Joint Learning of Semantic Segmentation and Height Estimation for Remote Sensing Image Leveraging Contrastive Learning.
IEEE Trans. Geosci. Remote. Sens.(2023)
摘要
Semantic segmentation (SS) and height estimation (HE) are two critical tasks in remote sensing scene understanding that are highly correlated with each other. To address both the tasks simultaneously, it is natural to consider designing a unified deep learning model that aims to improve performance by jointly learning complementary information among the associated tasks. In this article, we learn the two tasks jointly under a deep multitask learning (MTL) framework and propose two novel objective functions, called cross-task contrastive (CTC) loss and cross-pixel contrastive (CPC) loss, respectively, to enhance MTL performance through contrastive learning. Specifically, the CTC loss is designed to maximize the mutual information of different task features and enforce the model to learn the consistency between SS and height estimation. In addition, our method goes beyond previous approaches that only apply contrastive learning at the instance level. Instead, we design a pixelwise contrastive loss function that pulls together pixel embeddings belonging to the same semantic class, while pushing apart pixel embeddings from different semantic classes. Furthermore, we find that this semantic-guided contrastive loss simultaneously improves the performance of the HE task. Our proposed approach is simple and effective and does not introduce any additional overhead to the model during the testing phase. We extensively evaluate our method on the Vaihingen and Potsdam datasets, and the experimental results demonstrate that our approach significantly outperforms the state-of-the-art methods in both HE and SS.
更多查看译文
关键词
Contrastive learning, height estimation, multi-task learning (MTL), remote sensing, semantic segmentation (SS)
AI 理解论文
溯源树
样例
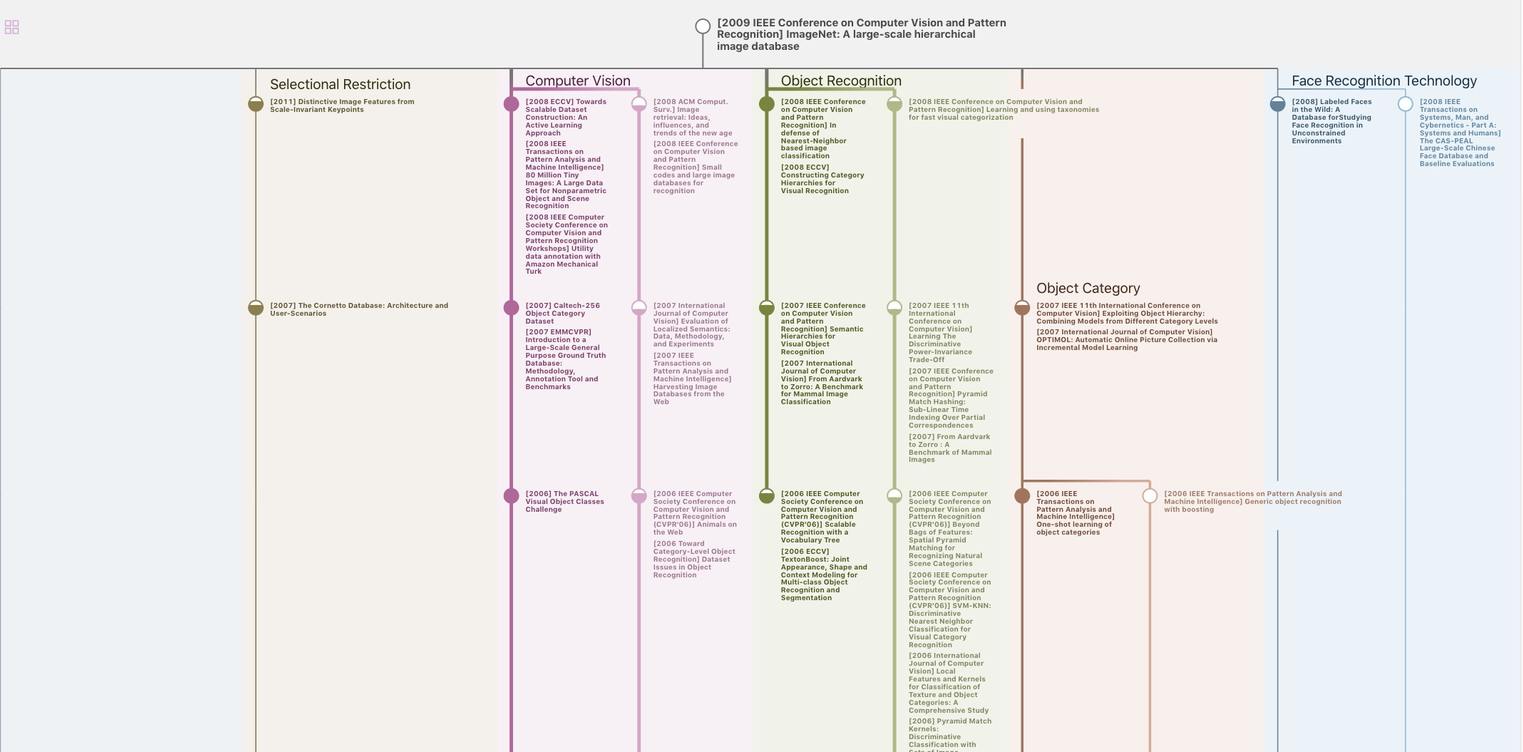
生成溯源树,研究论文发展脉络
Chat Paper
正在生成论文摘要