WPT-enabled Multi-UAV Path Planning for Disaster Management Deep Q-Network
IWCMC(2023)
摘要
Unmanned aerial vehicles (UAVs) have been more prevalent over the past several years with the intent to be widely deployed in many industries, including agriculture, cinematography, healthcare, delivery, and disaster management missions due to their ability to provide real-time situational awareness. However, various limitations such as the battery capacity, the charging method, and the flying range make it difficult for most applications to carry out routine tasks in vast areas. In this paper, a deep reinforcement learning (DRL) method for multi-UAV path planning that considers a cooperative action amongst UAVs in which they share the next destination to avoid visiting the same location at the same time. The Deep Q-Network algorithm (DQN) enables UAVs to autonomously plan their fastest path and ensure the continuity of the mission by deciding when to schedule a visit to a charging station or a data collection point. An objective function with a tailored reward is designed to maintain the stability of the model and ensure the quick convergence of the model. Lastly, the proposed strategy has been demonstrated by the experiments on different scenarios and showed its effectiveness in ensuring the continuity of the mission with a fastest path possible.
更多查看译文
关键词
unmanned aerial vehicles,wireless power transfer,deep reinforcement learning,wireless sensor networks,aerial data collection,smart health
AI 理解论文
溯源树
样例
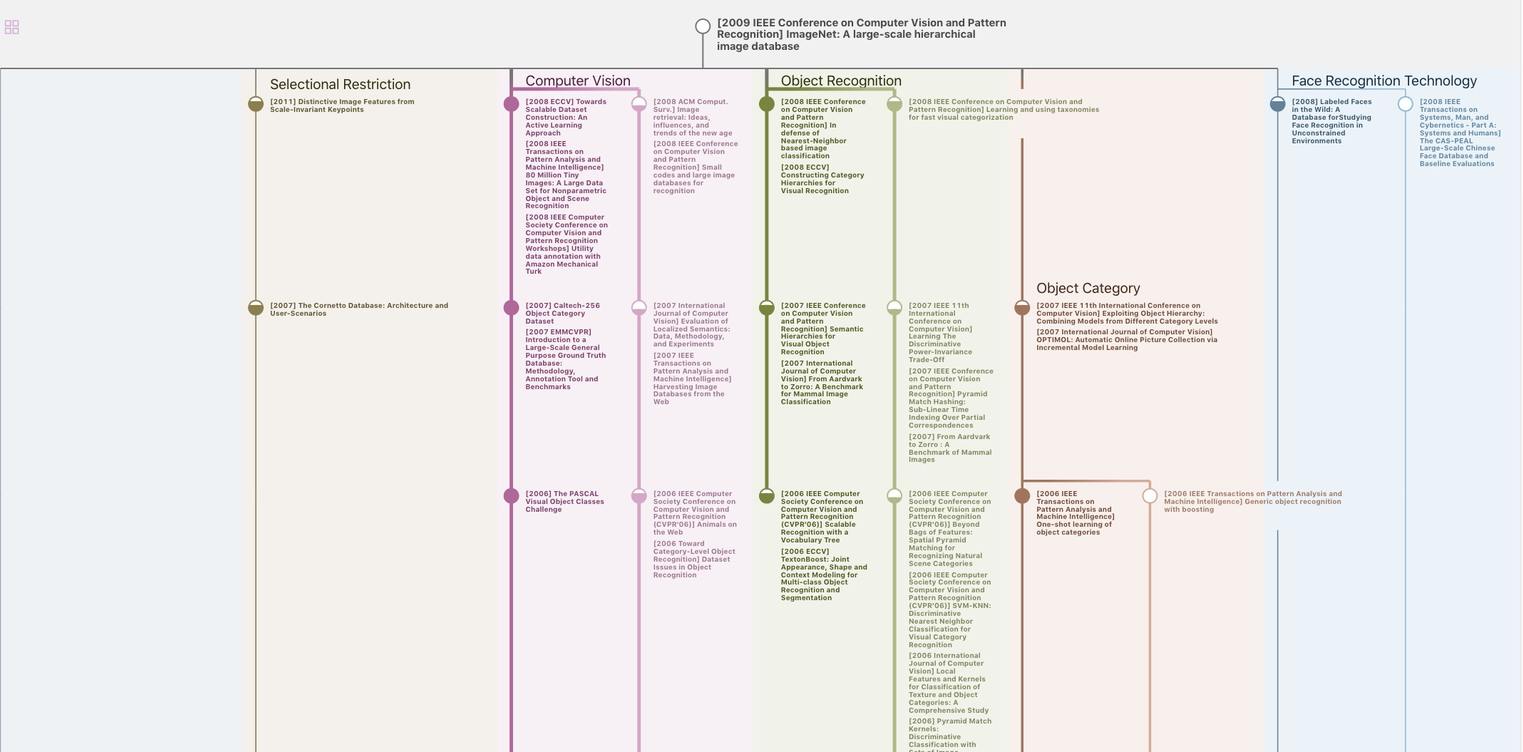
生成溯源树,研究论文发展脉络
Chat Paper
正在生成论文摘要