Few-shot Malicious Domain Detection on Heterogeneous Graph with Meta-learning.
CSCWD(2023)
摘要
The Domain Name System (DNS), one of the essential basic services on the Internet, is often abused by attackers to launch various cyber attacks, such as phishing and spamming. Researchers have proposed many machine learning-based and deep learning-based methods to detect malicious domains. However, these methods rely on a large-scale dataset with labeled samples for model training. The fact is that the labeled domain samples are limited in the real-world DNS dataset. In this paper, we propose a few-shot malicious domain detection model named MetaDom, which employs a meta-learning algorithm for model optimization. Specifically, We first model the DNS scenario as a heterogeneous graph to capture richer information by analysing the complex relations among domains, IP addresses and clients. Then, we learn the domain representations with a heterogeneous graph neural network on the DNS HG. Finally, considering that only few labeled data are available in the real-world DNS scenario, a meta-learning algorithm with knowledge distillation is introduced to optimize the model. Extensive experiments on the real DNS dataset show that MetaDom outperforms other state-of-the-art methods.
更多查看译文
关键词
malicious domain detection,heterogeneous graph neural networks,few-shot learning,meta-learning
AI 理解论文
溯源树
样例
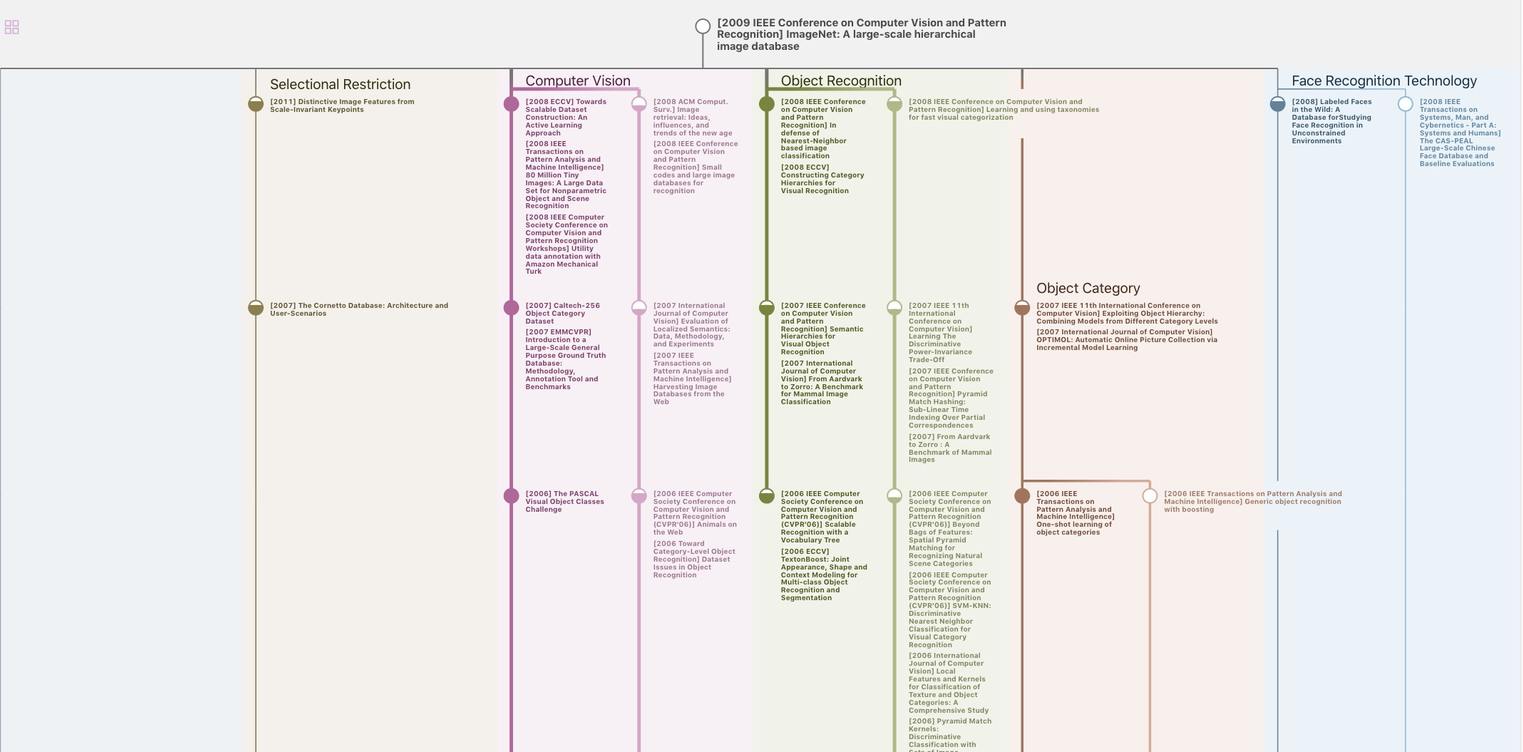
生成溯源树,研究论文发展脉络
Chat Paper
正在生成论文摘要