Improving Recommender System Diversity with Variational Autoencoders.
BIAS(2023)
摘要
Focusing only on relevance when training recommender systems can lead to suboptimal recommendations. On eCommerce platforms, this not only leads to poor user experience for customers but also limits opportunities for vendors due to popularity bias. Consequently, there is an increasing interest in systems that produce recommendations that are not only relevant but also diverse. In this work, we have shown how diversity can be measured and improved in the context of a large eCommerce recommendation platform. We first evaluate how diversity should be measured feasibly in real-world recommender systems and introduce TILD: a novel diversity metric that measures representational diversity across user sessions. Second, we introduce VAE-based Generation of User Profiles (VAE-GUP), wherein we use the generative nature of VAEs to learn a user profile distribution and sample multiple user profiles. In a simple post-processing step, we combine the recommendations generated by these profiles to generate a final list of more diverse recommendations. Through empirical analysis of benchmark and real-world datasets, we show that VAE-GUP can improve several diversity metrics without significantly compromising relevance. This research has been done in collaboration with Bol.com, the largest e-commerce retailer in the Benelux region.
更多查看译文
关键词
recommender system diversity
AI 理解论文
溯源树
样例
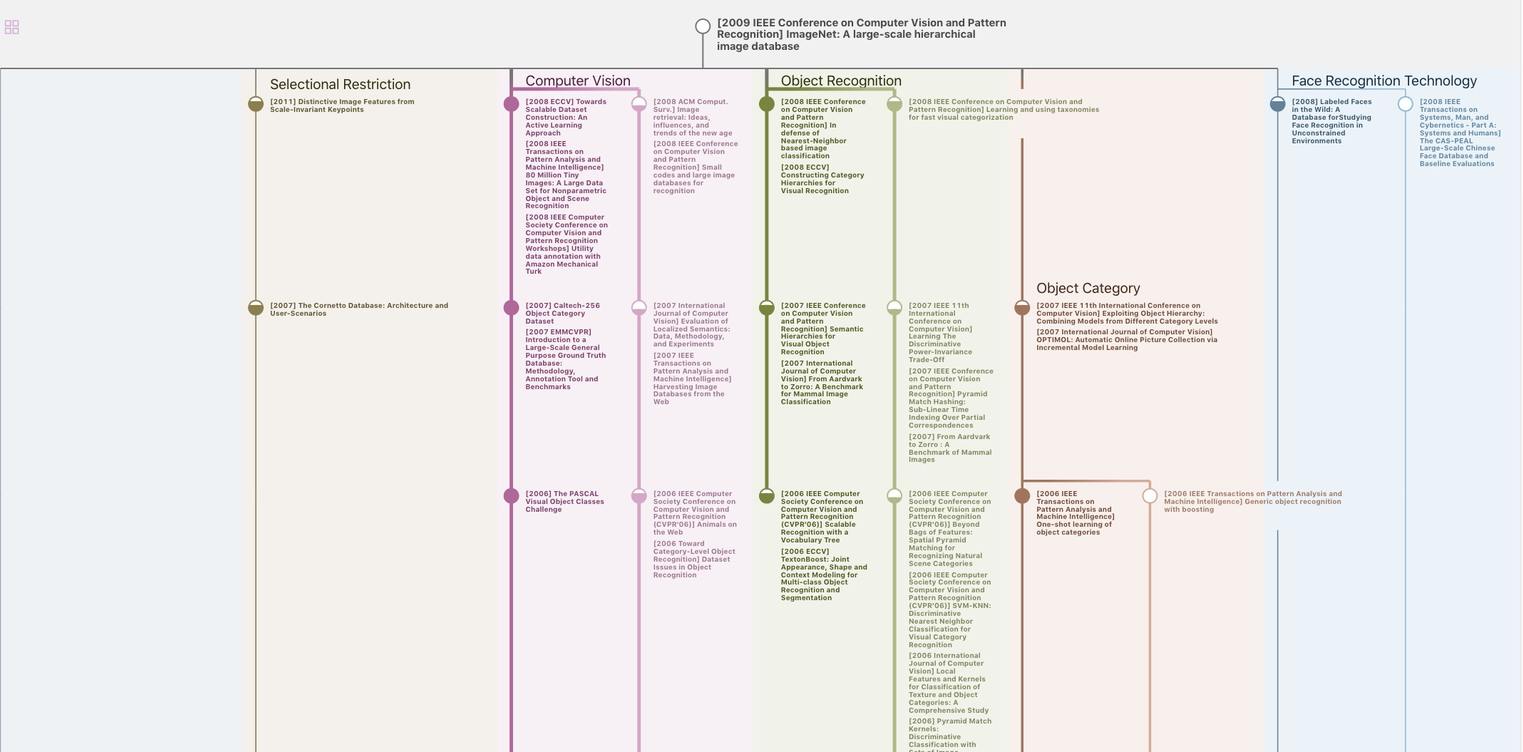
生成溯源树,研究论文发展脉络
Chat Paper
正在生成论文摘要