The attentive reconstruction of objects facilitates robust object recognition
biorxiv(2024)
摘要
Humans are extremely robust in our ability to perceive and recognize objects—we see faces in tea stains and can recognize friends on dark streets. Yet, neurocomputational models of primate object recognition have focused on the initial feed-forward pass of processing through the ventral stream and less on the top-down feedback that likely underlies robust object perception and recognition. Aligned with the generative approach, we propose that the visual system actively facilitates recognition by reconstructing the object hypothesized to be in the image. Top-down attention then uses this reconstruction as a template to bias feedforward processing to align with the most plausible object hypothesis. Building on auto-encoder neural networks, our model makes detailed hypotheses about the appearance and location of the candidate objects in the image by reconstructing a complete object representation from potentially incomplete visual input due to noise and occlusion. The model then leverages the best object reconstruction, measured by reconstruction error, to direct the bottom-up processing of selectively routing low-level features, a top-down biasing that captures a core function of attention. We evaluated our model using the MNIST-C (handwritten digits under corruptions) and ImageNet-C (real-world objects under corruptions) datasets. Not only did our model achieve superior performance on these challenging tasks designed to approximate real-world noise and occlusion viewing conditions, but also better accounted for human behavioral reaction times and error patterns than a standard feedforward Convolutional Neural Network. Our model suggests that a complete understanding of object perception and recognition requires integrating top-down and attention feedback, which we propose is an object reconstruction.
Author Summary Humans can dream and imagine things, and this means that the human brain can generate perceptions of things that are not there. We propose that humans evolved this generation capability, not solely to have more vivid dreams, but to help us better understand the world, especially when what we see is unclear or missing some details (due to occlusion, changing perspective, etc.). Through a combination of computational modeling and behavioral experiments, we demonstrate how the process of generating objects—actively reconstructing the most plausible object representation from noisy visual input—guides attention towards specific features or locations within an image (known as functions of top-down attention), thereby enhancing the system’s robustness to various types of noise and corruption. We found that this generative attention mechanism could explain, not only the time that it took people to recognize challenging objects, but also the types of recognition errors made by people (seeing an object as one thing when it was really another). These findings contribute to a deeper understanding of the computational mechanisms of attention in the brain and their potential connection to the generative processes that facilitate robust object recognition.
### Competing Interest Statement
The authors have declared no competing interest.
更多查看译文
AI 理解论文
溯源树
样例
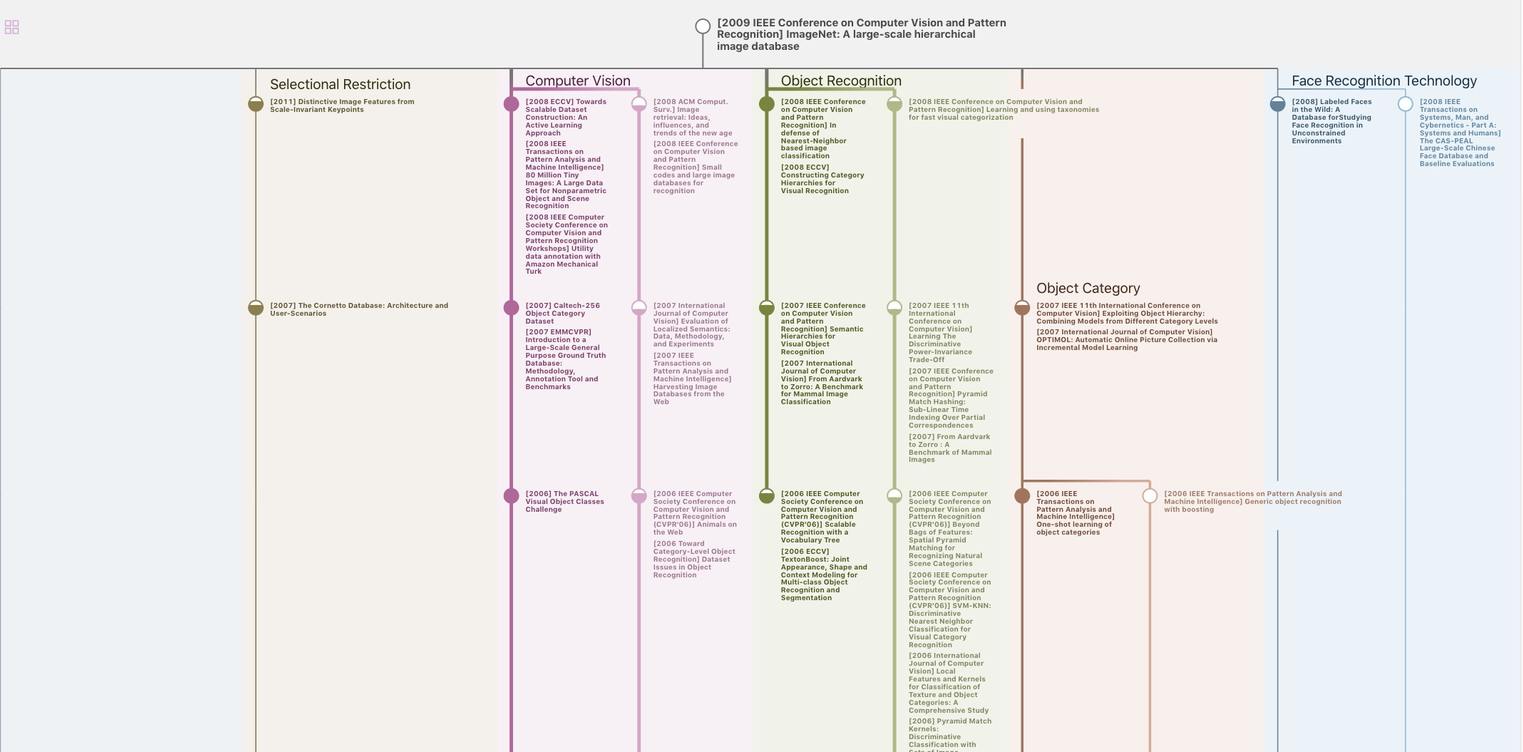
生成溯源树,研究论文发展脉络
Chat Paper
正在生成论文摘要