CLDM: convolutional layer dropout module
Mach. Vis. Appl.(2023)
摘要
Deep convolutional neural networks (CNNs) are prone to overfitting due to their overparameterization. Structural dropout methods such as weighted channel dropout alleviate this problem by dropping continuous regions based on the importance degrees computed from the average activation values of each channel in the feature map. However, there is insufficient evidence supporting the mean value as a representative evaluation method of importance degree. Additionally, the importance degree of a channel may also be related to kernel information. To better represent the importance degree of channels, this work proposes using the variance instead of the mean as the importance evaluation method of regions in structural dropout methods and proposes a convolutional layer dropout module (CLDM), which utilizes the variance values of both the kernel and feature map to determine the regions that can be dropped. CLDM is a parameter-free plug-and-play module used for regularizing various deep CNNs without any additional computational cost during the test phase. Extensive experimental results on various datasets demonstrate that the proposed CLDM outperforms other state-of-the-art structural dropout methods and proves the effectiveness of the variance evaluation method and the benefit of introducing kernel information in the dropout process, respectively.
更多查看译文
关键词
CNN,Regularization method,Structural dropout,Variance
AI 理解论文
溯源树
样例
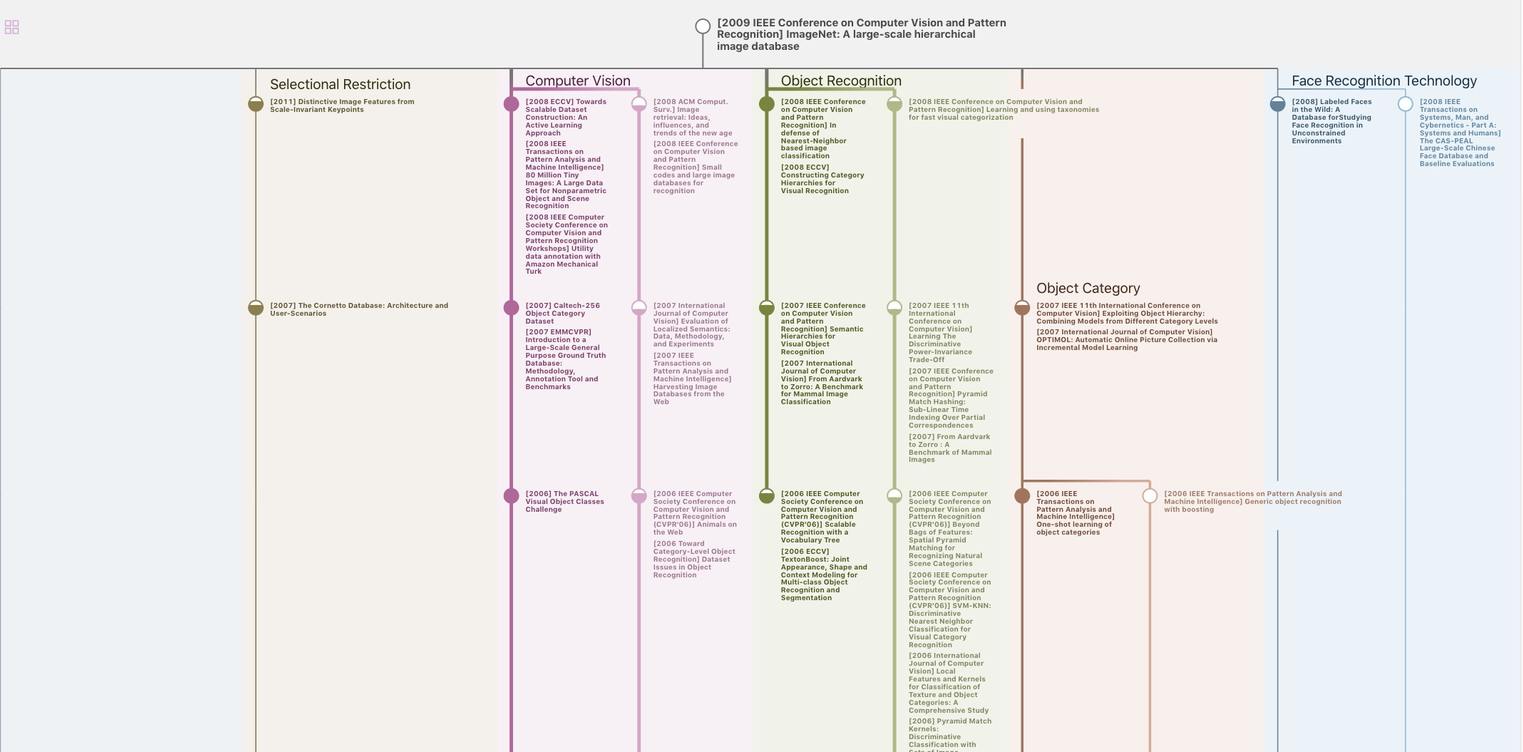
生成溯源树,研究论文发展脉络
Chat Paper
正在生成论文摘要