Dream to Generalize: Zero-Shot Model-Based Reinforcement Learning for Unseen Visual Distractions.
AAAI(2023)
摘要
Model-based reinforcement learning (MBRL) has been used to efficiently solve vision-based control tasks in high-dimensional image observations. Although recent MBRL algorithms perform well in trained observations, they fail when faced with visual distractions in observations. These task-irrelevant distractions (e.g., clouds, shadows, and light) may be constantly present in real-world scenarios. In this study, we propose a novel self-supervised method, D ream to G eneralize ( Dr. G ), for zero-shot MBRL. Dr. G trains its encoder and world model with dual contrastive learning which efficiently captures task-relevant features among multi-view data augmentations. We also introduce a recurrent state inverse dynamics model that helps the world model to better understand the temporal structure. The proposed methods can enhance the robustness of the world model against visual distractions. To evaluate the generalization performance, we first train Dr. G on simple backgrounds and then test it on complex natural video backgrounds in the DeepMind Control suite, and the randomizing environments in Robosuite. Dr. G yields a performance improvement of 117% and 14% over prior works, respectively. Our code is open-sourced and available.
更多查看译文
关键词
unseen visual distractions,learning,zero-shot,model-based
AI 理解论文
溯源树
样例
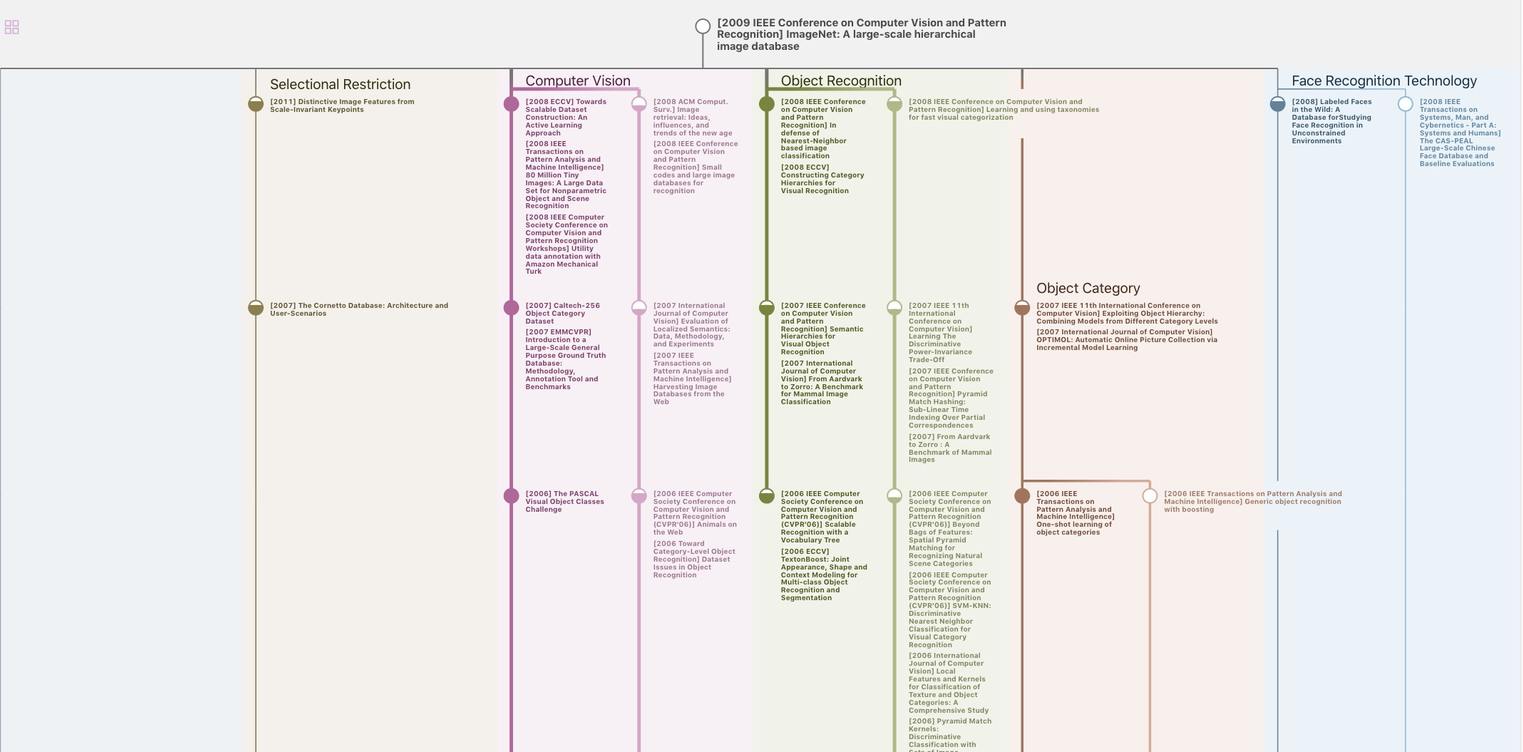
生成溯源树,研究论文发展脉络
Chat Paper
正在生成论文摘要