Bayesian Target Detection Algorithms for Solid Subpixel Targets in Hyperspectral Images.
IEEE Trans. Geosci. Remote. Sens.(2023)
摘要
We investigate the use of Bayesian methods for hyperspectral subpixel target detection, where the uncertainty associated with the target fill factor is "probabilized" by a suitable prior. Specifically, we present a general framework for Bayesian target detection by employing different models for the background distribution, comparing different choices for the Bayesian prior, and investigating different numerical schemes for evaluating the Bayesian integral. The Bayesian methods are furthermore compared to their generalized likelihood ratio test (GLRT)-based counterparts. Experiments performed over real hyperspectral imagery, with both real and implanted subpixel targets, show that incorporating prior knowledge by means of nonuniform priors emphasizing smaller target fill factors outperforms usage of the "noninformative" uniform prior and enhances Bayes performance beyond the GLRT, a result observed for both parametric and nonparametric background models. We find that even "rough" priors can successfully leverage the context-based information by emphasizing target sizes that are of most interest. We further observe that the Gauss-Legendre numerical integration scheme provides efficient integral approximation while maintaining the desirable admissibility property of Bayesian methods.
更多查看译文
关键词
Bayesian statistics,generalized likelihood ratio test (GLRT),hyperspectral,kernel density estimation,likelihood ratio,target detection
AI 理解论文
溯源树
样例
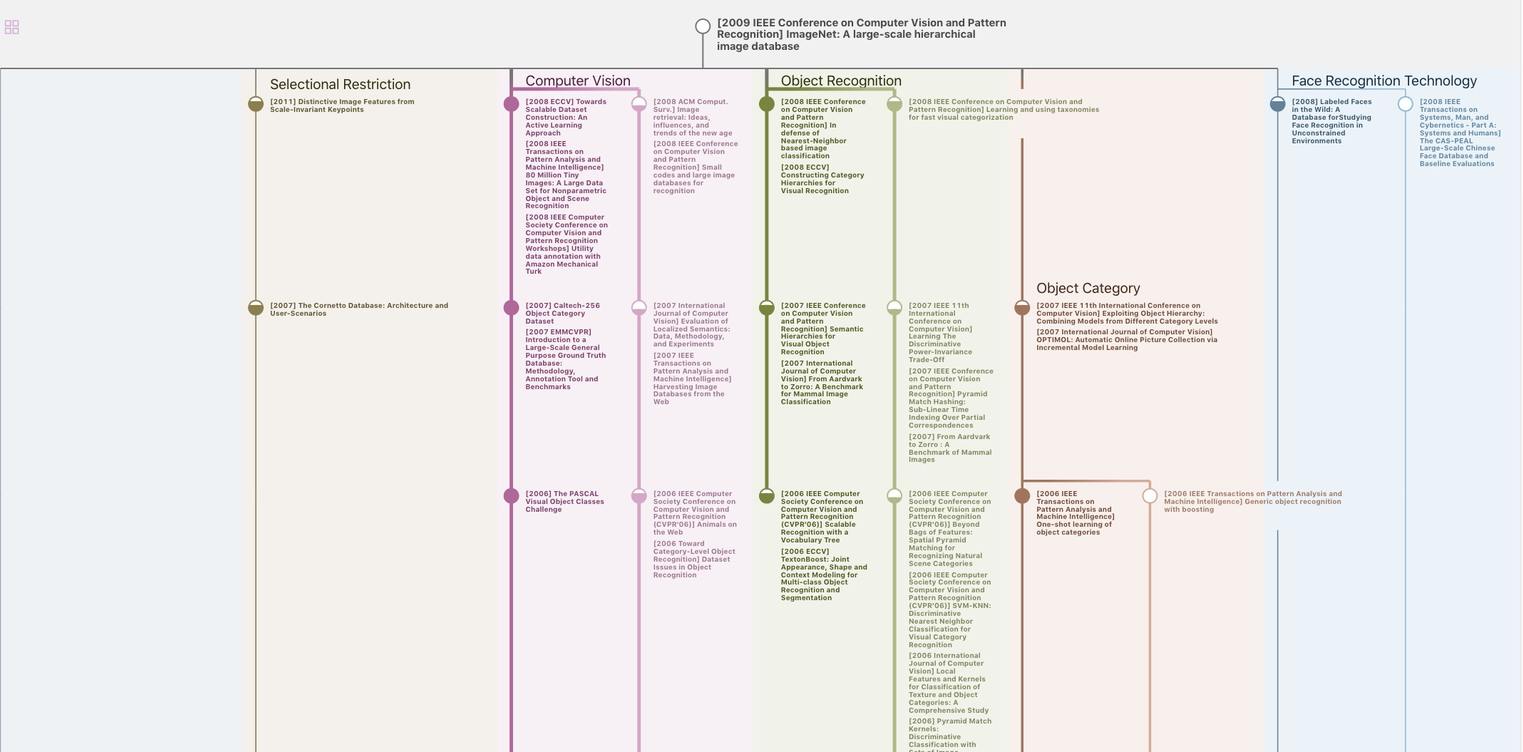
生成溯源树,研究论文发展脉络
Chat Paper
正在生成论文摘要