Optimizing the impact of data augmentation for low-resource grammatical error correction.
J. King Saud Univ. Comput. Inf. Sci.(2023)
摘要
Grammatical Error Correction (GEC) refers to the automatic identification and amendment of grammat-ical, spelling, punctuation, and word-positioning errors in monolingual texts. Neural Machine Translation (NMT) is nowadays one of the most valuable techniques used for GEC but it may suffer from scarcity of training data and domain shift, depending on the addressed language. However, current techniques (e.g., tuning pre-trained language models or developing spell-confusion methods without focusing on lan-guage diversity) tackling the data sparsity problem associated with NMT create mismatched data distri-butions. This paper proposes new aggressive transformation approaches to augment data during training that extend the distribution of authentic data. In particular, it uses augmented data as auxiliary tasks to provide new contexts when the target prefix is not helpful for the next word prediction. This enhances the encoder and steadily increases its contribution by forcing the GEC model to pay more attention to the text representations of the encoder during decoding. The impact of these approaches was investi-gated using the Transformer-based for low-resource GEC task, and Arabic GEC was used as a case study. GEC models trained with our data tend more to source information, are more domain shift robustness, and have less hallucinations with tiny training datasets and domain shift. Experimental results showed that the proposed approaches outperformed the baseline, the most common data augmentation methods, and classical synthetic data approaches. In addition, a combination of the three best approaches Misspelling, Swap, and Reverse achieved the best F1 score in two benchmarks and outperformed previous Arabic GEC approaches.& COPY; 2023 The Authors. Published by Elsevier B.V. on behalf of King Saud University. This is an open access article under the CC BY license (http://creativecommons.org/licenses/by/4.0/).
更多查看译文
关键词
data augmentation,correction,low-resource
AI 理解论文
溯源树
样例
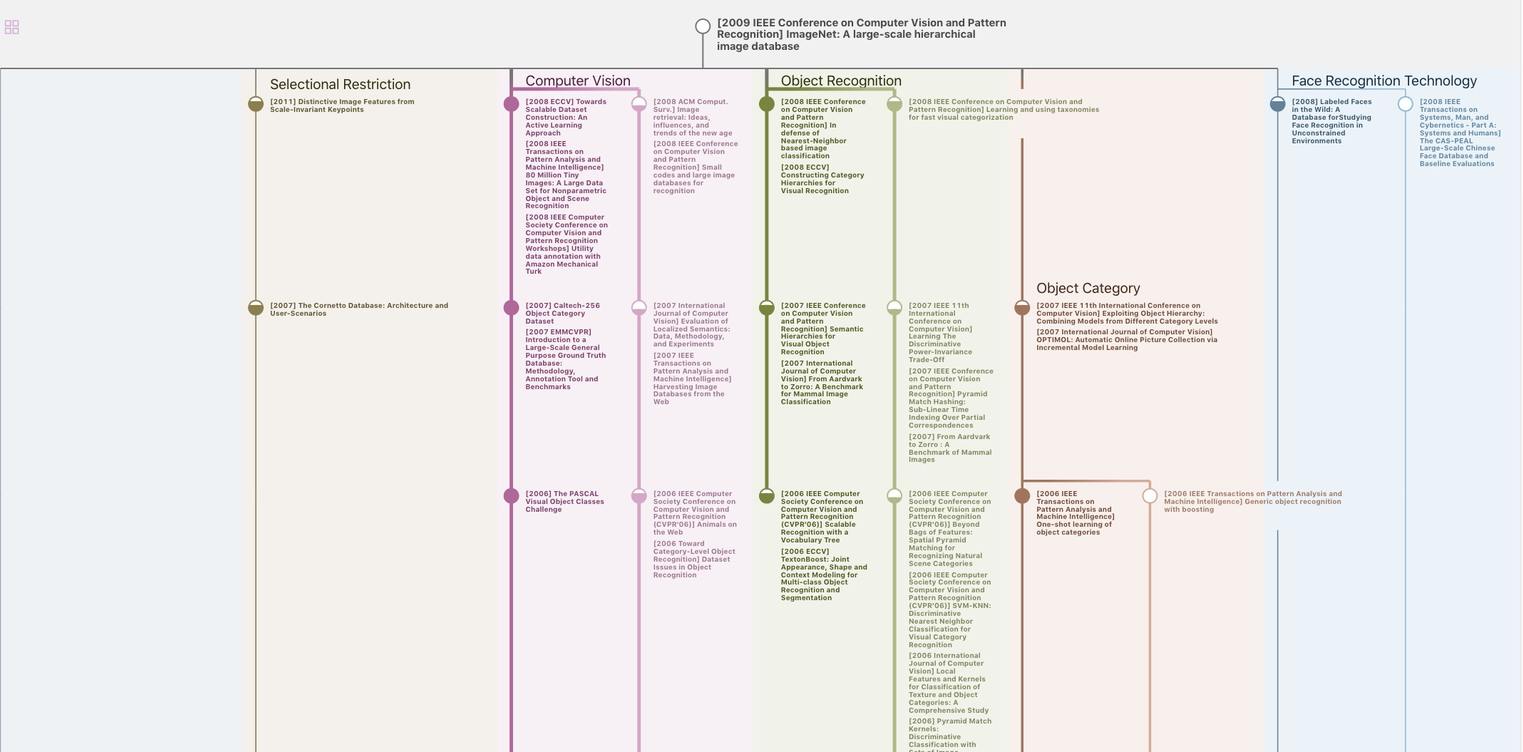
生成溯源树,研究论文发展脉络
Chat Paper
正在生成论文摘要