A High Performance and Robust FPGA Implementation of a Driver State Monitoring Application
Sensors (Basel, Switzerland)(2023)
摘要
A high-performance Driver State Monitoring (DSM) application for the detection of driver drowsiness is presented in this paper. The popular Ensemble of Regression Trees (ERTs) machine learning method has been employed for the alignment of 68 facial landmarks. Open-source implementation of ERTs for facial shape alignment has been ported to different platforms and adapted for the acceleration of the frame processing speed using reconfigurable hardware. Reducing the frame processing latency saves time that can be used to apply frame-to-frame facial shape coherency rules. False face detection and false shape estimations can be ignored for higher robustness and accuracy in the operation of the DSM application without sacrificing the frame processing rate that can reach 65 frames per second. The sensitivity and precision in yawning recognition can reach 93% and 97%, respectively. The implementation of the employed DSM algorithm in reconfigurable hardware is challenging since the kernel arguments require large data transfers and the degree of data reuse in the computational kernel is low. Hence, unconventional hardware acceleration techniques have been employed that can also be useful for the acceleration of several other machine learning applications that require large data transfers to their kernels with low reusability.
更多查看译文
关键词
shape alignment, driver drowsiness, face detection validity, machine learning, hardware acceleration, Vitis HLS
AI 理解论文
溯源树
样例
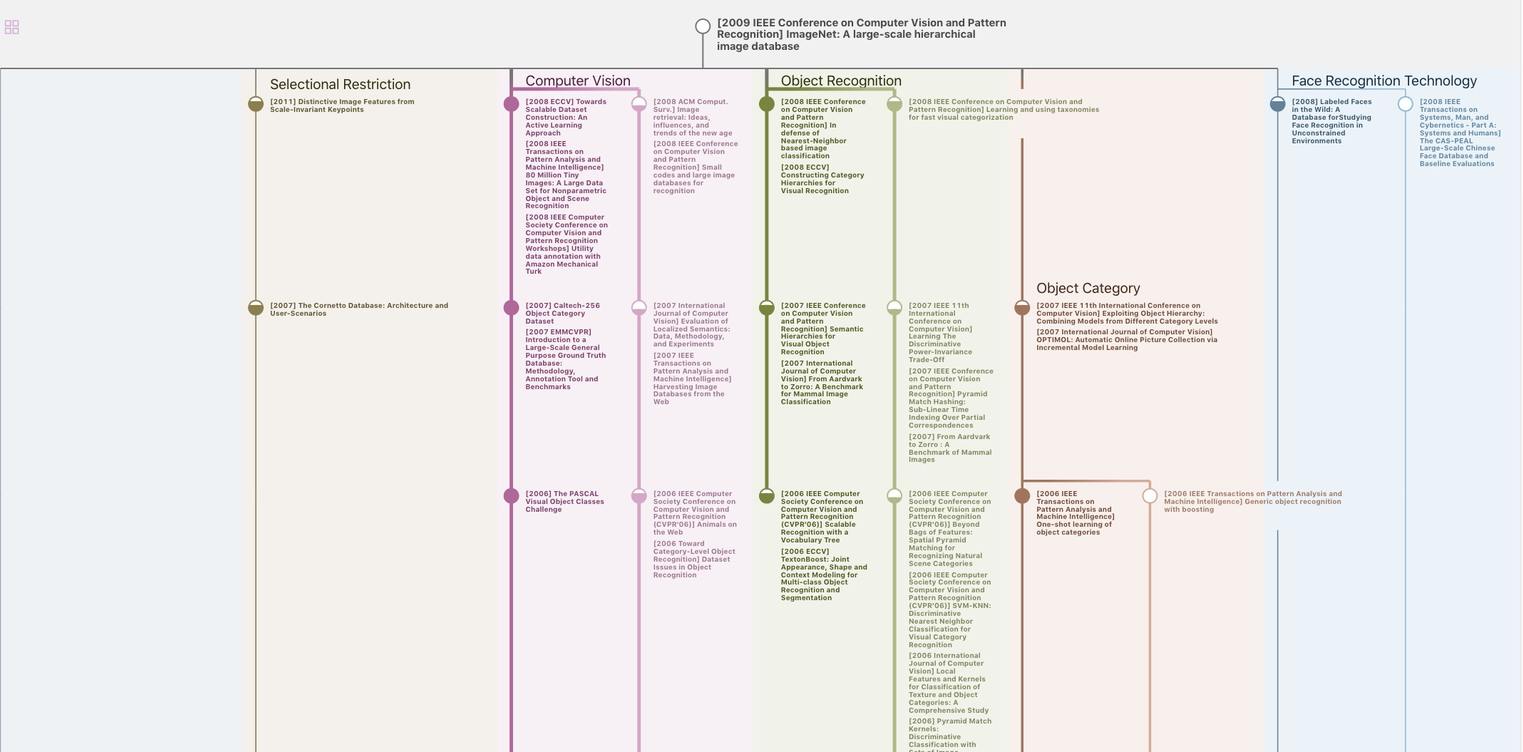
生成溯源树,研究论文发展脉络
Chat Paper
正在生成论文摘要