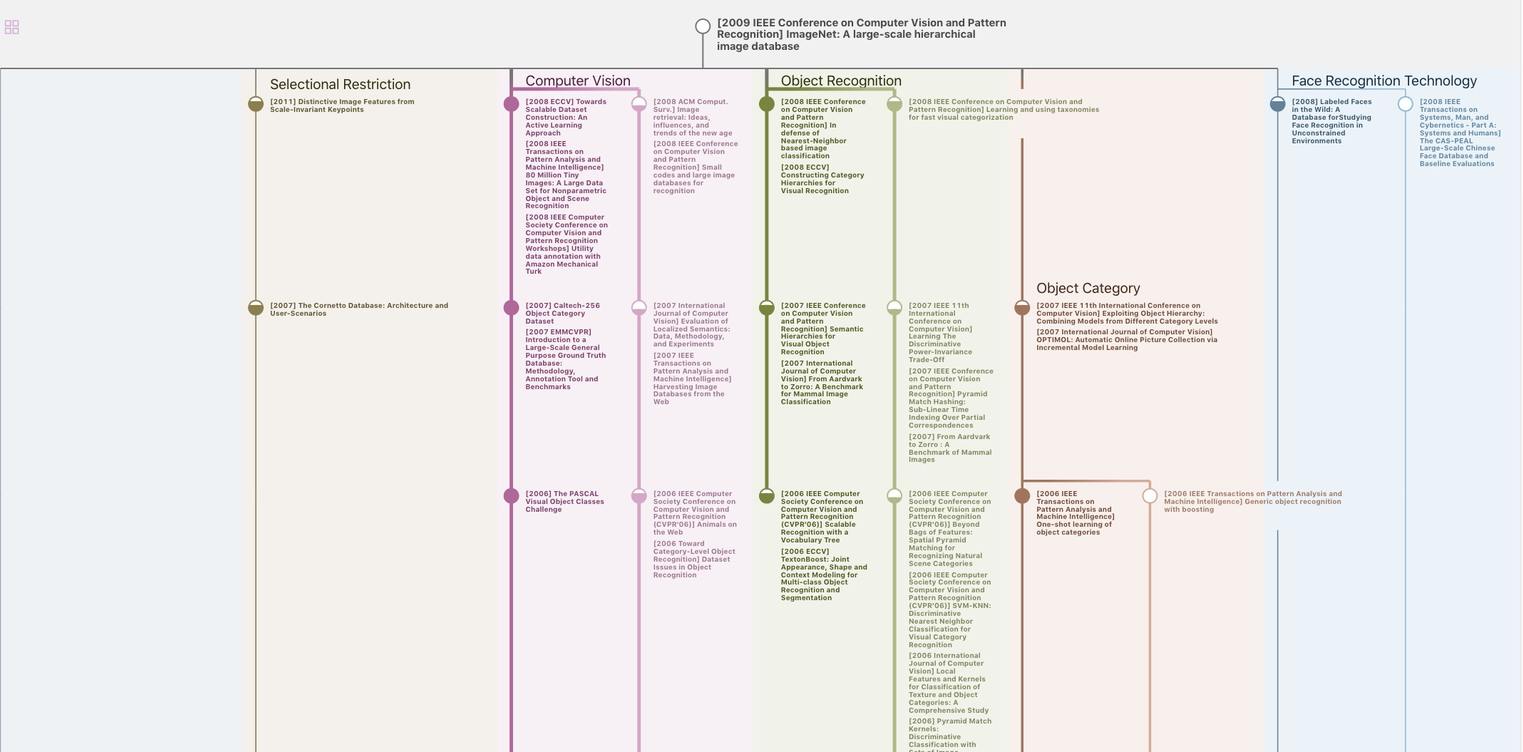
生成溯源树,研究论文发展脉络
Chat Paper
正在生成论文摘要