Deep neural networks predict class I major histocompatibility complex epitope presentation and transfer learn neoepitope immunogenicity
Nature Machine Intelligence(2023)
摘要
Identifying neoepitopes that elicit an adaptive immune response is a major bottleneck to developing personalized cancer vaccines. Experimental validation of candidate neoepitopes is extremely resource intensive and the vast majority of candidates are non-immunogenic, creating a needle-in-a-haystack problem. Here we address this challenge, presenting computational methods for predicting class I major histocompatibility complex (MHC-I) epitopes and identifying immunogenic neoepitopes with improved precision. The BigMHC method comprises an ensemble of seven pan-allelic deep neural networks trained on peptide–MHC eluted ligand data from mass spectrometry assays and transfer learned on data from assays of antigen-specific immune response. Compared with four state-of-the-art classifiers, BigMHC significantly improves the prediction of epitope presentation on a test set of 45,409 MHC ligands among 900,592 random negatives (area under the receiver operating characteristic = 0.9733; area under the precision-recall curve = 0.8779). After transfer learning on immunogenicity data, BigMHC yields significantly higher precision than seven state-of-the-art models in identifying immunogenic neoepitopes, making BigMHC effective in clinical settings.
更多查看译文
关键词
Machine learning,Proteome informatics,Engineering,general
AI 理解论文
溯源树
样例
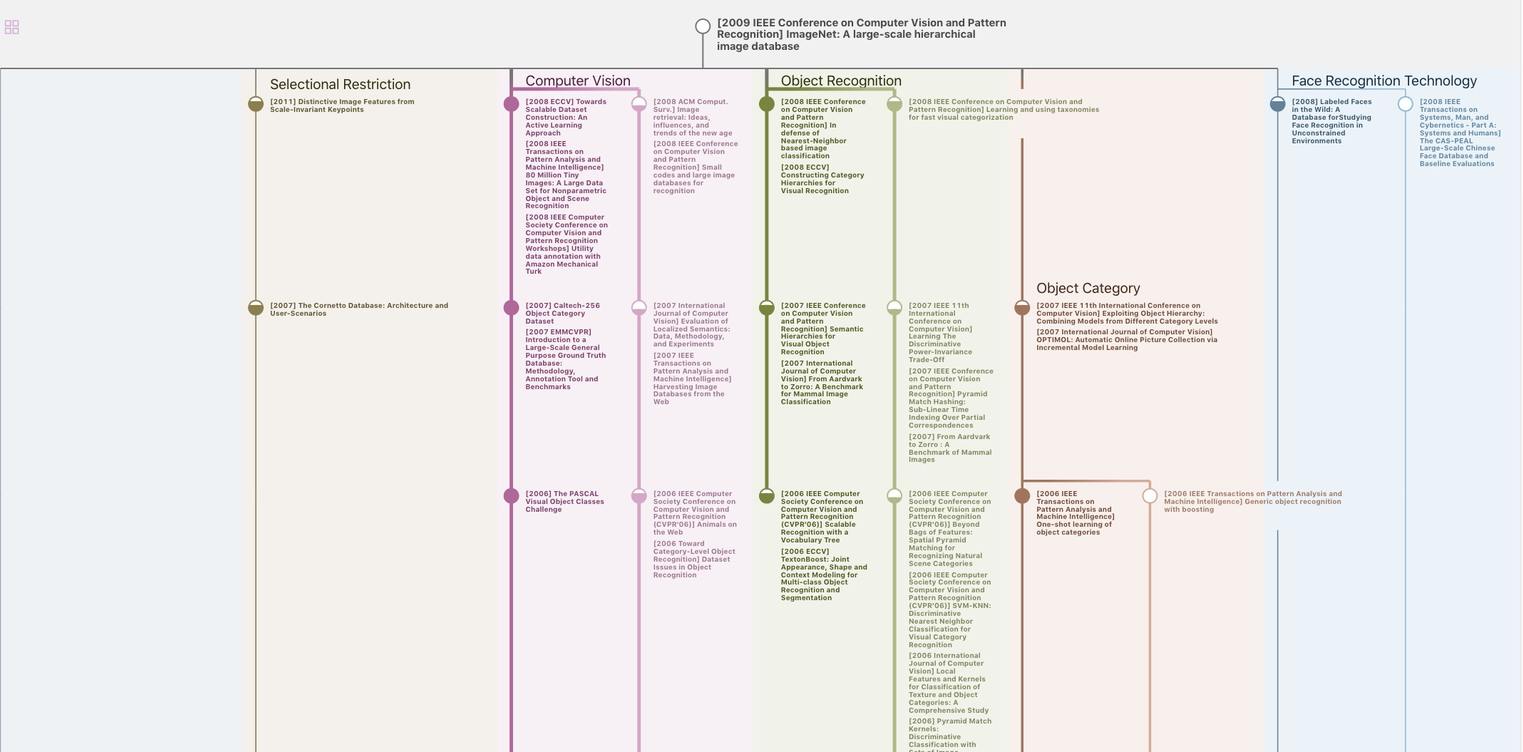
生成溯源树,研究论文发展脉络
Chat Paper
正在生成论文摘要