A Smart Healthcare Framework for Accurate Detection of Schizophrenia Using Multichannel EEG.
IEEE Trans. Instrum. Meas.(2023)
摘要
In recent years, advanced computing techniques have been investigated and employed for the computerized detection of brain disorders like schizophrenia. Schizophrenia is a neurological condition leading to hallucinations and disorganized speech in patients. Emerging deep-learning approaches can augment the computer-based diagnosis of this brain disorder by measuring and analyzing the electroencephalography (EEG) signals. This article proposes a novel concept of the easy-to-wear cap, namely the schizophrenia detection cap (SczCap), integrating hardware and software for acquiring EEG signals from the scalp for accurate schizophrenia detection. In this research, a seven-layer model comprising convolution neural networks (CNNs) and temporal convolution networks (TCNs) is designed and tested using raw EEG data (approach 1) and manually extracted features (approach 2). Three pretrained models, VGG 16, AlexNet, and ResNet 50, along with the proposed model, have also been implemented in this work. A dataset consisting of EEG signals of 14 healthy and 14 schizophrenic patients is used in this work for the implementations. The performance of the proposed CNN-TCN model (approaches 1 and 2) is also presented using nonsubject-wise and subject-wise experiments. It is found that the proposed CNN-TCN model using approach 1 outperformed all other implemented models with 99.57% accuracy, 99.51% sensitivity, 99.64% specificity, and 99.63% precision in nonsubject-wise experiments. The experimental results also exhibit the superiority of this model over other schizophrenia models in the state-of-the-art literature.
更多查看译文
关键词
Convolution neural network (CNN),electroencephalography (EEG) measurement,schizophrenia disorder,smart healthcare,temporal convolution network (TCN),wearable device
AI 理解论文
溯源树
样例
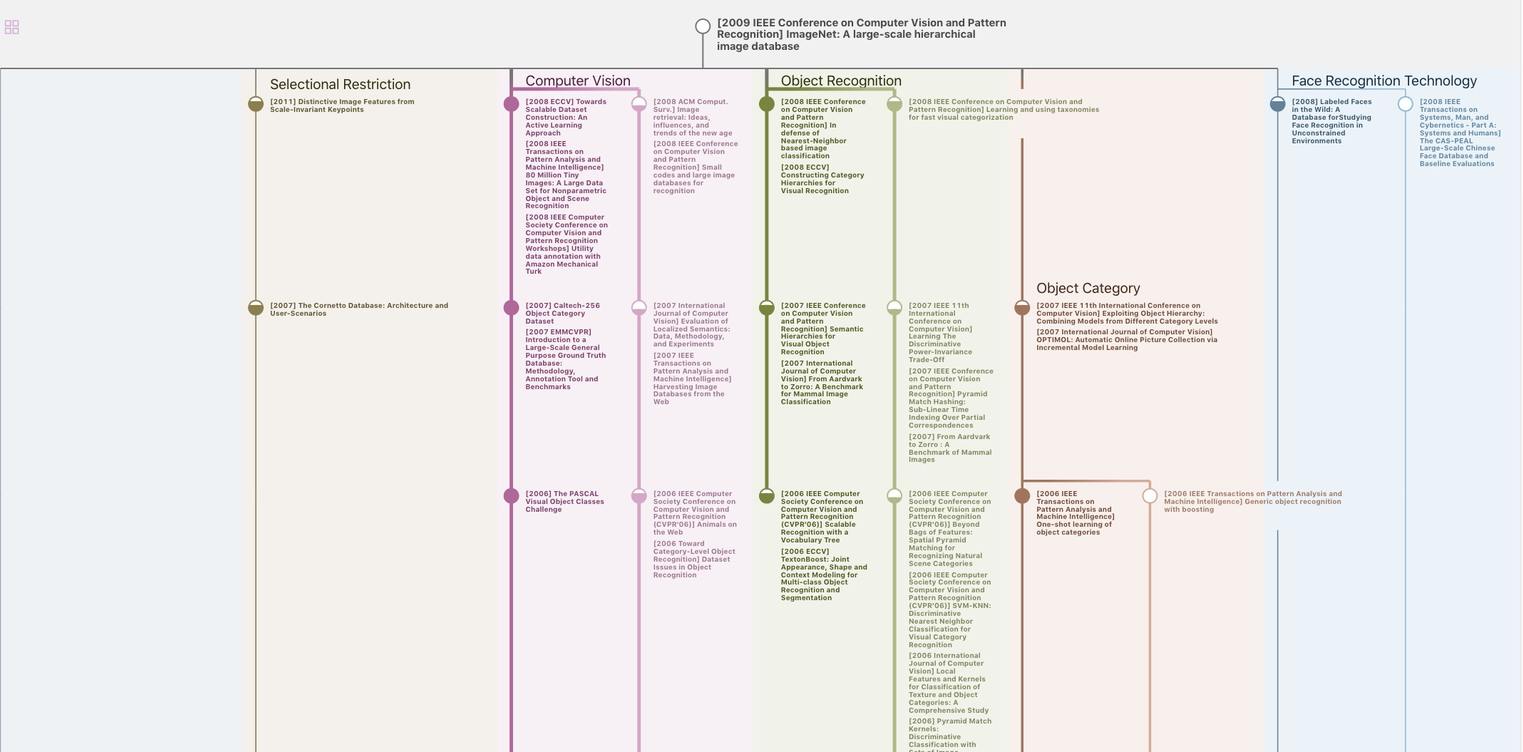
生成溯源树,研究论文发展脉络
Chat Paper
正在生成论文摘要