Feature-aware conditional GAN for category text generation.
Neurocomputing(2023)
摘要
Category text generation receives considerable attentions since it is beneficial for various natural language processing tasks. Recently, the generative adversarial network (GAN) has attained promising performance in text generation, attributed to its adversarial training process. However, there are several issues in text GANs, including discreteness, training instability, mode collapse, lack of diversity and controllability etc. To address these issues, this paper proposes a novel GAN framework, the feature-aware conditional GAN (FA-GAN), for controllable category text generation. In FA-GAN, the generator has a sequence-to-sequence structure for improving sentence diversity, which consists of three encoders including a special feature-aware encoder and a category-aware encoder, and one relational-memory-core-based decoder with the Gumbel SoftMax activation function. The discriminator has an additional category classification head. To generate sentences with specified categories, the multi-class classification loss is supplemented in the adversarial training. Comprehensive experiments have been conducted, and the results show that FA-GAN consistently outperforms 10 state-of-the-art text generation approaches on 6 text classification datasets. The case study demonstrates that the synthetic sentences generated by FA-GAN can match the required categories and are aware of the features of conditioned sentences, with good readability, fluency, and text authenticity.
更多查看译文
关键词
Category text generation, Conditional generative adversarial net, Adversarial text generation, Generative model, Sequence-to-sequence, Text classification
AI 理解论文
溯源树
样例
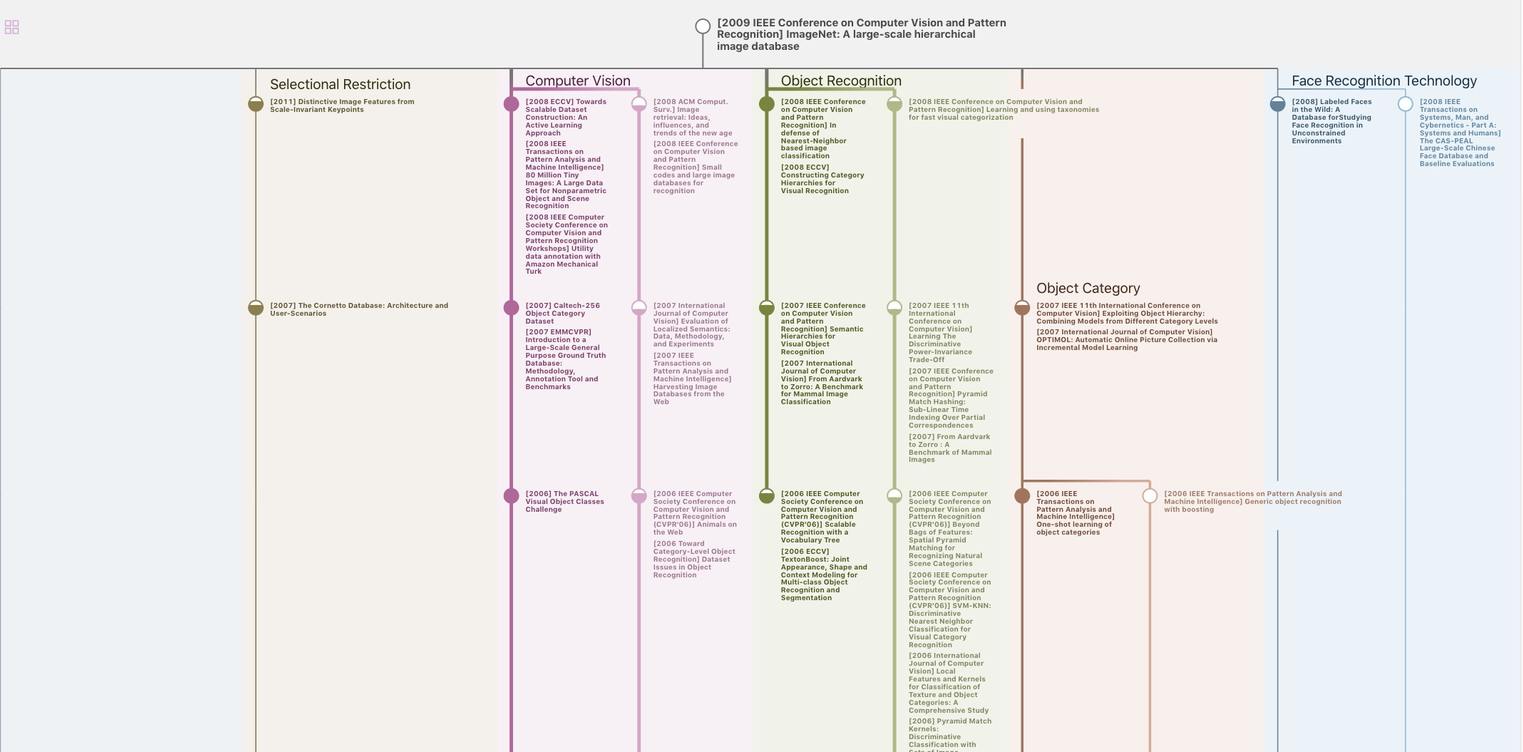
生成溯源树,研究论文发展脉络
Chat Paper
正在生成论文摘要