TinyML Custom AI Algorithms for Low-Power IoT Data Compression: A Bridge Monitoring Case Study.
MetroInd4.0&IoT(2023)
摘要
The use of low-power IoT devices presents challenges for transmitting large amounts of data, particularly in remote and infrastructure-lacking environments, as is often the case in monitoring the structural health of bridges. The low transmission rates and limited payloads of protocols like LoRaWAN exacerbate these challenges, making data compression techniques essential for efficient data transmission. This study evaluates the performance of TinyML custom AI algorithms designed for low-power IoT devices as a solution for data compression. We present a case study using real-world bridge monitoring data from the Queen’s Park Bridge in Chester. We compare the performance of TinyML custom AI algorithms with other data compression algorithm, evaluating their compression ratios, error metrics, and processing efficiency. Our results indicate that TinyML custom AI algorithms offer a promising solution for efficient data transmission in low-power IoT devices, particularly in infrastructure-lacking environments. These findings highlight significant opportunities for further research and development of custom AI algorithms for data compression in various IoT scenarios, emphasizing the importance of this study for optimizing data transmission in low-power IoT devices.
更多查看译文
关键词
Internet of Things,data compression,TinyML,custom AI algorithms,Low-power devices,bridge monitoring
AI 理解论文
溯源树
样例
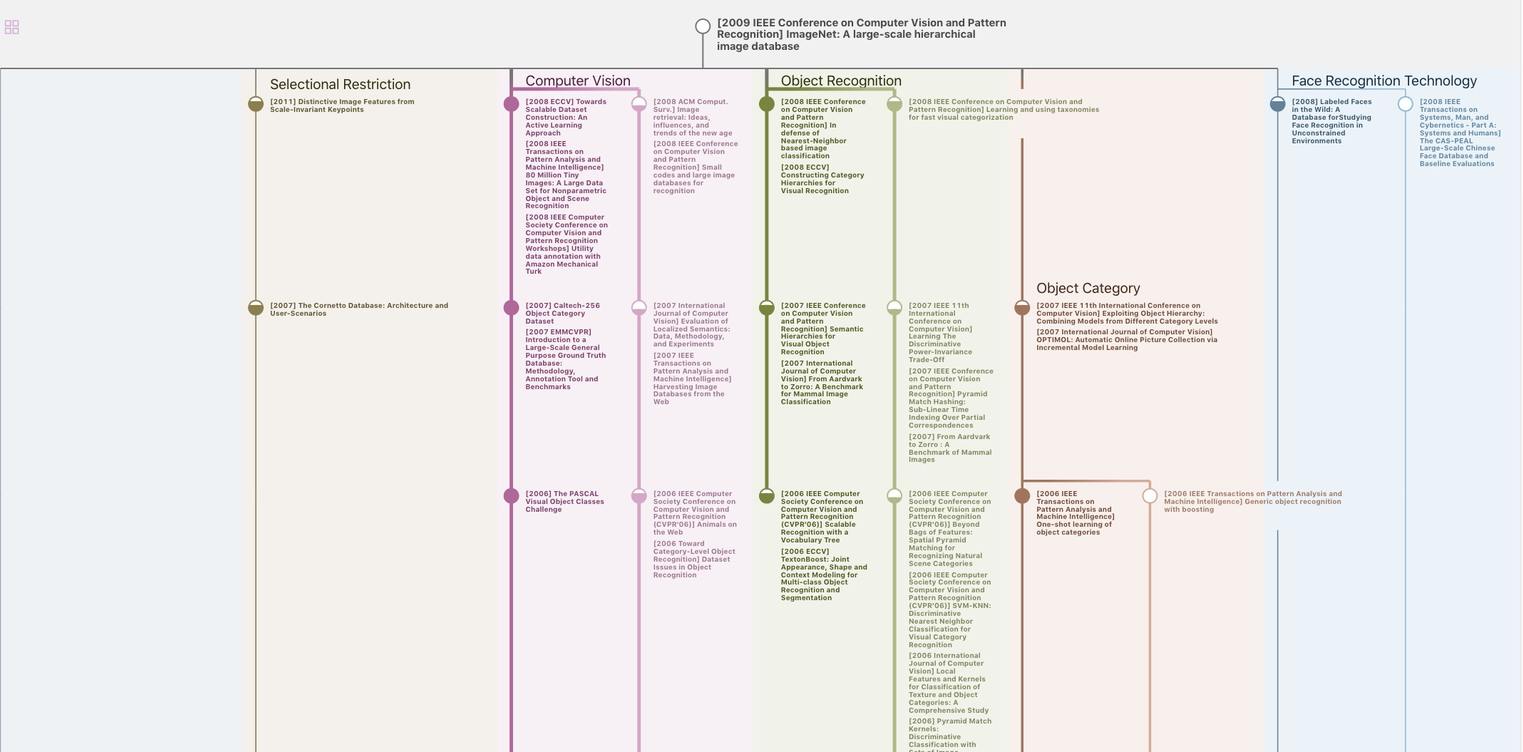
生成溯源树,研究论文发展脉络
Chat Paper
正在生成论文摘要