The effect of intrinsic dimension on the Bayes-error of projected quadratic discriminant classification
Stat. Comput.(2023)
摘要
High-dimensionality is a common hurdle in machine learning and pattern classification; mitigating its effects has attracted extensive research efforts. It has been found in a recent NeurIPS paper that, when the data possesses a low effective dimension, the predictive performance of a discriminative quadratic classifier with nuclear norm regularisation enjoys a reduced (logarithmic) dependence on the ambient dimension and depends on the effective dimension instead, while other regularisers are insensitive to the effective dimension. In this paper, we show that dependence on the effective dimension is also exhibited by the Bayes error of the generative Quadratic Discriminant Analysis (QDA) classifier, without any explicit regularisation, under three linear dimensionality reduction schemes. Specifically, we derive upper bounds on the Bayes error of QDA, which adapt to the effective dimension, and entirely bypass any dependence on the ambient dimension. Our findings complement previous results on compressive QDA that were obtained under compressive sensing type assumptions on the covariance structure. In contrast, our bounds make no a-priori assumptions on the covariance structure, in turn they tighten in the presence of benign traits of the covariance. We corroborate our findings with numerical experiments.
更多查看译文
关键词
classification,intrinsic dimension,bayes-error
AI 理解论文
溯源树
样例
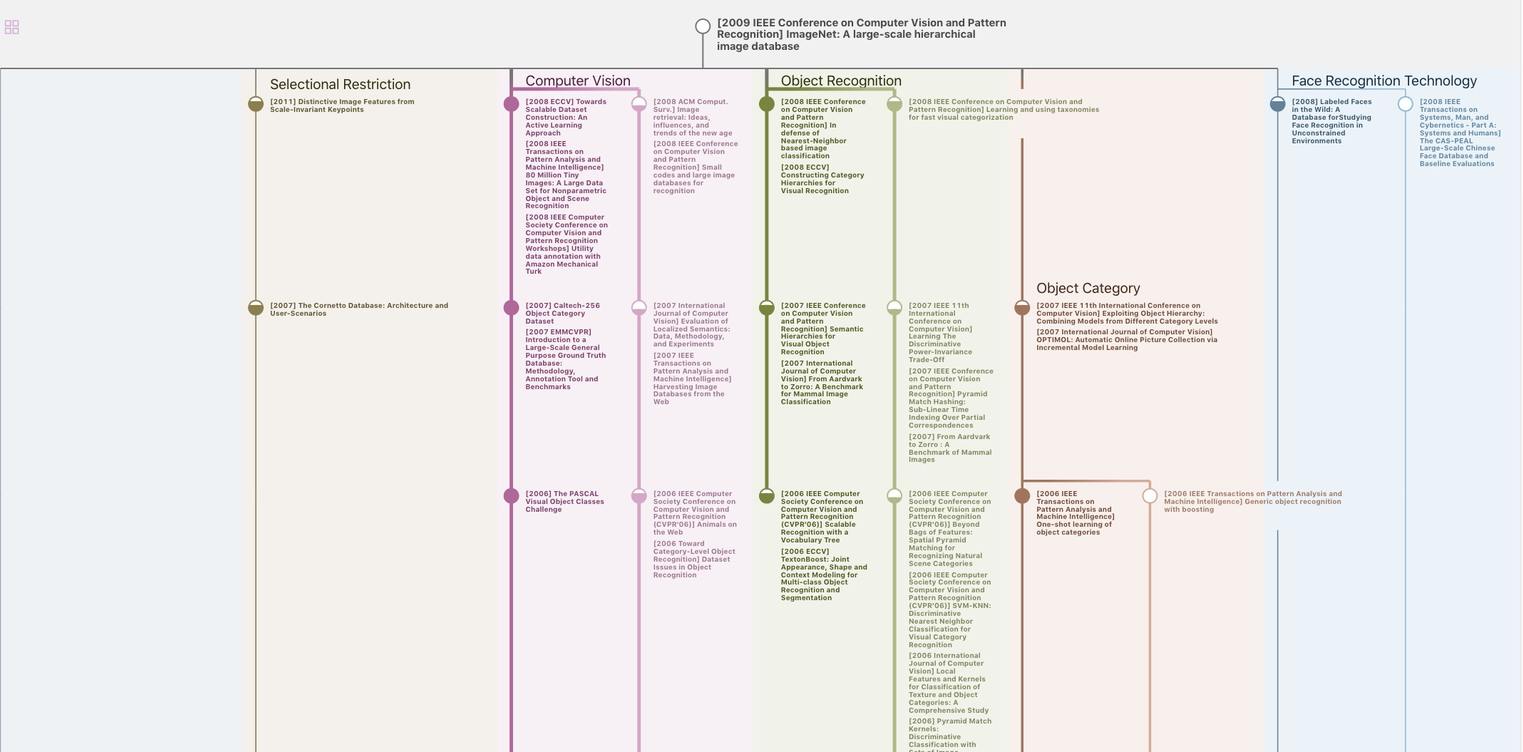
生成溯源树,研究论文发展脉络
Chat Paper
正在生成论文摘要