Biological insights on grammar-structured mutations improve fitness and diversity
GECCO(2023)
摘要
Grammar-Guided Genetic Programming (GGGP) employs a variety of concepts from evolutionary theory to autonomously design solutions for a given task. Recent insights from evolutionary biology can lead to further improvements in GGGP algorithms. In this paper, we propose a new mutation approach called Facilitated Mutation (FM) that is based on the theory of Facilitated Variation. We evaluate the performance of FM on the evolution of neural network optimizers for image classification, a relevant task in Evolutionary Computation, with important implications for the field of Machine Learning. We compare FM and FM combined with crossover (FMX) against a typical mutation approach to assess the benefits of the approach. We find that FMX provides statistical improvements in key metrics, creating a superior optimizer overall (+0.5% average test accuracy), improving the average quality of solutions (+53% average population fitness), and discovering more diverse high-quality behaviors (+523 high-quality solutions discovered on average). Additionally, FM and FMX reduce the number of fitness evaluations in an evolutionary run, reducing computational costs. FM's implementation cost is minimal and the approach is theoretically applicable to any algorithm where genes are associated with a grammar non-terminal, making this approach applicable in many existing GGGP systems.
更多查看译文
关键词
Grammar-guided Genetic Programming,Mutation,Optimizers
AI 理解论文
溯源树
样例
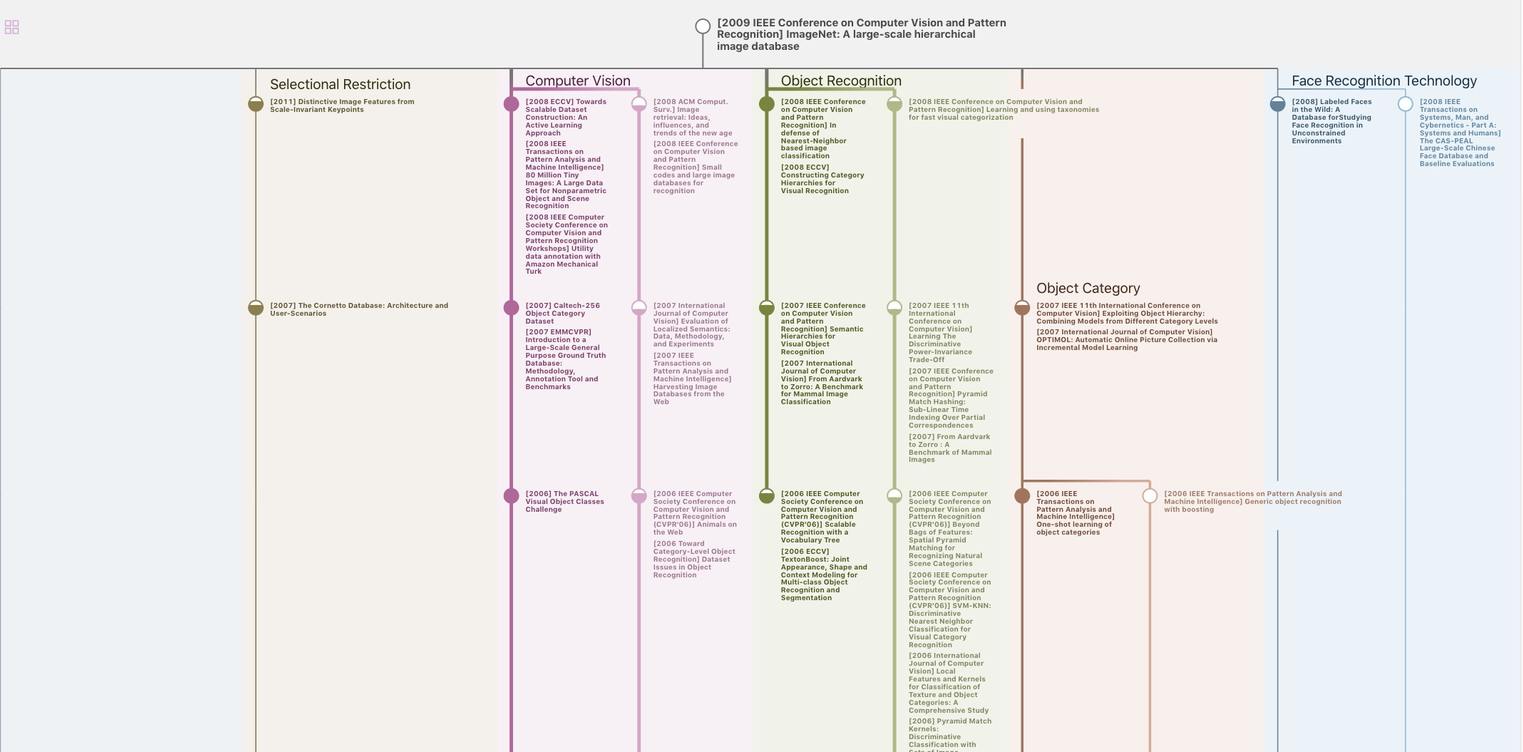
生成溯源树,研究论文发展脉络
Chat Paper
正在生成论文摘要