Deep Learning Ultrasound Computed Tomography under Sparse Sampling.
IEEE transactions on ultrasonics, ferroelectrics, and frequency control(2023)
摘要
Ultrasound computed tomography (USCT) is a fast-emerging imaging modality that is expected to help detect and characterize breast tumors by quantifying the distribution of the speed of sound (SOS) and acoustic attenuation in breast tissue. High-quality quantitative SOS reconstruction in USCT requires a large number of transducers, which incurs high system costs and slow computation. In contrast, sparsely distributed arrays are low-cost and fast but significantly degrade image quality. Thus, we propose a framework to achieve high-quality SOS Reconstruction under Sparse Sampling based on a convolutional neural Network (SRSS-Net) with faster computation. We first apply the bent-ray algorithm to sparsely sampled data and then apply the SRSS-Net to efficiently improve the image quality. Experimental results on synthetic and real datasets demonstrate that the proposed SRSS-Net provides reconstructions that are superior to those of state-of-the-art methods in terms of artifact suppression, structural preservation, quantitative restoration, and computational speed. As demonstrated in our experiments, the fine-tuning training strategy is suggested when applying SRSS-Net to real-world circumstances. The imaging and computational performance of SRSS-Net on the inhomogeneous breast phantom further demonstrates that SRSS-Net has great potential in real-time breast cancer detection.
更多查看译文
关键词
Deep learning,image reconstruction,sparse view,speed of sound (SOS),ultrasound computed tomography (USCT)
AI 理解论文
溯源树
样例
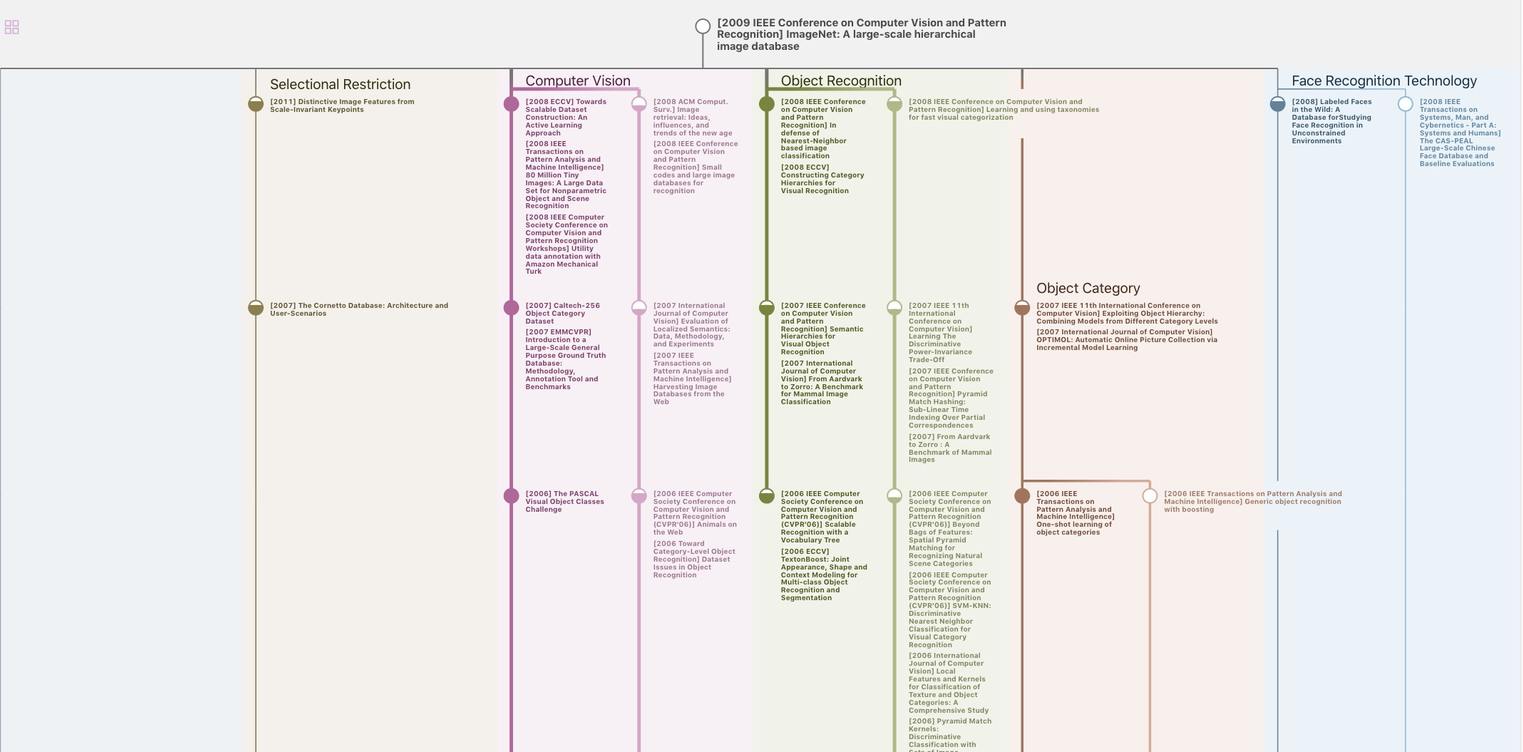
生成溯源树,研究论文发展脉络
Chat Paper
正在生成论文摘要