Benchmarking of Anomaly Detection Techniques in O-RAN for Handover Optimization.
IWCMC(2023)
摘要
With today's proliferation of IoT and real-time applications, it has become crucial to properly handle traffic, optimize the quality-of-service (QoS) of wireless networks and design novel approaches to enable reliable communications with bounded latency and high throughput for future wireless services. In order to meet these stringent QoS requirements, there has been a recent surge in research that investigates a deep restructuring of the Radio Access Network (RAN). In particular, the open radio access network (O-RAN) framework promises to deliver flexible, scalable, and agile solutions for improving the handover process of a moving user equipment (UE), by considering factors related to the requirements of applications in terms of QoS, traffic load, signal quality and the diversity of multiple access technologies or radio frequencies of the environment. Building on this basis, this paper investigates the handover process of a moving vehicle, by exploring and comparing the prediction accuracy results of different machine learning (ML) techniques used for anomaly detection. In particular, the structure of one of the O-RAN modules is presented. This module relates to a traffic steering application, specifically designed and used to detect anomalies within the network. Several ML techniques are then implemented in the O-RAN traffic steering module to predict the handover. The results pertaining to the comparison of the implemented ML techniques show that the random forest algorithm gives the highest accuracy (up to 98%), which helps boosting the handover process.
更多查看译文
关键词
5G, Anomaly Detection, Handover, Artificial Intelligence, Machine Learning, Open RAN, RIC, xApp
AI 理解论文
溯源树
样例
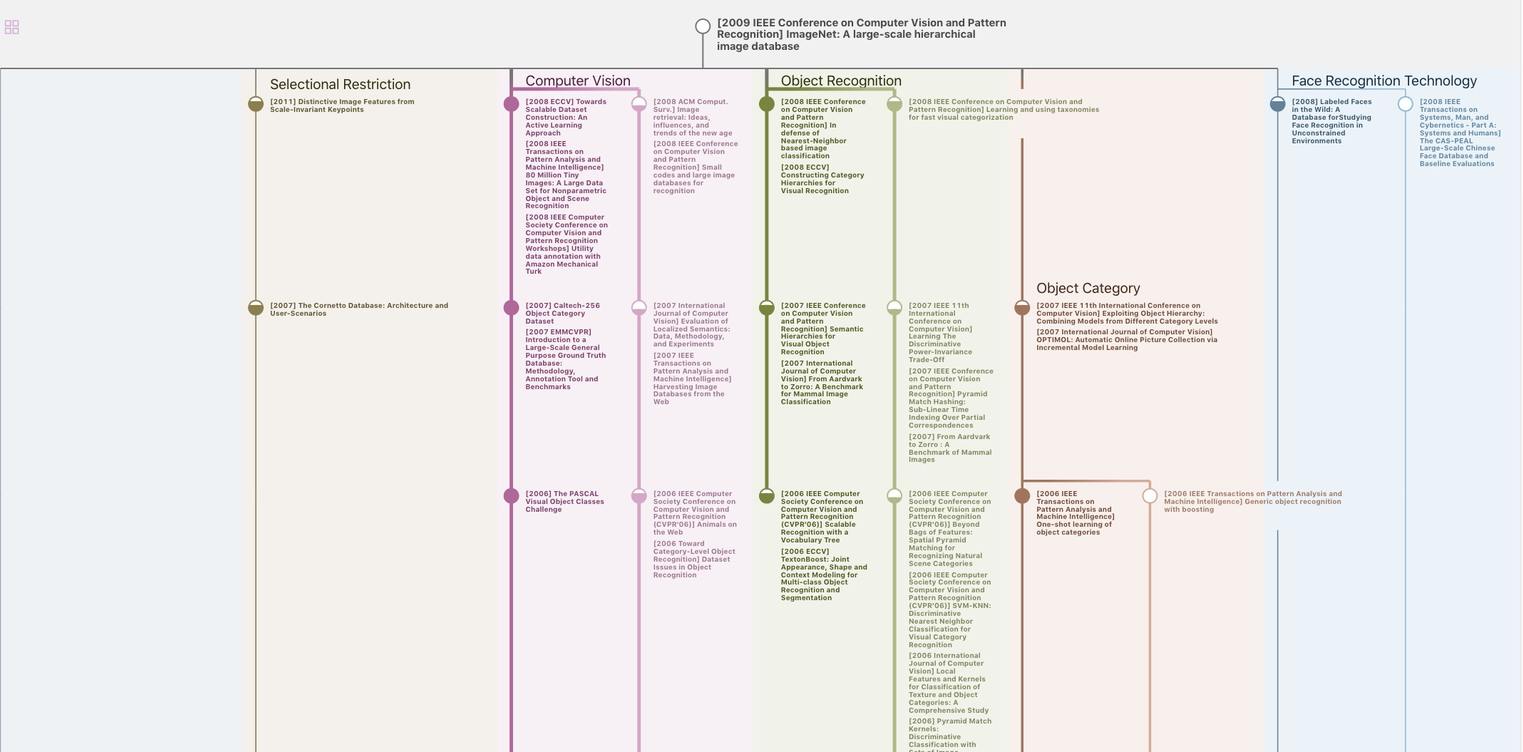
生成溯源树,研究论文发展脉络
Chat Paper
正在生成论文摘要