Intracranial Haemorrhage Diagnosis Using Willow Catkin Optimization With Voting Ensemble Deep Learning on CT Brain Imaging.
IEEE Access(2023)
摘要
Intracranial haemorrhage (ICH) has become a critical healthcare emergency that needs accurate assessment and earlier diagnosis. Due to the high rates of mortality (about 40%), the early classification and detection of diseases through computed tomography (CT) images were needed to guarantee a better prognosis and control the occurrence of neurologic deficiencies. Generally, in the earlier diagnoses test for severe ICH, CT imaging of the brain was implemented in the emergency department. Meanwhile, manual diagnoses are labour-intensive, and automatic ICH recognition and classification techniques utilizing artificial intelligence (AI) models are needed. Therefore, the study presents an Intracranial Haemorrhage Diagnosis using Willow Catkin Optimization with Voting Ensemble (ICHD-WCOVE) Model on CT images. The presented ICHD-WCOVE technique exploits computer vision and ensemble learning techniques for automated ICH classification. The presented ICHD-WCOVE technique involves the design of a multi-head attention-based CNN (MAFNet) model for feature vector generation with optimal hyperparameter tuning using the WCO algorithm. For automated ICH detection and classification, the majority voting ensemble deep learning (MVEDL) technique is used, which comprises recurrent neural network (RNN), Bi-directional long short-term memory (BiLSTM), and extreme learning machine-stacked autoencoder (ELM-SAE). The experimental analysis of the ICHD-WCOVE approach can be tested by a medical dataset and the outcomes signified the betterment of the ICHD-WCOVE technique over other existing approaches.
更多查看译文
关键词
Brain imaging,intracranial haemorrhage,deep learning,computer vision,ensemble learning,willow catkin optimization
AI 理解论文
溯源树
样例
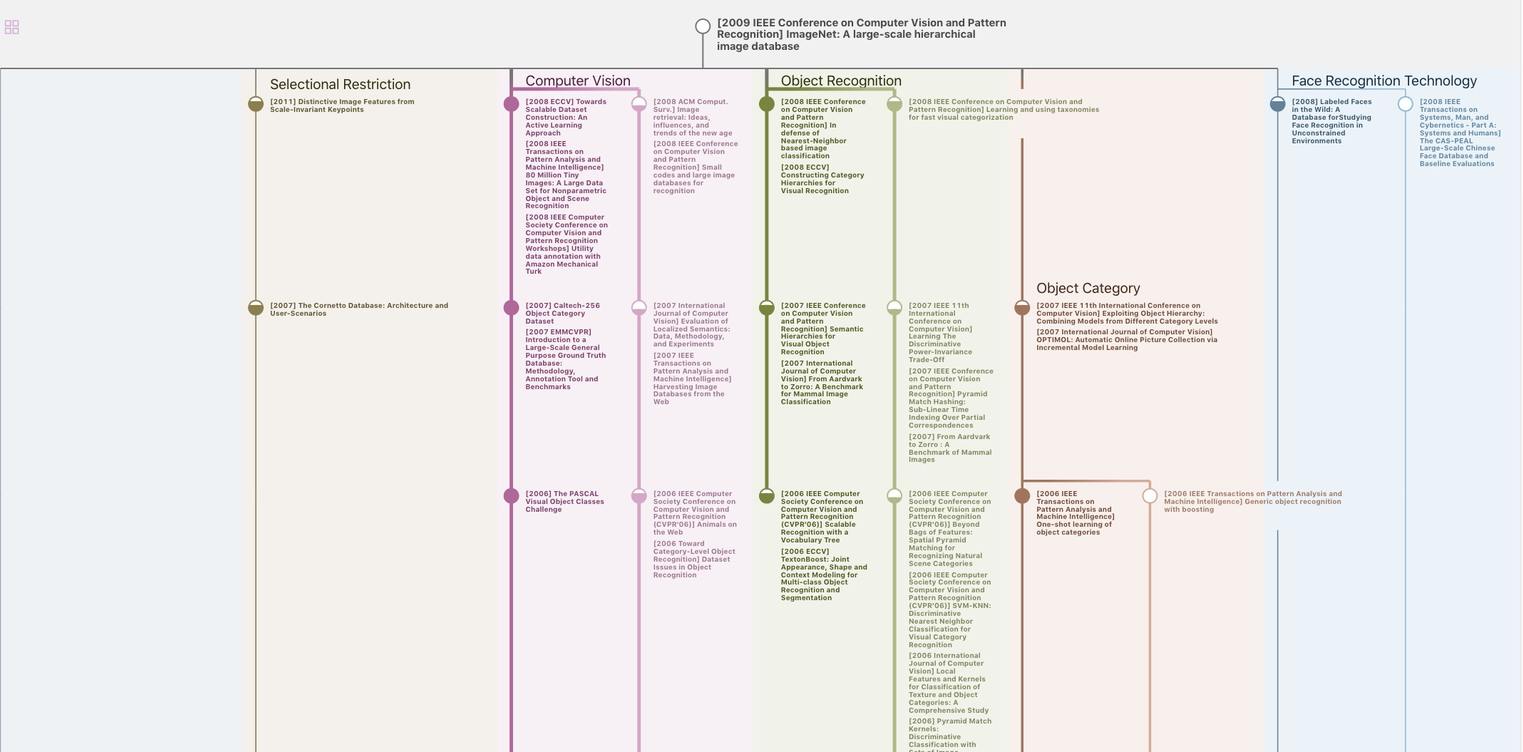
生成溯源树,研究论文发展脉络
Chat Paper
正在生成论文摘要