DCPDN: High-Performance Pedestrian Detection Networks for Difficult Conditions.
IEEE Access(2023)
摘要
Pedestrian detection is the use of computer vision technology to identify and accurately locate pedestrians in image or video data, which has a strong use value. This technology can be used as the research basis for visual tasks such as person re-identification, human pose estimation and behavior analysis, and can also be applied to industrial fields such as intelligent security, automatic driving and human-computer interaction. However, the problems of low image resolution, blurred appearance, large scale difference of pedestrians, occluded pedestrians and complex background still bring great challenges to the detection performance. To solve these problems, this paper proposes a high-performance pedestrian detection network dedicated to difficult conditions: DCPDN. Firstly, we design an optimized super-resolution reconstruction network to preprocess the image to alleviate the performance damage caused by low-resolution and blurred images. Then, to solve the multi-scale problem in pedestrian detection, we propose a weighted cross-scale feature fusion module, which adopts a hierarchical detection strategy to deal with pedestrian objects of different scales while fully fusing feature maps of different levels. Finally, to solve the occlusion problem that has plagued pedestrian detection for a long time, we design an occlusion processing module based on graph convolutional network, which can effectively use the correlation information between different parts of the human body and promote the feature expression of occluded objects. On the CityPersons dataset, the MR-2 of the detector is reduced by 6.9%, 19.2%, 8.9%, 1.9%, 3.6% and 14.2%, respectively, corresponding to different partition subsets of R, HO, A, L, M and S. On the Caltech dataset, corresponding to different divisions of R, HO, A, L and S, the MR-2 of the detector is reduced by 9.9%, 15.8%, 16.3%, 6.8% and 25.8%, respectively. The experimental results show that the performance improvement of the detector is significant on both severe occlusion (HO) and small scale (S) subsets. After testing, the algorithm has strong robustness to occluded pedestrians, and can be easily embedded in other detection frameworks. Our DCPDN is able to compete with the state of the art methods and is especially effective when dealing with the pedestrian detection problem under difficult conditions.
更多查看译文
关键词
Pedestrian detection,super-resolution reconstruction,feature fusion,graph convolutional network
AI 理解论文
溯源树
样例
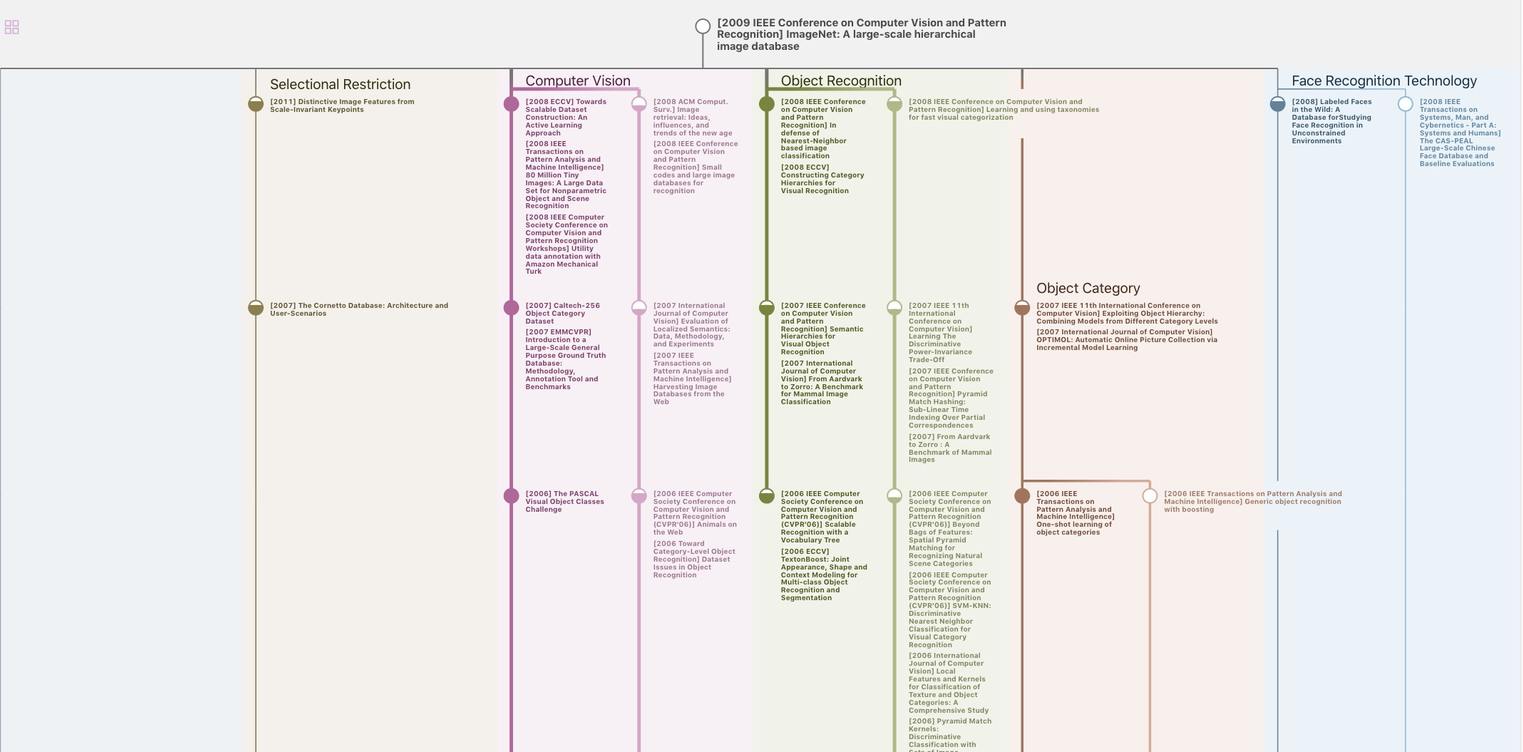
生成溯源树,研究论文发展脉络
Chat Paper
正在生成论文摘要