CNN-Based Quantification of Blood Vessels Lumen in 3D Images.
ICCS (2)(2023)
摘要
The aim of this work is to develop a method for automated, fast and accurate geometric modeling of blood vessels from 3D images, robust to image limited resolution, noise and artefacts. Within the centerline-radius paradigm, convolutional neural networks (CNNs) are used to approximate the mapping from the image cross-sections to vessel lumen parameters. A six-parameter image formation model is utilized to derive conditions for this mapping to exist, and to generate images for the CNN training, validation and testing. The trained networks are applied to real-life time-of-flight (TOF) magnetic resonance images (MRI) of a blood-flow phantom. Excellent agreement is observed between the predictions made by the CNN and those obtained via model fitting as the reference method. The latter is a few orders of magnitude slower than the CNN and suffers from local minima problem. The CNN is also trained and tested on publicly available contrast-enhanced (CE) computed tomography angiography (CTA) clinical datasets. It accurately predicts the coronary-tree lumen parameters in seconds, compared to hours needed by human experts. The method can be an aid to vascular diagnosis and automated annotation of images.
更多查看译文
关键词
blood vessels lumen,cnn-based
AI 理解论文
溯源树
样例
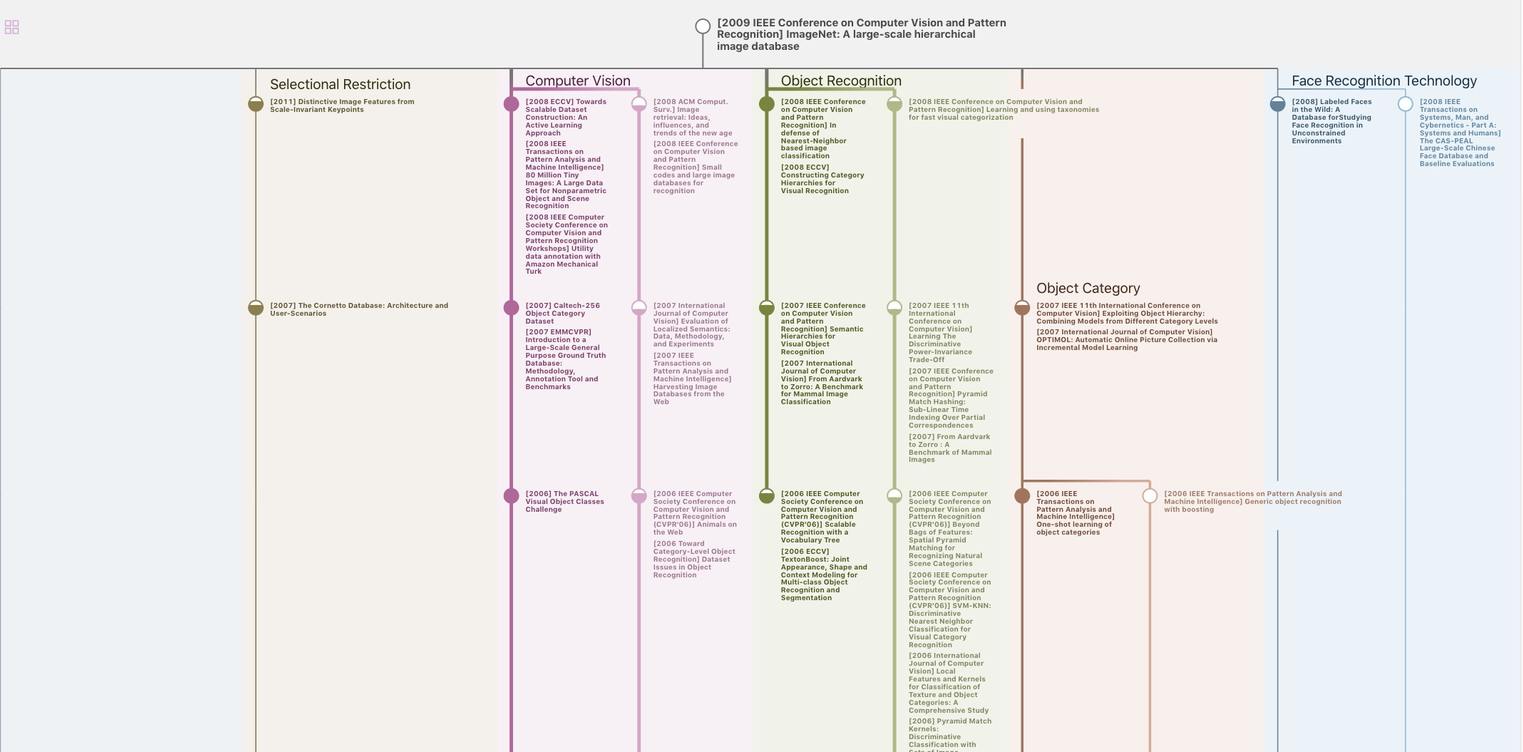
生成溯源树,研究论文发展脉络
Chat Paper
正在生成论文摘要