Replacing the FitzHugh-Nagumo Electrophysiology Model by Physics-Informed Neural Networks.
ICCS (2)(2023)
摘要
This paper presents a novel approach to replace the FitzHugh-Nagumo (FHN) model with physics-informed neural networks (PINNs). The FHN model, a system of two ordinary differential equations, is widely used in electrophysiology and neurophysiology to simulate cell action potentials. However, in tasks such as whole-organ electrophysiology modeling and drug testing, the numerical solution of millions of cell models is computationally expensive. To address this, we propose using PINNs to accurately approximate the two variables of the FHN model at any time instant, any initial condition, and a wide range of parameters. In particular, this eliminates the need for causality after training. We employed time window marching and increased point cloud density on transition regions to improve the training of the neural network due to nonlinearity, sharp transitions, unstable equilibrium, and bifurcations of parameters. The PINNs were generated using NVIDIA’s Modulus framework, allowing efficient deployment on modern GPUs. Our results show that the generated PINNs could reproduce FHN solutions with average numerical errors below 0.5%, making them a promising lightweight computational model for electrophysiology and neurophysiology research.
更多查看译文
关键词
electrophysiology,neural networks,model,fitzhugh-nagumo,physics-informed
AI 理解论文
溯源树
样例
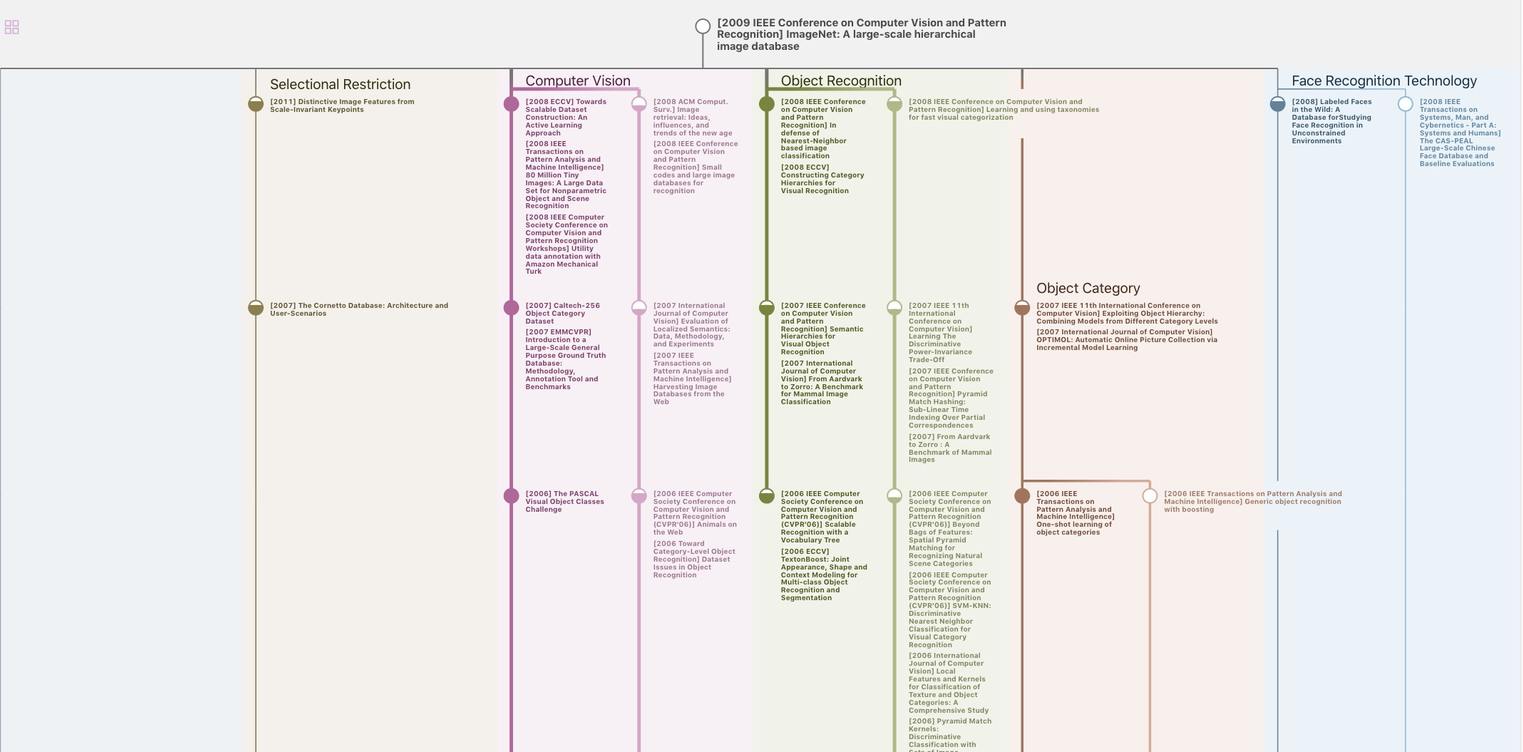
生成溯源树,研究论文发展脉络
Chat Paper
正在生成论文摘要