Multiscale Margin Disparity Adversarial Network Transfer Learning for Fault Diagnosis.
IEEE Trans. Instrum. Meas.(2023)
摘要
Due to the limitation in obtaining sample data in the real world, domain adaption transfer learning (DAT) has been a research focus in fault diagnosis. However, the existing DAT-based fault diagnosis has the problem that the extracted feature in different domains is limited, many existing methods only consider aligning different domains, and the loss function is single. To address these issues, Multiscale Margin Disparity Adversarial Network Transfer Learning (MMDAN) for Fault Diagnosis is proposed in this article. First, the abundant features of different domains are extracted by the proposed multiscale neural network. Specifically, the discrepancy between different domains is measured by Margin Disparity and adversarial loss. Meanwhile, the classifier achieves the fault diagnosis. Finally, a joint loss function is proposed to update the neural network parameters. Two different case studies are carried out to verify the effectiveness of MMDAN. The experimental results show that MMDAN can achieve the highest diagnosis accuracy than other methods even in multitask transfer learning (TL).
更多查看译文
关键词
fault diagnosis,transfer,learning,multi-scale
AI 理解论文
溯源树
样例
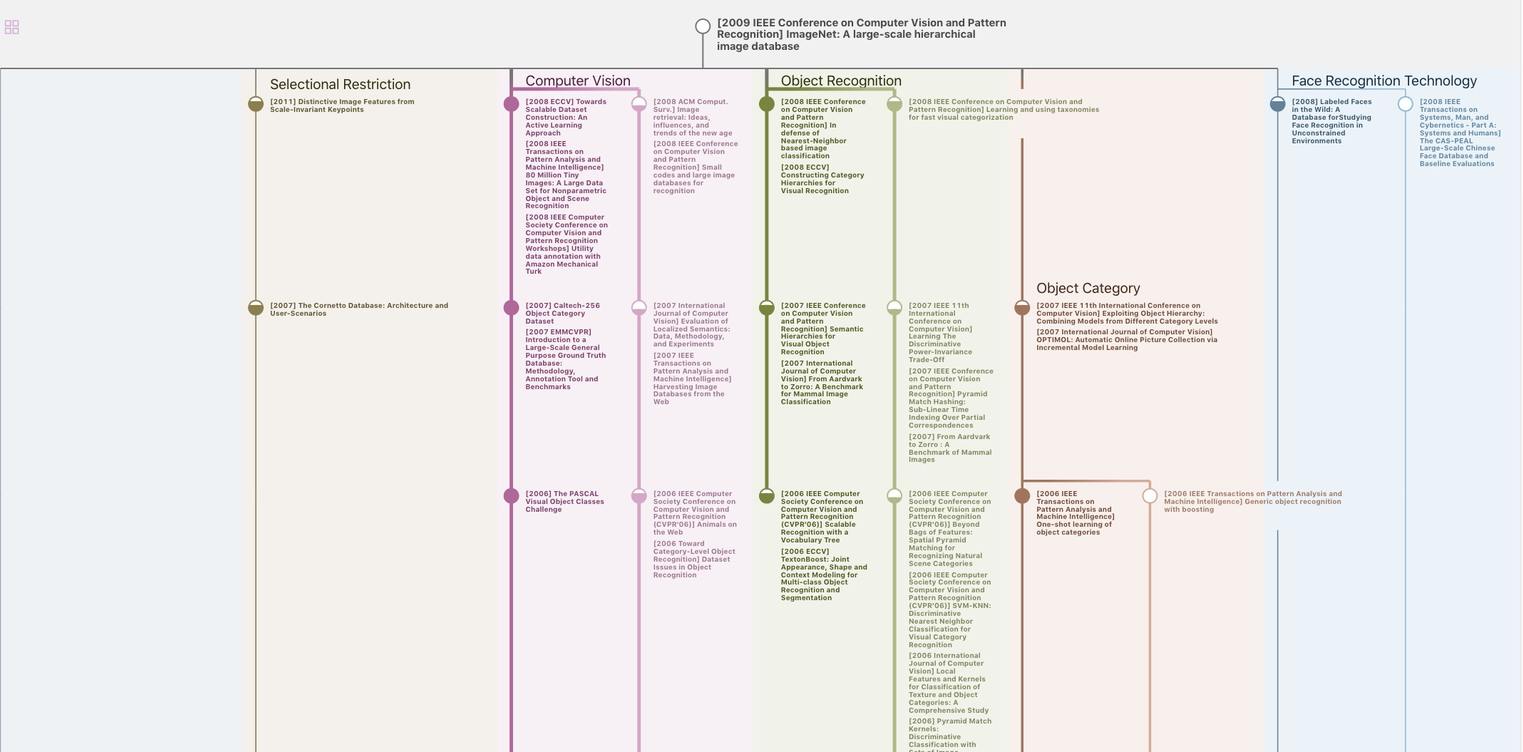
生成溯源树,研究论文发展脉络
Chat Paper
正在生成论文摘要